Uncertainty-aware Non-Invasive Patient–ventilator Asynchrony Detection Using Latent Gaussian Mixture Generative Classifier with Noisy Label Correction
International journal of data science and analytics(2024)
摘要
Patient–ventilator asynchrony (PVA) refers to instances where a mechanical ventilator’s cycles are desynchronised from the patient’s breathing efforts, and may result in patient discomfort and potential ineffective ventilation. Typically, they are identified with constant monitoring by trained clinicians. Such expertise is often limited; therefore, it is desirable to automate PVA detection with machine learning methods. However, there are three major challenges to applying machine learning to the problem: data collected from non-invasive ventilation are often noisy, there exists high variability between patients or between setting changes, and manual annotations of PVA events are not always consistent. To produce meaningful inference from such noisy data, a model needs to not only provide a measure of uncertainty, but also take into account potential inconsistencies in the training signal it is based on. In this work, we propose a conditional latent Gaussian mixture generative classifier with noisy label correction, which is capable of capturing variations within and between classes, providing well-calibrated class probabilities, detecting unlikely input instances that deviates from training data, while also taking into account possible mislabelling of event classes. We show that our model is able to match the performance of a well-tuned gradient boosting classifier, but also produce better calibrated predictions and smaller performance variability between patients.
更多查看译文
关键词
Patient–ventilator asynchrony,Generative classifier,Uncertainty quantification,Path signature
AI 理解论文
溯源树
样例
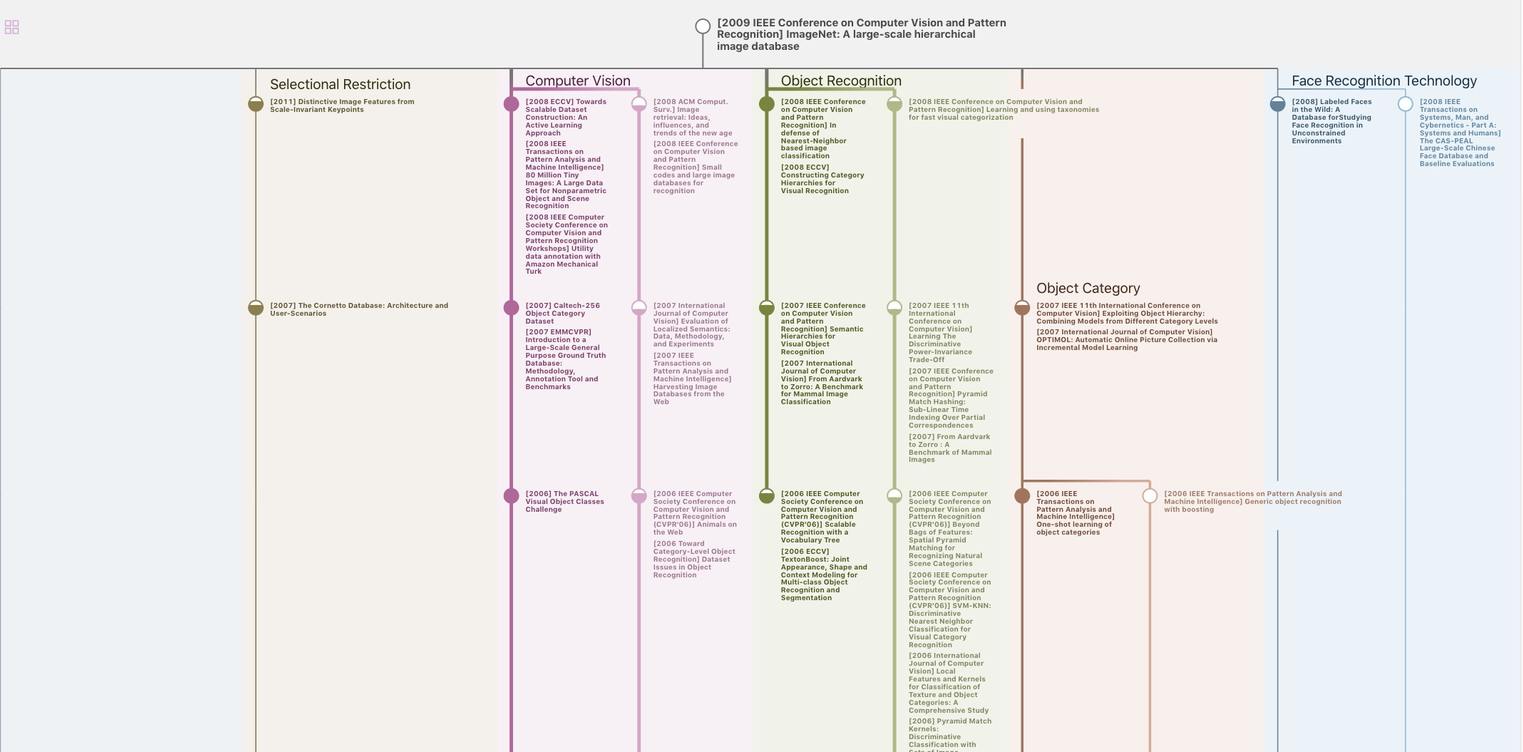
生成溯源树,研究论文发展脉络
Chat Paper
正在生成论文摘要