Towards Real-world Violence Recognition Via Efficient Deep Features and Sequential Patterns Analysis
Mobile Networks and Applications(2024)
摘要
Surveillance video analysis using automated AI-based techniques is a prominent research field with real-world applications. Several techniques aiming at recognizing activities, behaviour, and violent actions are present in literature. Real-world data analysis for violence detection is still a major challenge due to the limited datasets available for training with complex scenarios and varied scaling of objects performing different activities. In this paper, we present a novel deep learning model considering specially designed frame encoders for spatial feature extraction that are generalized towards many challenges, such as light conditions and indoor and outdoor scenarios. Furthermore, the spatial features in the stacked form are analyzed using a temporal deep learning model to observe and learn the temporal patterns dependencies by considering past and future information while predicting the violent or normal class. In the literature, violence is considered a binary classification of either fight or no fight. Different from these techniques, we present a multi-class classification of violent activities considering different types of human violence, such as assault, shooting, etc. The data for multi-class violence classification is extracted from the famous real-world anomaly detection UCF-crime dataset, where only six human-involved types of anomalies are considered for preparing training and testing sets. We report 41.1
更多查看译文
关键词
Violence detection,Violence recognition,Deep learning,Convolutional neural networks,Activity recognition
AI 理解论文
溯源树
样例
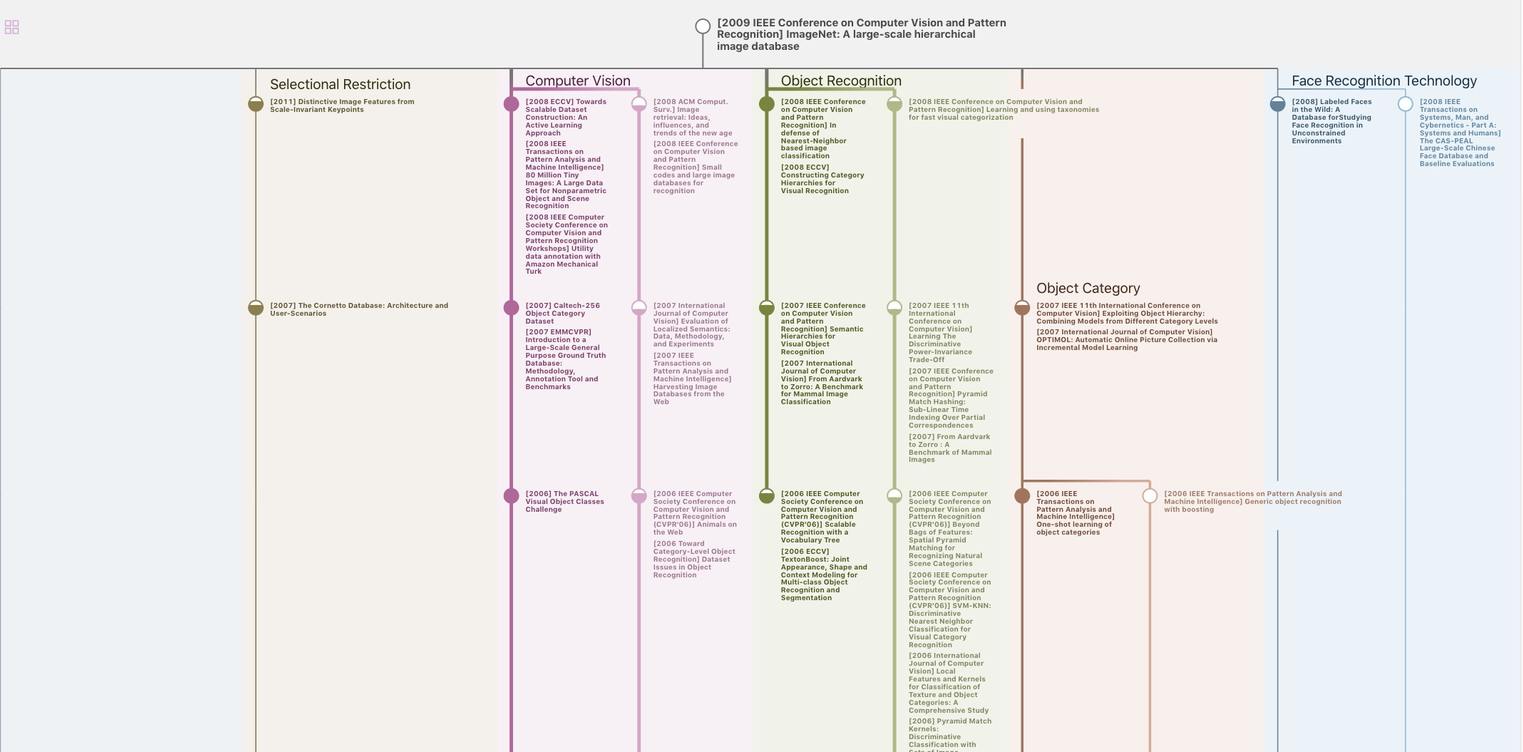
生成溯源树,研究论文发展脉络
Chat Paper
正在生成论文摘要