Evaluation of enzyme activity predictions for variants of unknown significance in Arylsulfatase A.
bioRxiv : the preprint server for biology(2024)
摘要
Continued advances in variant effect prediction are necessary to demonstrate the ability of machine learning methods to accurately determine the clinical impact of variants of unknown significance (VUS). Towards this goal, the ARSA Critical Assessment of Genome Interpretation (CAGI) challenge was designed to characterize progress by utilizing 219 experimentally assayed missense VUS in the Arylsulfatase A (ARSA) gene to assess the performance of community-submitted predictions of variant functional effects. The challenge involved 15 teams, and evaluated additional predictions from established and recently released models. Notably, a model developed by participants of a genetics and coding bootcamp, trained with standard machine-learning tools in Python, demonstrated superior performance among submissions. Furthermore, the study observed that state-of-the-art deep learning methods provided small but statistically significant improvement in predictive performance compared to less elaborate techniques. These findings underscore the utility of variant effect prediction, and the potential for models trained with modest resources to accurately classify VUS in genetic and clinical research.
更多查看译文
AI 理解论文
溯源树
样例
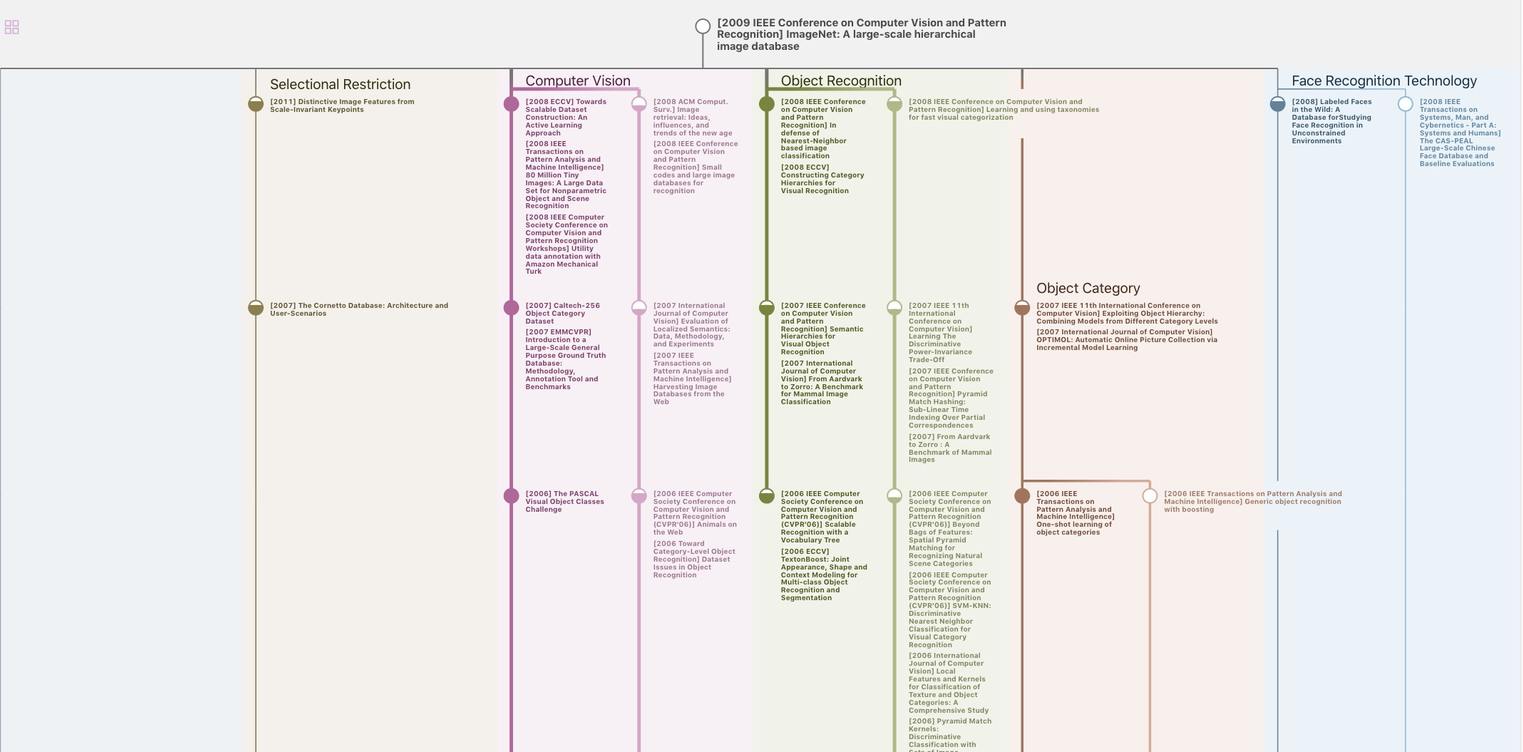
生成溯源树,研究论文发展脉络
Chat Paper
正在生成论文摘要