SGNet: A Transformer-Based Semantic-Guided Network for Building Change Detection
IEEE Journal of Selected Topics in Applied Earth Observations and Remote Sensing(2024)
摘要
Building change detection (BCD) is a widely used method for monitoring human activities. Despite advancements in deep learning (DL) in computer vision, recent DL-based BCD methods still face challenges in extracting discriminative features due to irrelevant noisy changes and the lack of consideration for specific semantic information related to changes. To address this limitation, we propose the Semantic-Guided Network (SGNet), a multi-scale pure Transformer-based building change detection method that delves into more discriminative class-specific feature representations for changed buildings. Specifically, we first breakdown the change detection process into multiple stages: changed semantics extraction, enhancement, fusion, and decoding. Then, we analyze the nature of building change events in remote sensing images (RSIs) and categorize them into three main groups. To detect positive changes and reduce noise, we developed the Various Changes Extraction Module (VCEM). Additionally, we introduce the semantic-guided Changed-Semantics Augmentation Module (CSAM) to enhance the semantic information of target changes while suppressing irrelevant regions or objects. We also propose the Various Changes Fusion Module (VCFM) to model semantic latency in bi-temporal RSIs. Extensive experiments on two building change datasets, i.e., LEVIR-CD and WHU-CD demonstrate that our SGNet(MiT-b0) outperforms the state-of-the-art baselines and achieves F1 score of 90.99% and 92.47%, respectively, with improvements of 1.9% and 7.89%, respectively, highlighting the exceptional sample-efficient learning capability. The source code will be released at
https://github.com/Anderien/official-SGNet
更多查看译文
关键词
Building change detection,Semantic guided,Transformer,Remote sensing
AI 理解论文
溯源树
样例
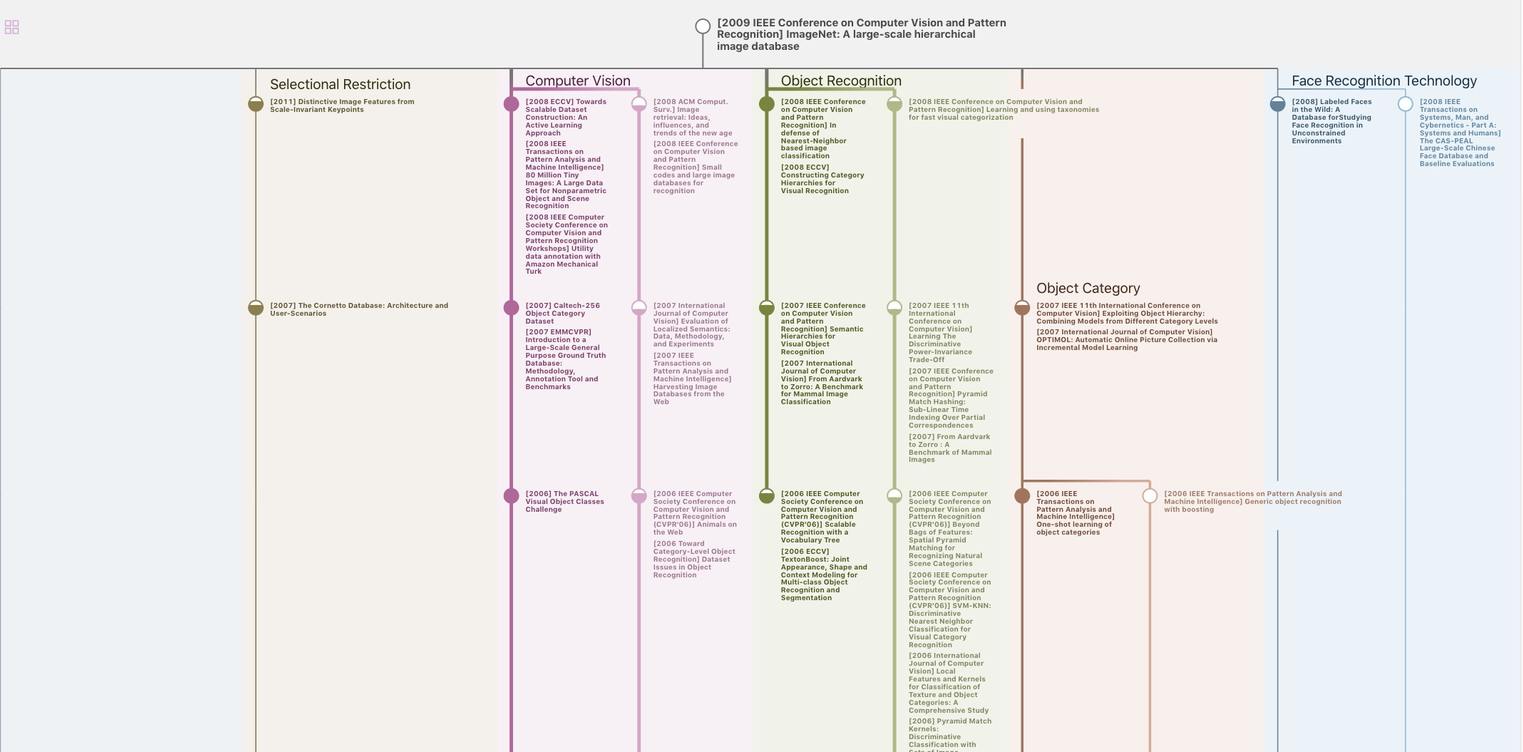
生成溯源树,研究论文发展脉络
Chat Paper
正在生成论文摘要