Authorship Style Transfer with Inverse Transfer Data Augmentation
AI Open(2024)
摘要
Authorship style transfer aims to modify the style of neutral text to match the unique speaking or writing style of a particular individual. While Large Language Models (LLMs) present promising solutions, their effectiveness is limited by the small number of in-context learning demonstrations, particularly for authorship styles not frequently seen during pre-training. In response, this paper proposes an inverse transfer data augmentation (ITDA) method, leveraging LLMs to create (neutral text, stylized text) pairs. This method involves removing the existing styles from stylized texts, a process made more feasible due to the prevalence of neutral texts in pre-training. We use this augmented dataset to train a compact model that is efficient for deployment and adept at replicating the targeted style. Our experimental results, conducted across four datasets with distinct authorship styles, establish the effectiveness of ITDA over traditional style transfer methods and forward transfer using GPT-3.5. For further research and application, our dataset and code are openly accessible at https://github.com/AnonymousRole/ITDA.
更多查看译文
关键词
Natural language generation,Stylistic analysis,Style transfer
AI 理解论文
溯源树
样例
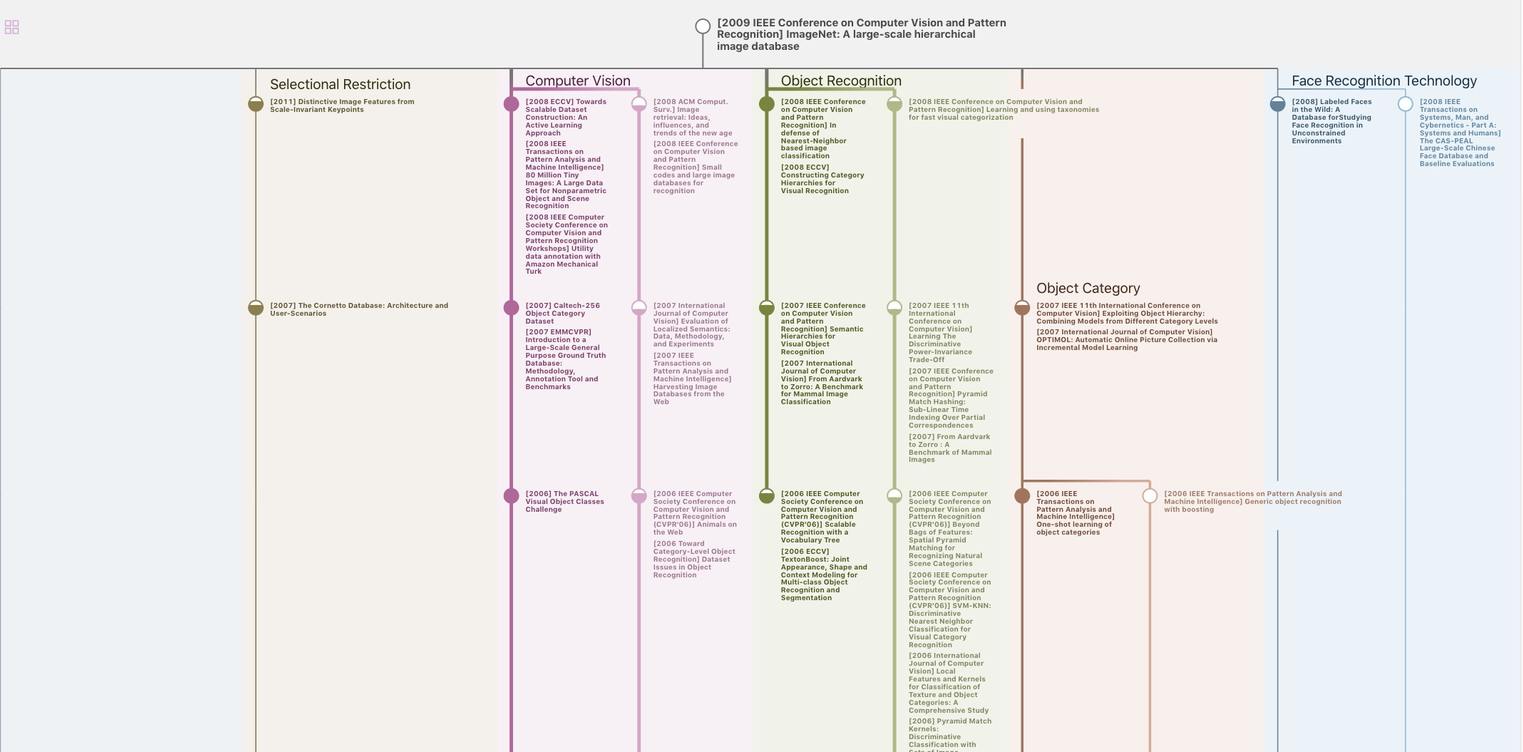
生成溯源树,研究论文发展脉络
Chat Paper
正在生成论文摘要