ARAIDA: Analogical Reasoning-Augmented Interactive Data Annotation
arXiv (Cornell University)(2024)
摘要
Human annotation is a time-consuming task that requires a significant amountof effort. To address this issue, interactive data annotation utilizes anannotation model to provide suggestions for humans to approve or correct.However, annotation models trained with limited labeled data are prone togenerating incorrect suggestions, leading to extra human correction effort. Totackle this challenge, we propose Araida, an analogical reasoning-basedapproach that enhances automatic annotation accuracy in the interactive dataannotation setting and reduces the need for human corrections. Araida involvesan error-aware integration strategy that dynamically coordinates an annotationmodel and a k-nearest neighbors (KNN) model, giving more importance to KNN'spredictions when predictions from the annotation model are deemed inaccurate.Empirical studies demonstrate that Araida is adaptable to different annotationtasks and models. On average, it reduces human correction labor by 11.02compared to vanilla interactive data annotation methods.
更多查看译文
AI 理解论文
溯源树
样例
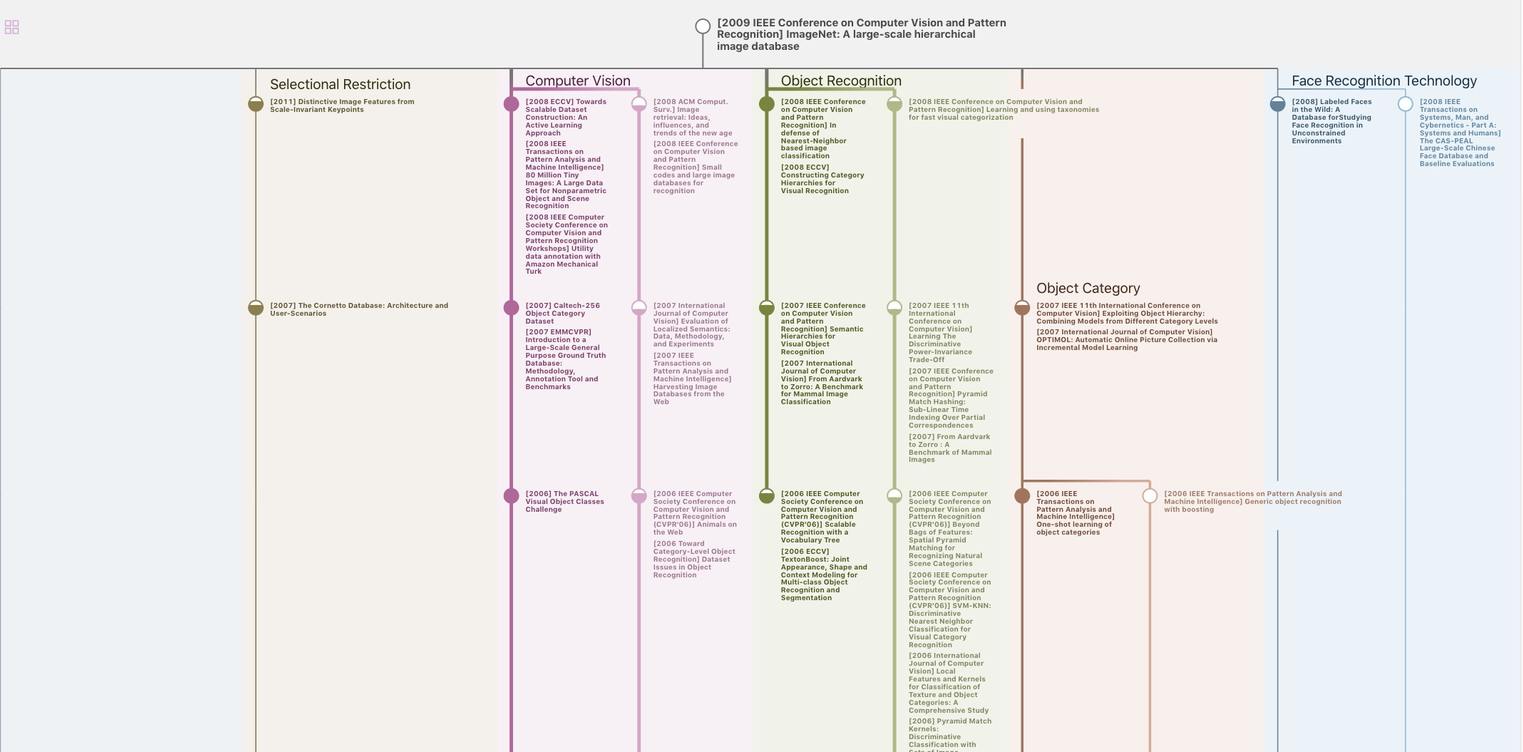
生成溯源树,研究论文发展脉络
Chat Paper
正在生成论文摘要