A Saliency Map Approach to Optimize VMAF for Video and Image Compression
2024 DATA COMPRESSION CONFERENCE, DCC(2024)
Abstract
The Video Multi-method Assessment Fusion (VMAF) has demonstrated a better correlation with Human Visual System than the conventional objective metrics, and has gradually gained adoption in the industry that needs to monitor the visual quality of compressed videos. However, due to its machine learning nature, it can not be expressed through a simple and explicit mathematical formula, which makes it difficult to incorporate VMAF into the rate-distortion optimization framework in video compression. In this work, we propose a new perspective that decomposes the VMAF as a superposition of spatial and temporal factors. The spatial factor, which also directly applies to image quality evaluation, is approximated by a saliency map. It in conjunction with the temporal factor approximated by the motion quantities allows a simple analytical formula that translates the mean squared distortion at each pixel to its impact to the overall VMAF metric. The proposed hypothesis is embedded into the rate-distortion optimization framework, and is experimentally shown to provide considerable coding gains in VMAF for both image and video compression.
MoreTranslated text
Key words
Saliency map,VMAF,rate-distortion optimization
求助PDF
上传PDF
View via Publisher
AI Read Science
AI Summary
AI Summary is the key point extracted automatically understanding the full text of the paper, including the background, methods, results, conclusions, icons and other key content, so that you can get the outline of the paper at a glance.
Example
Background
Key content
Introduction
Methods
Results
Related work
Fund
Key content
- Pretraining has recently greatly promoted the development of natural language processing (NLP)
- We show that M6 outperforms the baselines in multimodal downstream tasks, and the large M6 with 10 parameters can reach a better performance
- We propose a method called M6 that is able to process information of multiple modalities and perform both single-modal and cross-modal understanding and generation
- The model is scaled to large model with 10 billion parameters with sophisticated deployment, and the 10 -parameter M6-large is the largest pretrained model in Chinese
- Experimental results show that our proposed M6 outperforms the baseline in a number of downstream tasks concerning both single modality and multiple modalities We will continue the pretraining of extremely large models by increasing data to explore the limit of its performance
Upload PDF to Generate Summary
Must-Reading Tree
Example
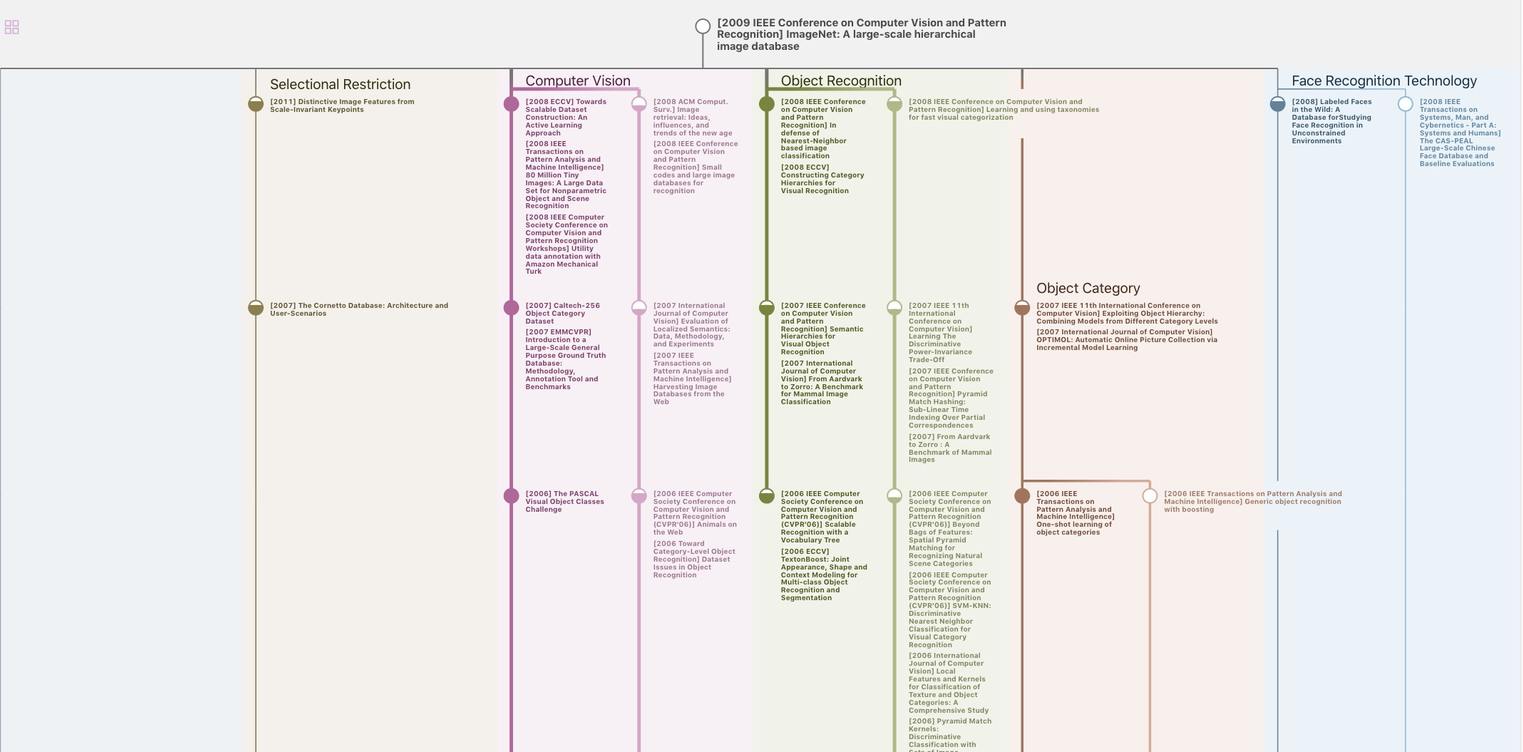
Generate MRT to find the research sequence of this paper
Related Papers
2010
被引用247 | 浏览
2019
被引用24 | 浏览
Data Disclaimer
The page data are from open Internet sources, cooperative publishers and automatic analysis results through AI technology. We do not make any commitments and guarantees for the validity, accuracy, correctness, reliability, completeness and timeliness of the page data. If you have any questions, please contact us by email: report@aminer.cn
Chat Paper
GPU is busy, summary generation fails
Rerequest