Heterogeneous Graph Contrastive Learning with Augmentation Graph
IEEE Transactions on Artificial Intelligence(2024)
摘要
Heterogeneous graph neural networks (HGNNs) have demonstrated promising capabilities in addressing various problems defined on heterogeneous graphs containing multiple types of nodes or edges. However, traditional HGNN models depend on label information and capture the local structural information of the original graph. In this paper, we propose a novel Heterogeneous Graph Contrastive Learning method with Augmentation Graph (AHGCL). Specifically, we construct an augmentation graph by calculating the feature similarity of nodes to capture latent structural information. For the original graph and the augmentation graph, we employ a shared Graph Neural Network (GNN) encoder to extract the semantic features of nodes with different meta-paths. The feature information is aggregated through a semantic-level attention mechanism to generate final node embeddings, which capture latent high-order semantic structural information. Considering the problems of label information for the real-world datasets, we adopt contrastive learning to train the GNN encoder for maximizing the common information between similar nodes from the original graph and the augmentation graph views. We conduct node classification experiments on four real-world datasets, AMiner, Freebase, DBLP, and ACM, to evaluate the performance of AHGCL. The results show that the proposed AHGCL demonstrates excellent stability and capability compared to existing graph representation learning methods.
更多查看译文
关键词
Heterogeneous graph neural networks,Self-supervised learning,Graph contrastive learning,Node classification
AI 理解论文
溯源树
样例
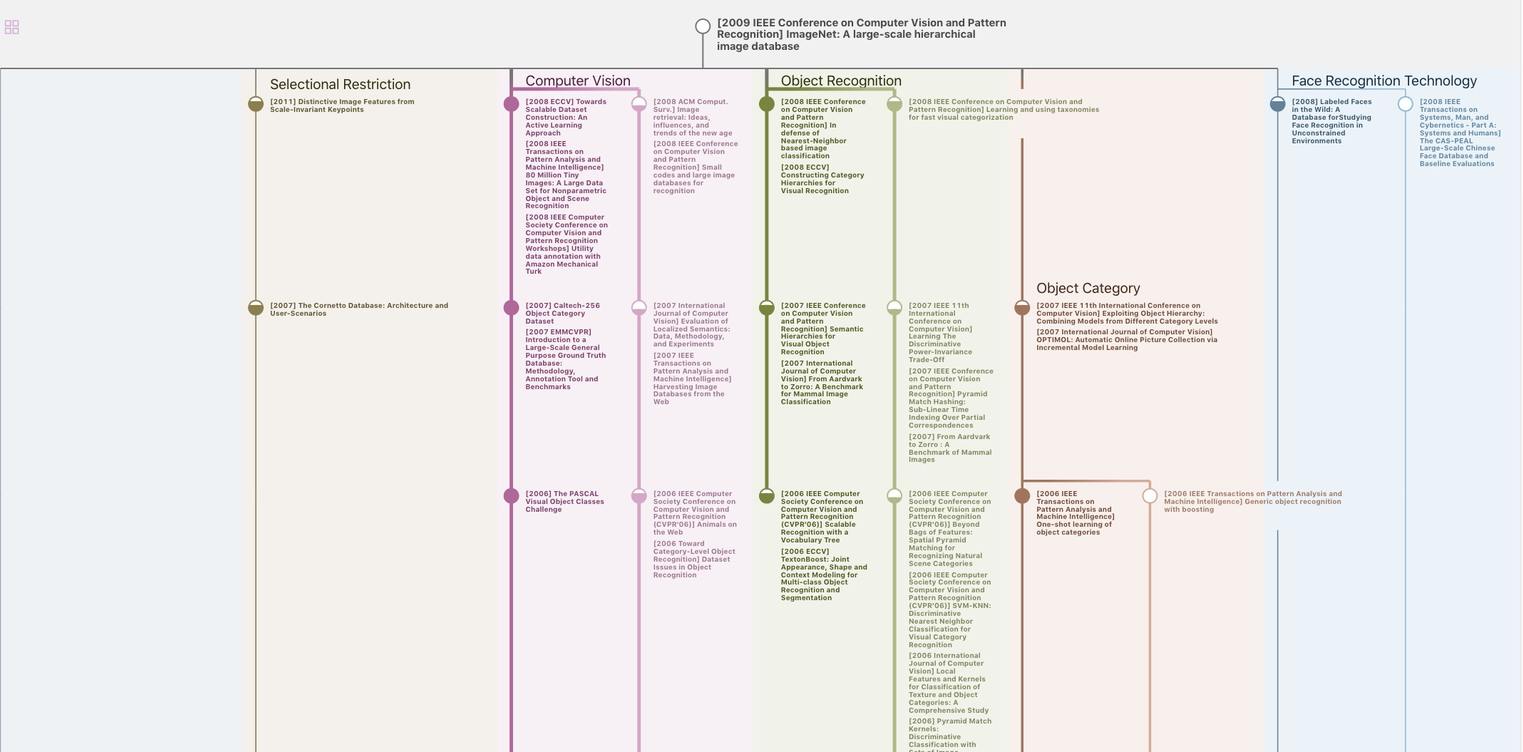
生成溯源树,研究论文发展脉络
Chat Paper
正在生成论文摘要