Edge Server Enhanced Secure and Privacy Preserving Federated Learning
COMPUTER NETWORKS(2024)
摘要
Federated learning (FL) has been smoothly embedded into current IoT edge computing architecture, and hatching advanced IoT-FL applications. While so, sensitive messages generated on terminal sides brings privacy issues; and vulnerable terminal devices are easy targets for Byzantine adversaries, they inject malicious data to manipulate the IoT-FL system. Now an inherent dilemma is, the privacy preserving requires anonymous indistinguishable individual characteristics, while the malicious adversary detection requires transparent distinguishable results. Existing fragmented schemes are not able to handle both problems coherently. This time, inherit our previous study, based on the IoT edge computing architecture, we utilize Homomorphic Encryption (HE) and Threshold Secret Sharing (SS) methods, propose a joint scheme (namely, Sec-IoTFL) for anonymous adversary detection. Specifically, we batch encode the clients' training result vectors (message) as polynomials, and split them as secret pieces with a unified SS key set; then edge server and cloud server joint deal with these secret pieces in a specialized flow, where Byzantine adversaries will be filtered out. The theoretical analysis and solid experimental results suggest, in our scheme, local sensitive data is well preserved, and malicious behavior is precisely screened. Comparing with traditional methods, our Sec-IoTFL scheme shows the superiority in accuracy and efficiency.
更多查看译文
关键词
Adversary detection,Edge computing,IoT-FL,Privacy preserving
AI 理解论文
溯源树
样例
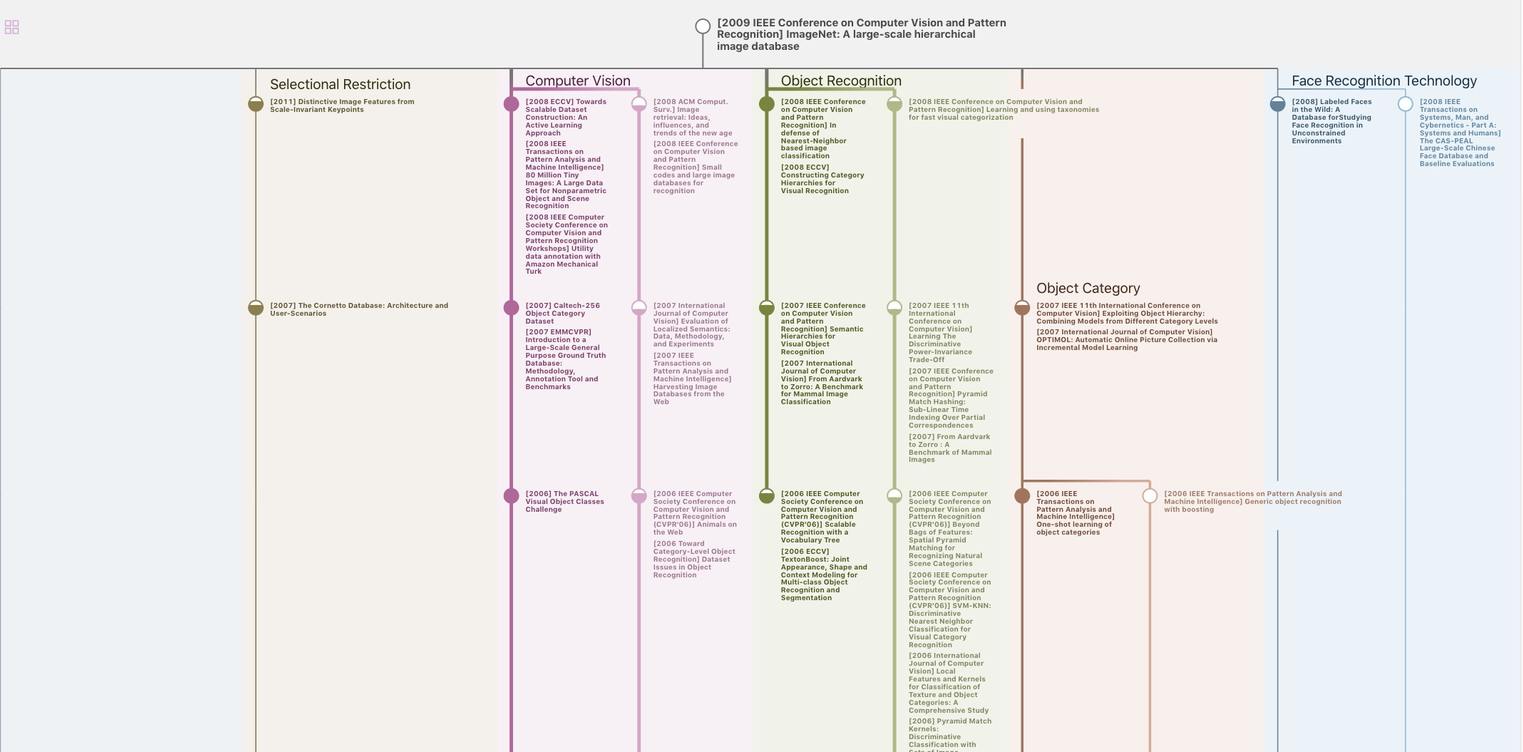
生成溯源树,研究论文发展脉络
Chat Paper
正在生成论文摘要