SC-CNN: LiDAR point cloud filtering CNN under slope and copula correlation constraint
ISPRS Journal of Photogrammetry and Remote Sensing(2024)
摘要
To tackle the issue of lack of semantic consistency between ground and non-ground points, as well as the damage to the integrity of terrain boundary information during network downsampling, we developed a Semantic Consistency-Convolutional Neural Network (SC-CNN) to improve the precision of point cloud filtering under complex terrain conditions. The novel aspects include: (1) farthest point sampling (FPS) with slope constraints, which enhances terrain contour preservation through adaptive subblock partitioning and slope-based sampling; (2) intra-class feature enhancement via copula correlation and attention mechanisms, improving the network’s ability to distinguish between ground and non-ground points by focusing on intra-class feature consistency and inter-class differences; and (3) filter error correction using copula correlation and confidence intervals. refining filtering accuracy by adjusting for negatively correlated point sets. Tested on the ISPRS and 3D Vaihingen datasets, SC-CNN notably outperformed existing methods, reducing the mean total error (MT.E) by 0.17% and 1.93%, respectively, thereby significantly enhancing point-cloud filtering accuracy under complex terrain conditions.
更多查看译文
AI 理解论文
溯源树
样例
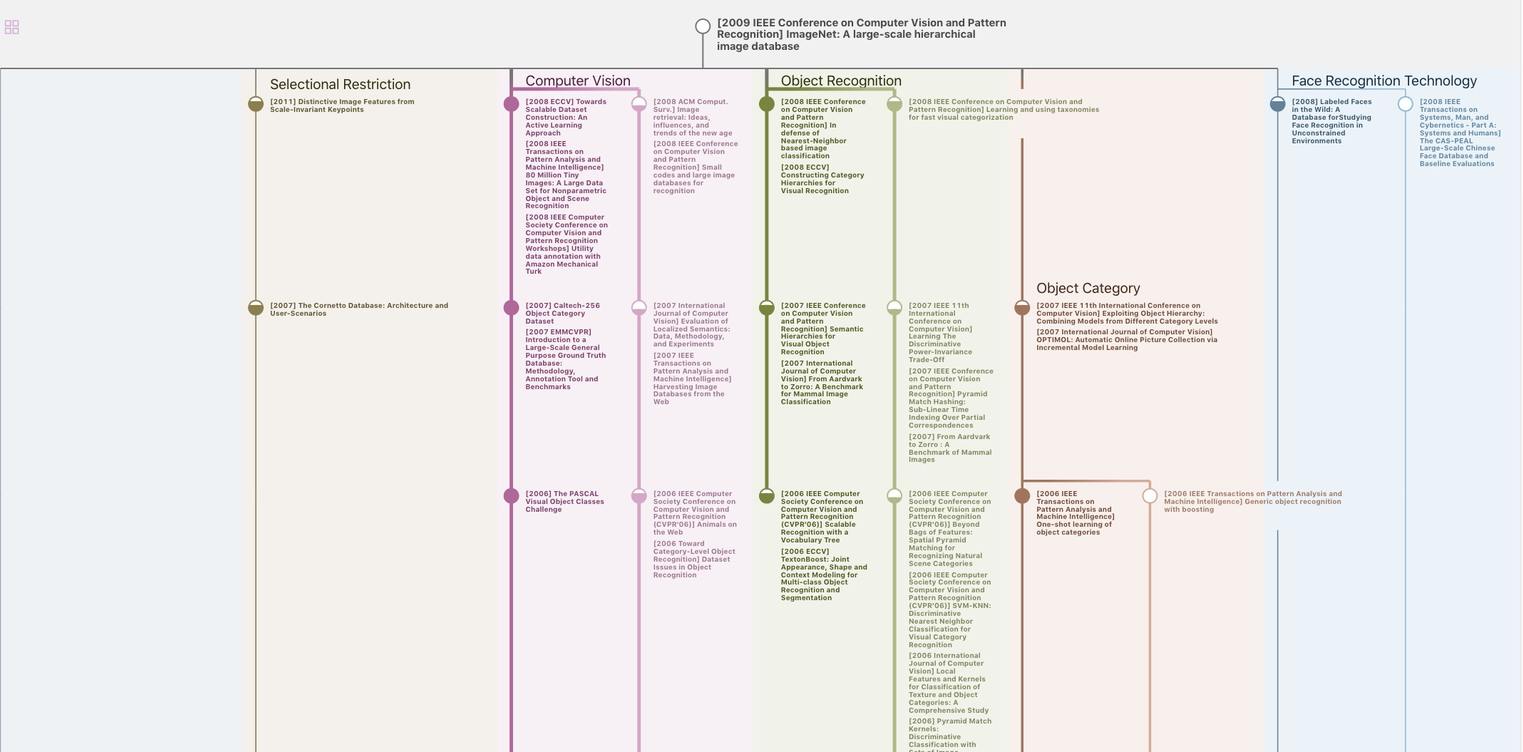
生成溯源树,研究论文发展脉络
Chat Paper
正在生成论文摘要