Multi-Task Learning for Near/Far Field Channel Estimation in STAR-RIS Networks
IEEE Transactions on Communications(2024)
摘要
A joint cascaded channel estimation scheme is proposed for simultaneously transmitting and reflecting reconfigurable intelligent surface (STAR-RIS) systems with hardware imperfections. In particular, the practical hybrid near- and far-field electromagnetic radiation with spatial non-stationarity is investigated. By exploiting the cascaded channel correlations between different users and between different STAR-RIS elements, a multi-task learning (MTL)-based channel estimation framework is proposed. This framework is capable of estimating the cascaded channels for transmission and reflection simultaneously based on noisy observations of the mixture channel. Following the design guideline of the proposed MTL framework, an efficient multi-task network (MTN) is developed to reconstruct the high-dimensional channels with limited pilot overhead. In the proposed MTN architecture, a mixed convolution and multilayer perception module is exploited to capture the effective hybrid-field channel features. This module integrates the locality bias modeling of the channel-wise convolution and the long-range dependency modeling of MLP, which finely learns both local spatial correlations and specific spatial non-stationarity of the hybrid-field cascaded channels. Numerical results show that the proposed MTN achieves superior channel estimation accuracy with less training overhead compared with the existing state-of-the-art benchmarks, in terms of required pilots, computations, and network parameters.
更多查看译文
关键词
Channel estimation,multi-task learning,reconfigurable intelligent surfaces,simultaneous transmission and reflection
AI 理解论文
溯源树
样例
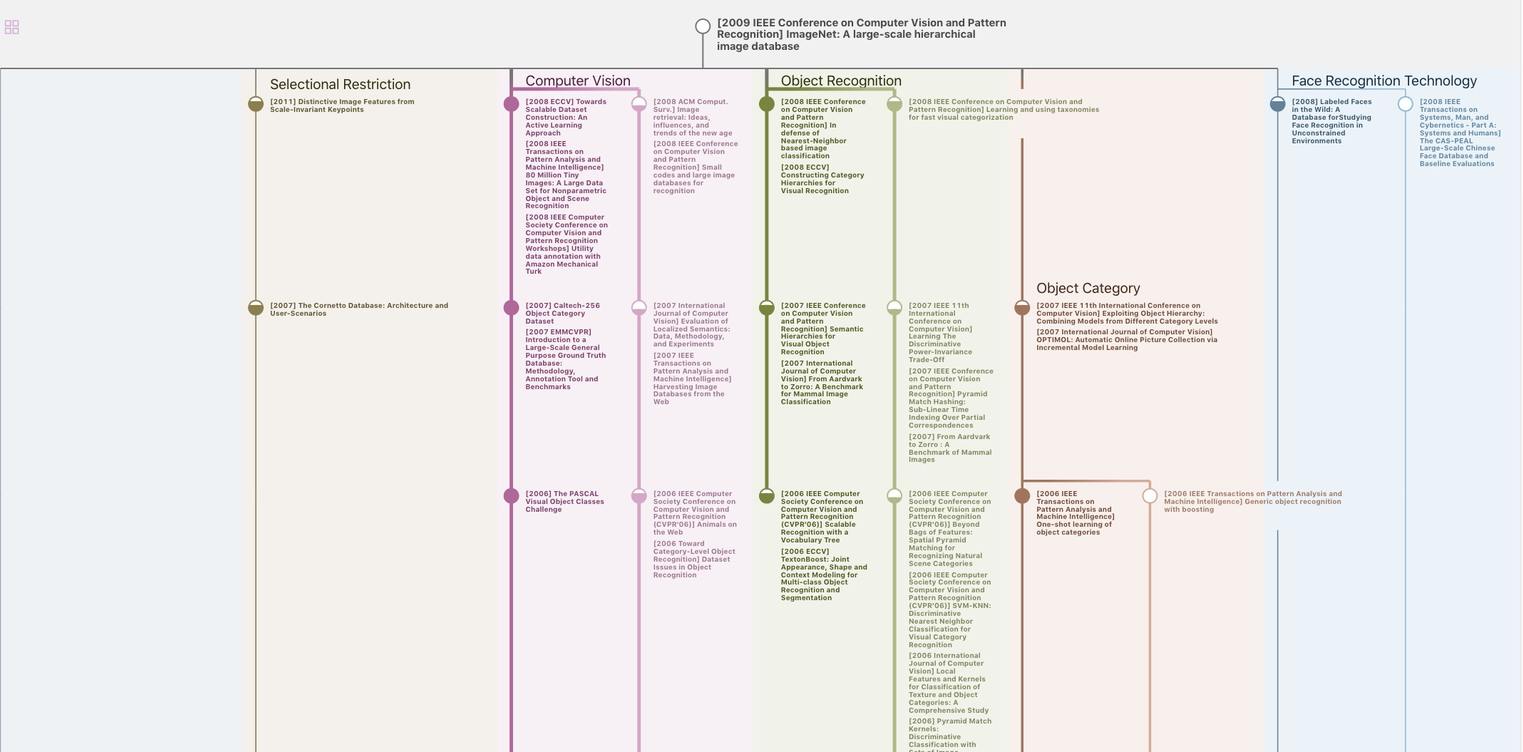
生成溯源树,研究论文发展脉络
Chat Paper
正在生成论文摘要