An Explainable Machine Learning Approach Using Contemporary UNOS Data to Identify Patients Who Fail to Bridge to Heart Transplantation
Frontiers in cardiovascular medicine(2024)
摘要
BackgroundThe use of Intra-aortic Balloon Pump (IABP) and Impella devices as a bridge to heart transplantation (HTx) has increased significantly in recent times. This study aimed to create and validate an explainable machine learning (ML) model that can predict the failure of status two listings and identify the clinical features that significantly impact this outcome.MethodsWe used the UNOS registry database to identify HTx candidates listed as UNOS Status 2 between 2018 and 2022 and supported with either Impella (5.0 or 5.5) or IABP. We used the eXtreme Gradient Boosting (XGBoost) algorithm to build and validate ML models. We developed two models: (1) a comprehensive model that included all patients in our cohort and (2) separate models designed for each of the 11 UNOS regions.ResultsWe analyzed data from 4,178 patients listed as Status 2. Out of them, 12% had primary outcomes indicating Status 2 failure. Our ML models were based on 19 variables from the UNOS data. The comprehensive model had an area under the curve (AUC) of 0.71 (±0.03), with a range between 0.44 (±0.08) and 0.74 (±0.01) across different regions. The models' specificity ranged from 0.75 to 0.96. The top five most important predictors were the number of inotropes, creatinine, sodium, BMI, and blood group.ConclusionUsing ML is clinically valuable for highlighting patients at risk, enabling healthcare providers to offer intensified monitoring, optimization, and care escalation selectively.
更多查看译文
关键词
heart transplantation,machine learning,UNOS,intra-aortic balloon pump,Impella
AI 理解论文
溯源树
样例
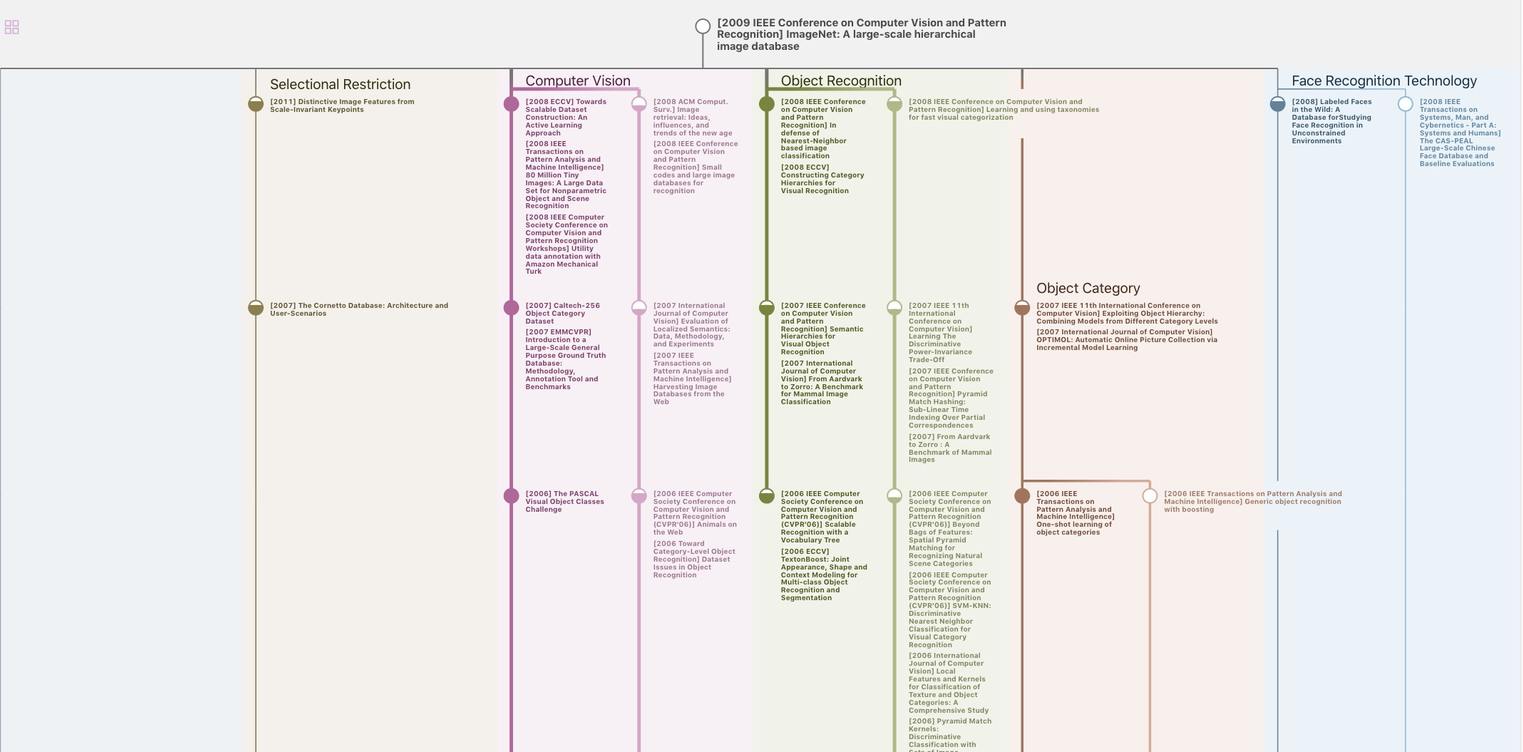
生成溯源树,研究论文发展脉络
Chat Paper
正在生成论文摘要