Efficient Application of Deep Neural Networks for Identifying Small and Multiple Weed Patches Using Drone Images
IEEE access(2024)
摘要
Deep learning (DL) based camouflage target localization, classification and detection using UAV data is currently an evolving and promising hotspot in the field of digital image processing and computer vision. Weed, an undesirable plant that hinders crop growth, acts as a camouflage target w.r.t sugarcane crop (Saccharum Officinarum). Weed exhibits green-on-green color-based merging capability (background matching) and similar spectral behavior with the sugarcane crop, resulting in a visually complex camouflage habitat, making it difficult to distinguish and detect weed from crop. The concealed nature of weed in the sugarcane field, qualifies it as a camouflage target. In this scenario, weed is distributed in the form of multiple small patches across the UAV imagery, making the detection even more complicated, leading to a requirement of a pixel-based classification and subsequent target detection technique. The research problem is to successfully detect weed (camouflage target) at the smallest resolvable pixel size (2-4 cm/px), keeping in mind the similar behavior (merging color) and its difficulty to differentiate it from the sugarcane crop (background). To achieve this challenge, color and texture are exploited as important feature representations. They are extracted via UAV image(s), which can aid in small and multiple weed patch detection, by implementing a rich feature-based Deep Neural Network (DNN). In this revolutionary era of AI and modern drones, DNNs provide feature based elastic transformations, expedited processing, improved accuracy, and optimized mapping and detection. These representation-based networks traverse deeper into color and texture feature representations as a unified component, pixel by pixel, eventually detecting the camouflage targets by their self-learning capabilities in comparison to traditional classification approaches. DNNs exhibit good capabilities to solve camouflage target detection problems, therefore we have proposed a methodology based on deep learning feature-based modelling which generates classified and localized image maps that highlight multiple and small weed patches at the minimal pixel level possible from a merged green-on green background. We have explored and proposed a methodology that self learns exhaustive features from the UAV imagery via feature transformations which in turn reduces the feature bias and exhibits enhanced prospects w.r.t sensitivity of imagery background. This eventually results in improved localization and classification of camouflage targets with respect to traditional target detection and localization modelling techniques in natural background. It is observed that the proposed methodology is capable of detecting small patches of weed (similar color behavior) from its background with an accuracy of 90.5%.
更多查看译文
关键词
UAV,deep neural networks,classification,target detection,computer vision
AI 理解论文
溯源树
样例
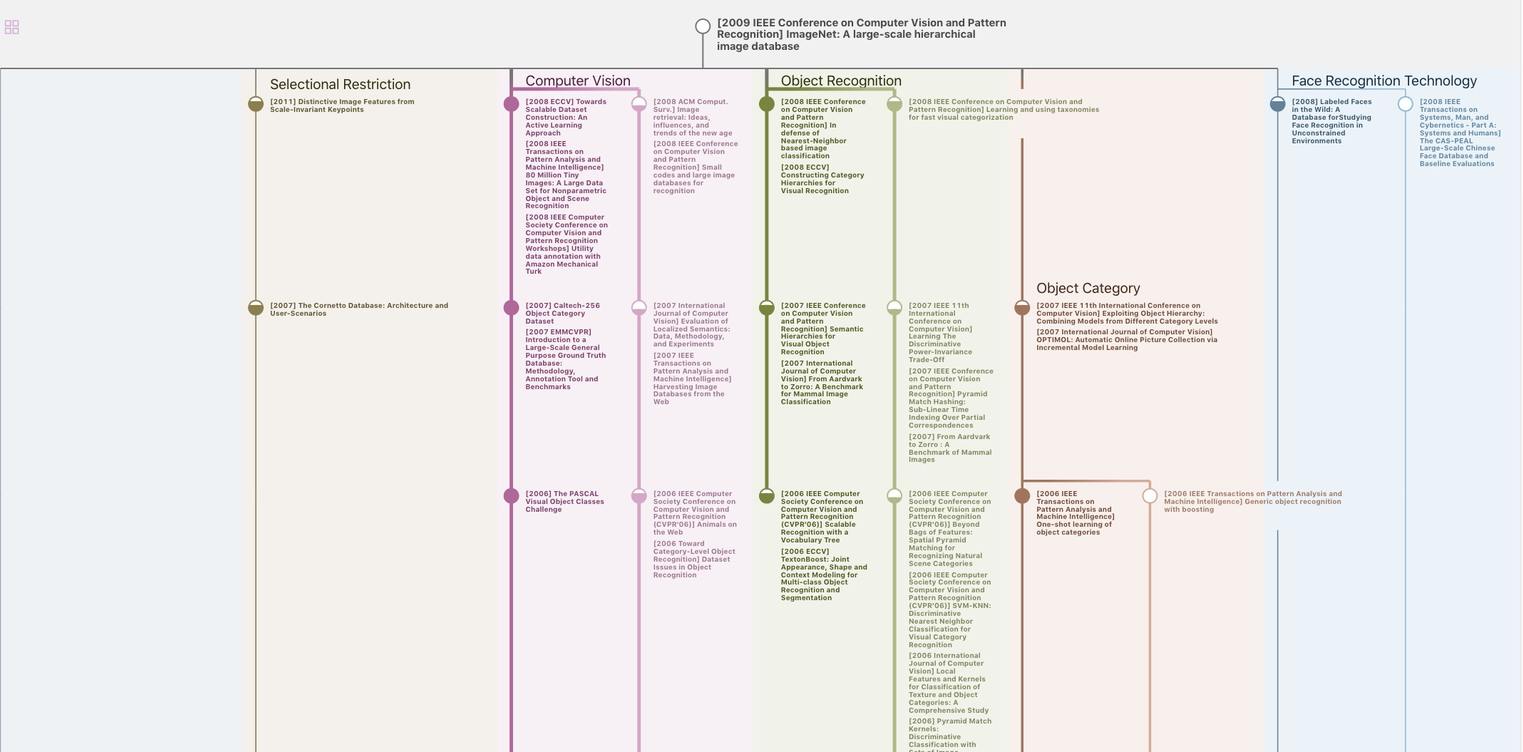
生成溯源树,研究论文发展脉络
Chat Paper
正在生成论文摘要