Learning Self- and Cross-Triplet Context Clues for Human-Object Interaction Detection
IEEE Transactions on Circuits and Systems for Video Technology(2024)
摘要
Human-Object Interaction (HOI) detection aims to infer interactions between humans and objects, and it is very important for scene analysis and understanding. The existing methods usually focus on exploring instance-level (e.g., object appearance) or interaction-level (e.g., action semantic) features to conduct interaction prediction. However, most of these methods only consider the self-triplet feature aggregation, which may lead to learning ambiguity without exploring the cross-triplet context exchange. In this paper, from both visual and textual perspectives, we propose a novel method to jointly explore self-and cross-triplet interaction context clues for HOI detection. First, we employ a graph neural network to perform self-triplet aggregation, where human and object features represent graph nodes and visual interaction feature and textual prior knowledge are acted as two different edges. Furthermore, we also attempt to explore cross-triplet context exchange by incorporating symbiotic and layout relationships among different HOI triplets. Extensive experiments on two benchmarks demonstrate that our proposed method outperforms the state-of-the-art ones and achieves the impressive performance of 40.32 mAP on HICO-DET and 69.1 mAP on V-COCO datasets, respectively.
更多查看译文
关键词
Human–object interaction,graph neural network,textual prior
AI 理解论文
溯源树
样例
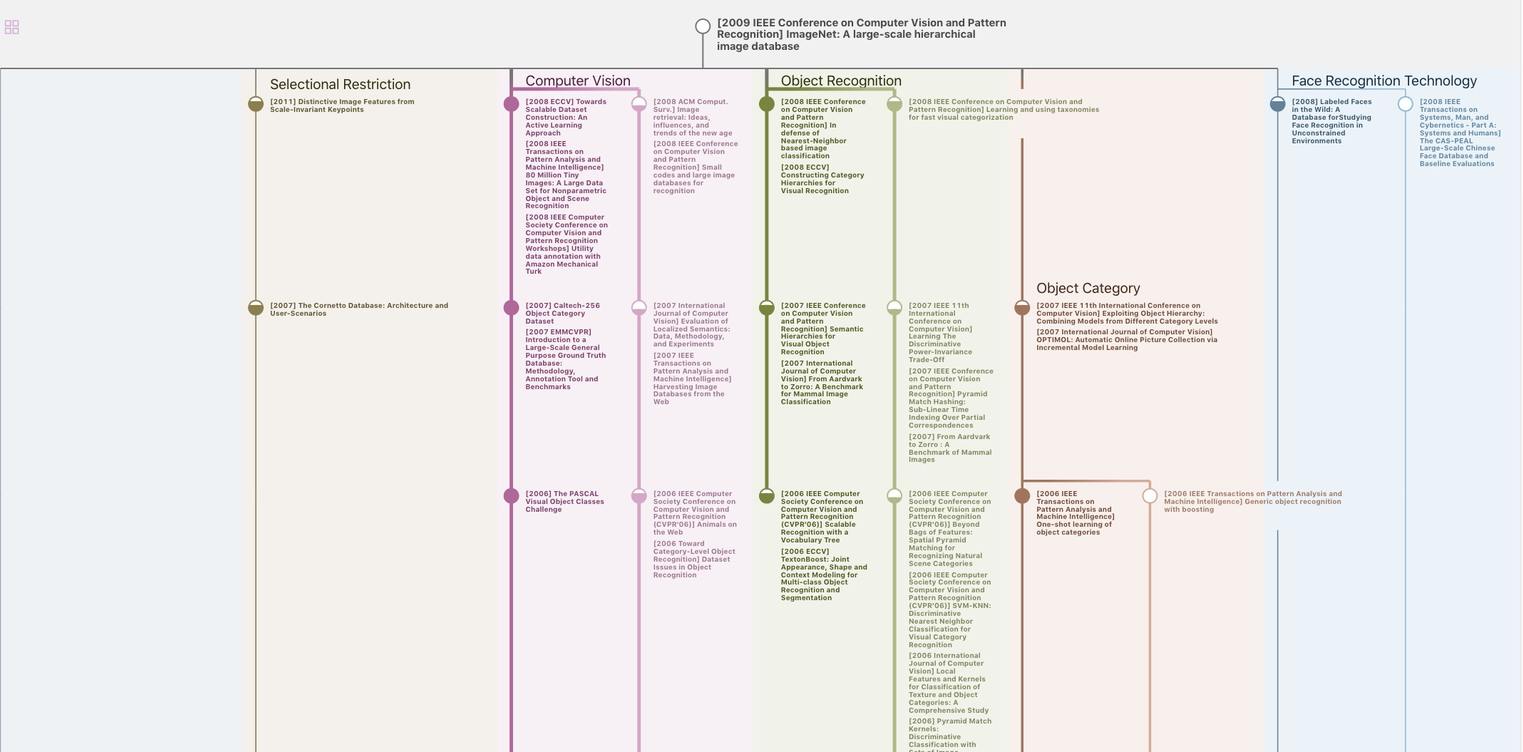
生成溯源树,研究论文发展脉络
Chat Paper
正在生成论文摘要