Large Language Models Can Infer Personality from Free-Form User Interactions
crossref(2024)
摘要
This study investigates the capacity of Large Language Models (LLMs) to infer the Big Five personality traits from free-form user interactions. The results demonstrate that a chatbot powered by GPT-4 can infer personality with moderate accuracy, outperforming previous approaches drawing inferences from static text content. The accuracy of inferences varied across different conversational settings. Performance was highest when the chatbot was prompted to elicit personality-relevant information from users (mean r=.443, range=[.245, .640]), followed by a condition placing greater emphasis on naturalistic interaction (mean r=.218, range=[.066, .373]). Notably, the direct focus on personality assessment did not result in a less positive user experience, with participants reporting the interactions to be equally natural, pleasant, engaging, and humanlike across both conditions. A chatbot mimicking ChatGPT’s default behavior of acting as a helpful assistant led to markedly inferior personality inferences and lower user experience ratings but still captured psychologically meaningful information for some of the personality traits (mean r=.117, range=[-.004, .209]). Preliminary analyses suggest that the accuracy of personality inferences varies only marginally across different socio-demographic subgroups. Our results highlight the potential of LLMs for psychological profiling based on conversational interactions. We discuss practical implications and ethical challenges associated with these findings.
更多查看译文
AI 理解论文
溯源树
样例
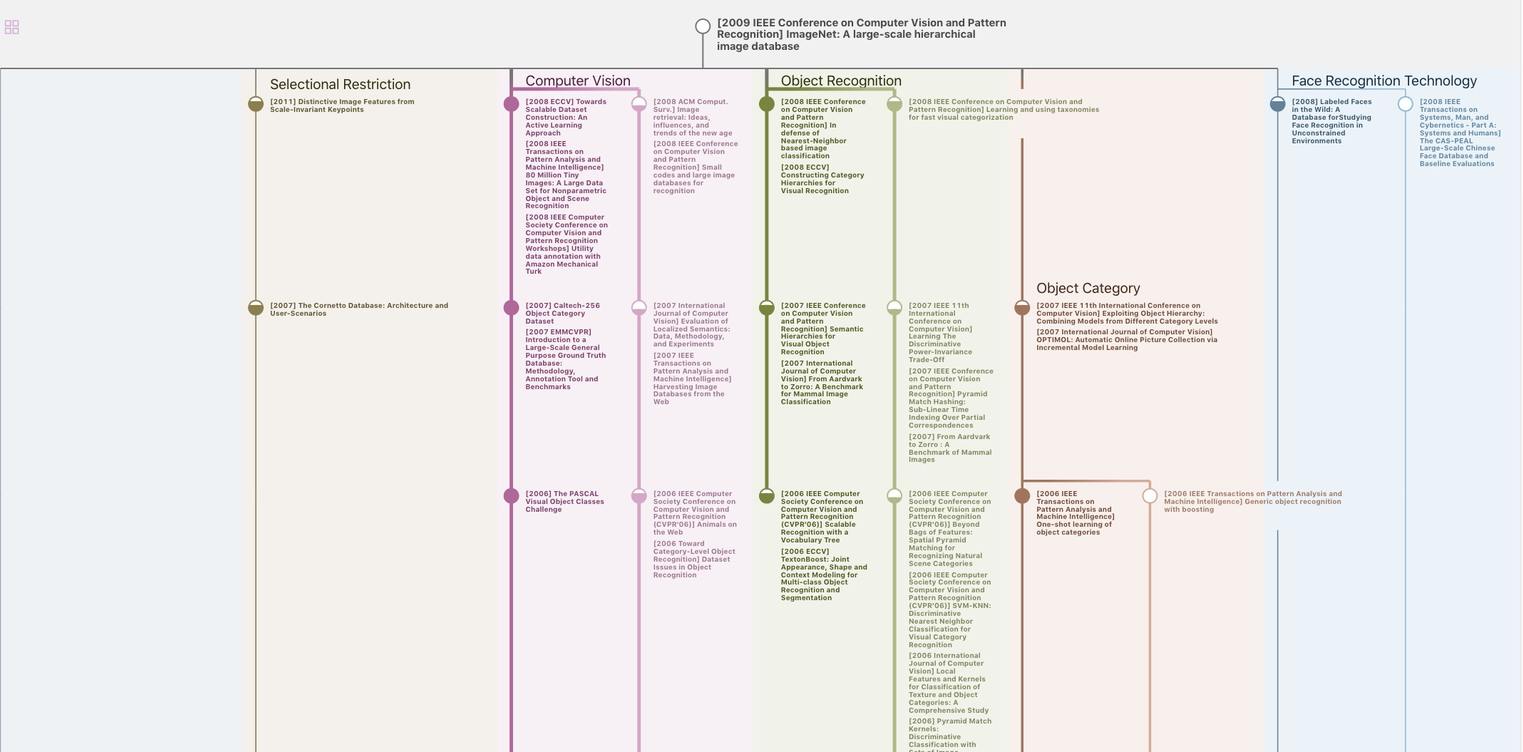
生成溯源树,研究论文发展脉络
Chat Paper
正在生成论文摘要