IT2F-SEDNN: an interval type-2 fuzzy logic-based stacked ensemble deep learning approach for early phase software dependability analysis
Innovations in Systems and Software Engineering(2024)
摘要
The growing size, complexity of software systems, and complex development process pose a difficult challenge in predicting dependability attributes during the early stages of development. Key attributes such as reliability, security, maintainability, availability, and aging play a critical role in defining the dependability of software modules. Therefore, accurate prediction of dependability attributes is of utmost importance. Existing prediction methodologies often rely on machine learning models. Software metrics are used in these studies as input for predicting dependability attributes. However, metric values can become uncertain, and machine learning models cannot address uncertainty issues of metric values. Due to the presence of uncertainty, the vulnerability or different types of defects may get overlooked during the early phase of development. Addressing this issue, this study proposes a type-2 fuzzy logic (T2FL)-based model to estimate dependability attributes effectively during an early phase. The proposed approach has leveraged human expertise in developing a rule base for the proposed T2FL model. A neural network-based approach called stacked deep neural network (SDNN) has also been proposed to analyze the impact of dependability attributes on software modules. SDNN uses the estimated dependability attributes as input, trains various architecture-based deep neural networks to classify software modules, and ensemble them to achieve better and more robust solutions. Two simulation studies have been carried out to validate the proposed methodology. The performance of the proposed approach achieves better accuracy than other baseline models. This proposed approach aims to assist software developers in developing dependable software in scheduled time with optimized costs.
更多查看译文
关键词
Software dependability,Faults,Interval type-2 fuzzy inference system (IT2FIS),Deep neural network
AI 理解论文
溯源树
样例
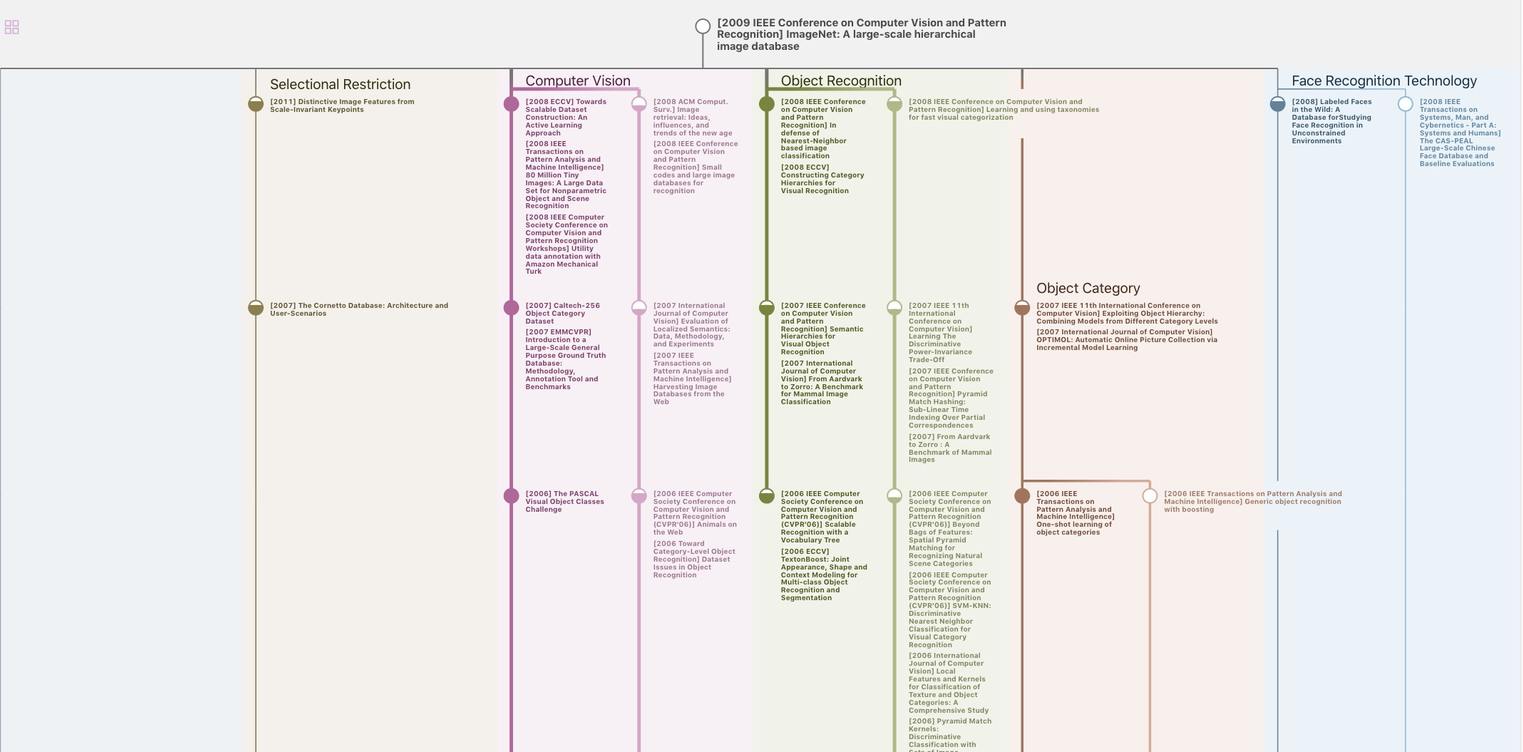
生成溯源树,研究论文发展脉络
Chat Paper
正在生成论文摘要