RFG-HELAD: A Robust Fine-Grained Network Traffic Anomaly Detection Model Based on Heterogeneous Ensemble Learning
IEEE Transactions on Information Forensics and Security(2024)
摘要
Fine-grained attack detection is an important network security task. A large number of machine learning/deep learning( ML/DL) based algorithms have been proposed. However, attacks not present in the training set pose a challenge to the model (openset problem). Further, ML/DL based models face the problem of adversarial attacks. Despite the large amount of work attempting to address these problems, there are still some challenges as follows. First, the open-set problem in fine-grained attack detection is difficult to solve because there is no effective representation of the distribution of unknown attacks. Second, in the open set environment, how the fine-grained attack detection model resists the adversarial attack is a more difficult problem. For example, the presence of unknown attacks poses a challenge for adversarial defense. For these reasons, we propose the RFG-HELAD model, which consists of a
K
classification model based on deep neural network (DNN) with contrastive learning (CL), and a
K
+ 1 classification model combining a generative adversarial networks (GAN) with two discriminators and deep
k
-nearest neighbors (Deep kNN). Among them, Deep kNN uses latent features from GAN and contrastive learning as input, which is essentially a distance-based out-of-distribution detection algorithm used to determine unknown attacks. The large category of unknown attacks has been added to the
K
classification, so it is a
K
+ 1 classification. To further improve the robustness of the RFG-HELAD model, we perform Fourier transform as well as feature fusion on the features, and also conduct adversarial training on the
K
classification model. Generative adversarial training of our GAN model can implicitly defend against adversarial attack. Experiments show that our model is superior to other state-of-the-art (SOTA) models in the presence of unknown attacks as well as under adversarial attacks. Especially, our model improves the accuracy by at least 18.7% over the corresponding SOTA model with adversarial defense. Further, we discuss the grounded deployment of the model and demonstrate its feasibility.
更多查看译文
关键词
Network anomaly detection,Adversarial attack,Unknown attack detection,Ensemble learning,Fine-grained attack detection
AI 理解论文
溯源树
样例
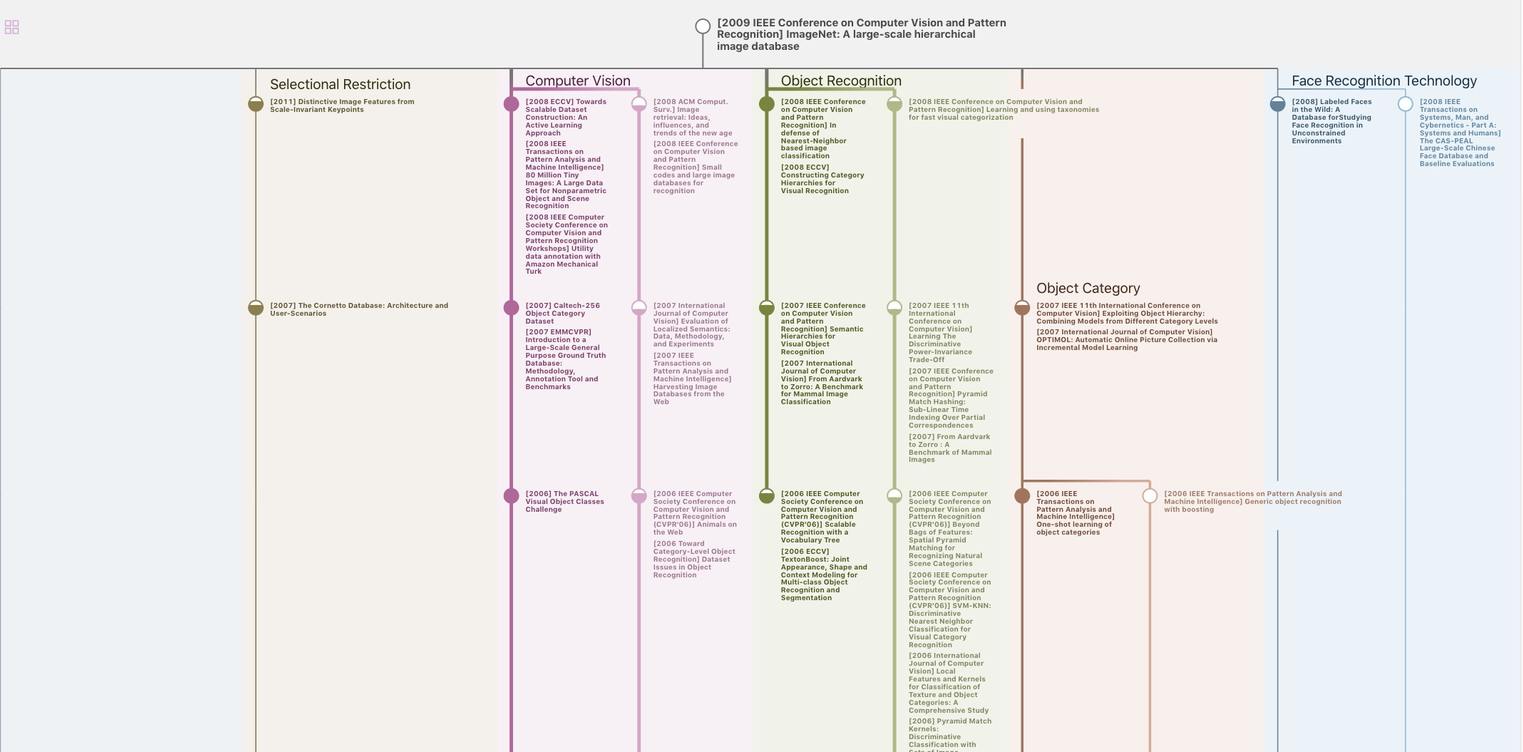
生成溯源树,研究论文发展脉络
Chat Paper
正在生成论文摘要