Semi-Supervised Contrastive Learning for Time Series Classification in Healthcare
IEEE TRANSACTIONS ON EMERGING TOPICS IN COMPUTATIONAL INTELLIGENCE(2024)
关键词
Time series analysis,Data models,Feature extraction,Medical services,Training,Biomedical monitoring,Task analysis,Contrastive learning,EEG,healthcare,medical time series,semi-supervised
AI 理解论文
溯源树
样例
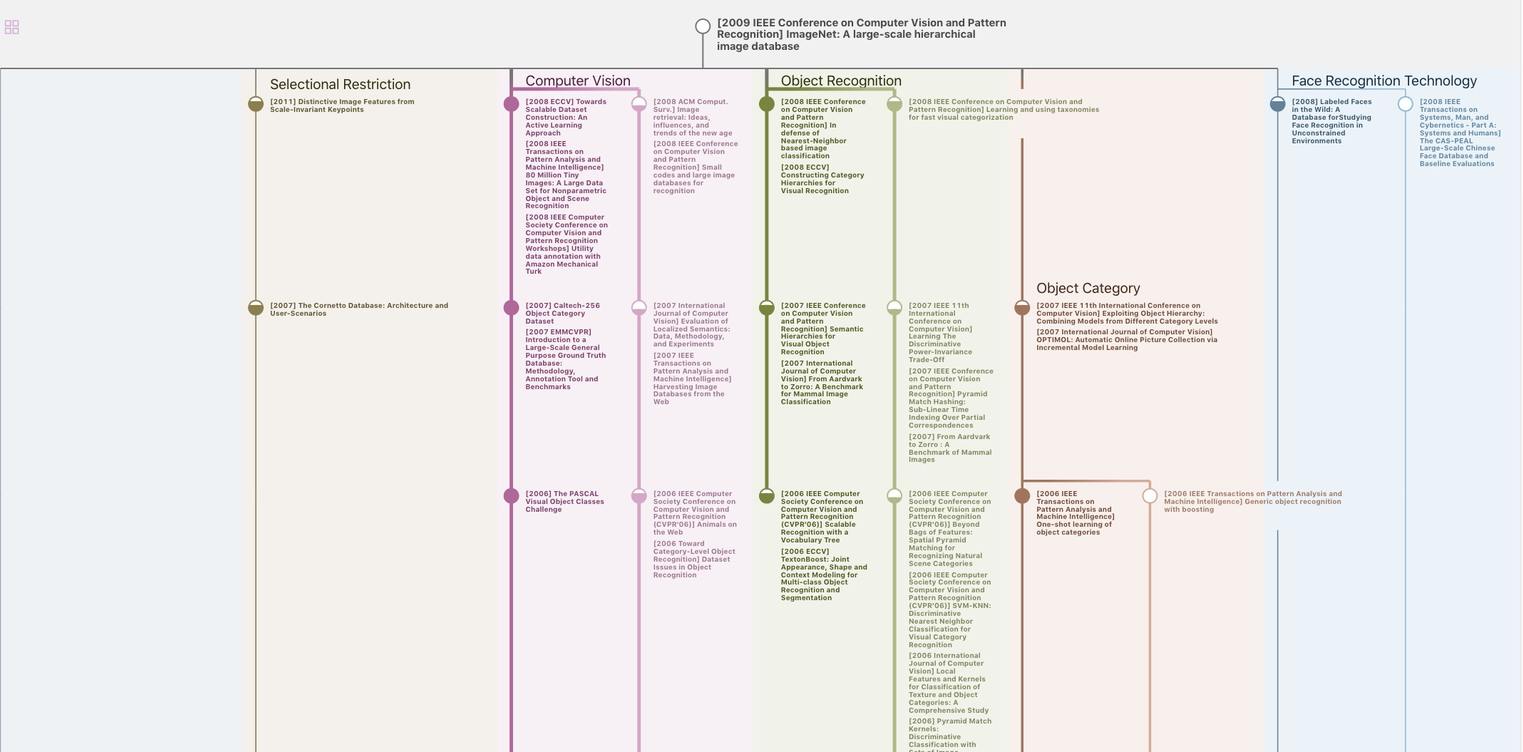
生成溯源树,研究论文发展脉络
Chat Paper
正在生成论文摘要