FieldSeg-DA2.0: Further Enhancing the Spatiotemporal Transferability of an Individual Arable Field (IAF) Extraction Network Using Multisource Remote Sensing and Land Cover Data
Computers and electronics in agriculture(2024)
摘要
Deep learning has become the leading technique for precisely extracting individual arable fields (IAFs) from high-resolution remote sensing images. Maintaining transferability remains a major concern for deep learning methodologies due to the significant cost of acquiring labelled samples for network training. FieldSeg-DA, introduced by Liu et al. (2022), enhanced network transferability by introducing a finely tuned adversarial domain adaptation module (FADA). An improved version of the FieldSeg-DA framework, FieldSeg-DA2.0, which further enhances transferability through incorporating multisource remote sensing and land cover data is introduced in this paper. First, we introduce a spatiotemporal fusion module, U-LSTM, to extract the IAF extent by merging textural information from the high-resolution image (Gaofen-2) and phenological information from the coarse time-series data (Sentinel-2). Incorporating phenological information mitigates the risk of overfitting associated with the high-resolution imagery acquired in specific seasons, thereby improving the temporal transferability of FieldSeg-DA2.0 compared to FieldSeg-DA. Second, we introduce a novel fine-grained adversarial domain adaptation module with ancillary data (FADA-A) to enhance spatial transferability. FADA-A incorporates prior knowledge from the Dynamic World (DW) land cover dataset to guide adversarial training in the standard FADA, thereby enhancing the robustness of domain adaptation across diverse geographic regions. We evaluate the performance of FieldSeg-DA2.0 through various spatial and temporal transfer experiments utilizing GaoFen-2 and Sentinel-2 data. The results illustrate that the cross-domain performance of FieldSeg-DA2.0 is significantly better than that of the original FieldSeg-DA, highlighting its robust spatiotemporal transferability. FieldSeg-DA2.0 can accurately delineate IAFs across varied regions and seasons without requiring additional training samples, illustrating its considerable potential for large-scale IAF extraction.
更多查看译文
关键词
Individual arable field,Spatiotemporal transferability,Spatiotemporal fusion,Adversarial domain adaptation,Land cover prior
AI 理解论文
溯源树
样例
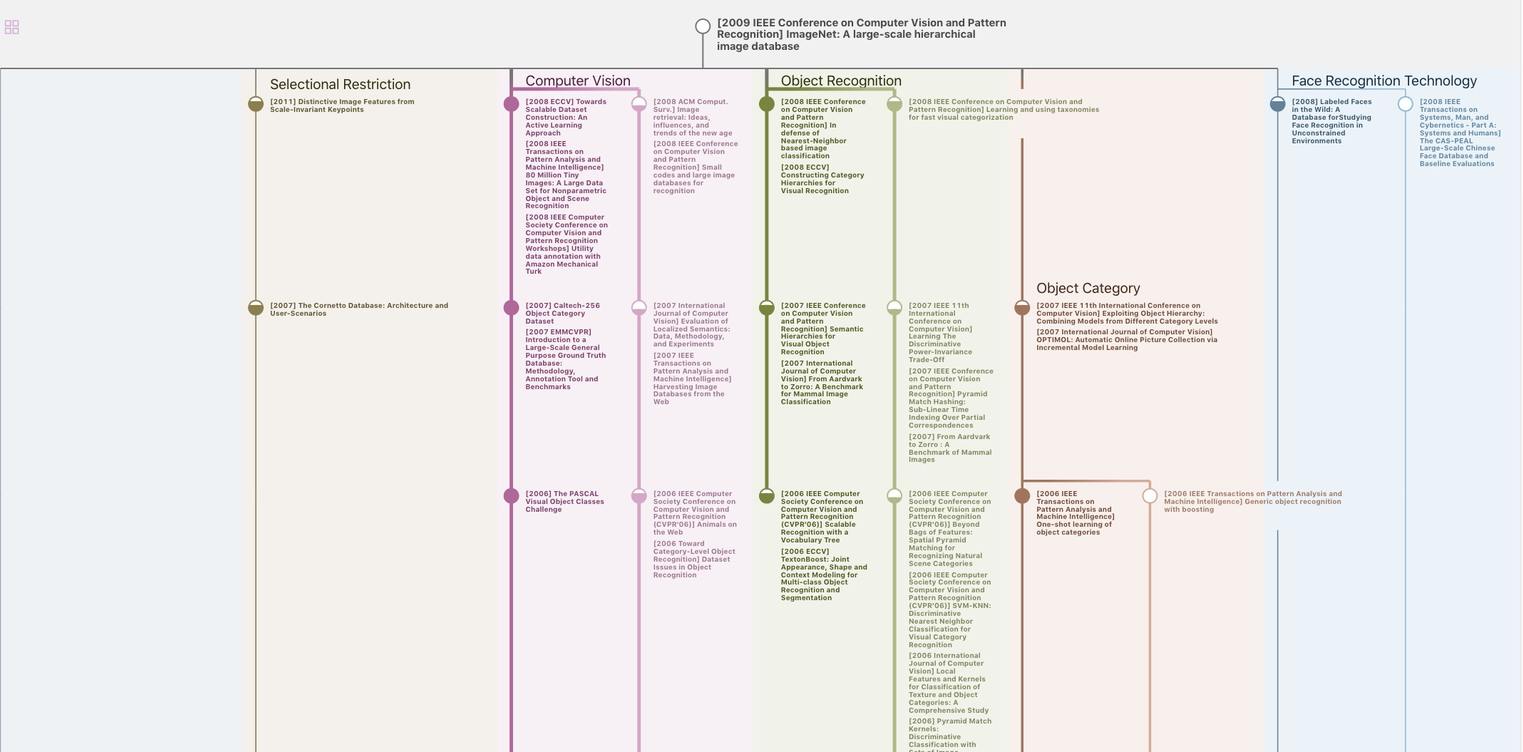
生成溯源树,研究论文发展脉络
Chat Paper
正在生成论文摘要