A Convolutional Neural Network Classifies Beat-to-Beat Arterial Pressure Spectrograms and Wavelet Transforms According to Age, Sex, and Metabolic State: Novel Frequencies for Cardiovascular Risk Appraisal
PHYSIOLOGY(2024)
摘要
Hemodynamic homeostasis is under the control of multiple systems. Continuous blood pressure (BP) time series carry information relevant to the pathophysiological status of the cardiovascular system. Beside average BP, different measures of variability provide prognostic value for distinguishing between cardiovascular risk states. The latter are derived from time- and frequency- domain analysis of BP recordings and are limited by their capacity to provide cumulative risk appraisal among different risk groups. The prediabetic state is associated with cardiovascular risk which is often described to be age- and sex-specific. In this study, we sought to determine distinctive features of continuous arterial pressure (AP) time-series for risk appraisal in a pre-established prediabetic rat model. To overcome the shortcomings associated with conventional BP variability parameters, we trained a convolutional neural network (CNN) using spectrograms and scalograms generated from short-term Fourier- and Morlet wavelet- transforms of AP time series, respectively, from male and female prediabetic rats fed a mild hypercaloric diet for 12- or 24- weeks and their corresponding controls. The CNN consisted of 5 convolutional layers, separated by batch normalization, ReLU activation, and max pooling. The outcome of the fifth convolutional layer had 40% of the data dropped out and delivered to a fully-connected dense layer and then to a softmax function. The data was divided into 80% training, 10% validation, and 10% test sets. The model classified spectrograms and scalograms with test accuracy of 90%+ for the different binary and multi-class classification tasks. On backward propagation of model scores, different salient frequencies were identified as critical features for classification of AP time-series. The identified frequencies were much higher than those typically used in conventional power spectral density (PSD) analysis. Using 3-way ANOVA or principal component analysis for comparison and clustering of the different cardiovascular risk groups of age, sex, and metabolic disease, respectively, according to PSD at the identified peaks does not yield suffcient risk appraisal. This indicates that the CNN possibly captures non-linear relations which are undetectable using inherently linear methods.The results indicate sex- and age-specific patterns of cardiovascular deterioration in prediabetes. Use of artificial intelligence models for the identification of abnormal BP fluctuation patterns can provide suffcient risk appraisal needed for early intervention, particularly in at-risk patients lacking clinical signs of overt cardiovascular disease. None. This is the full abstract presented at the American Physiology Summit 2024 meeting and is only available in HTML format. There are no additional versions or additional content available for this abstract. Physiology was not involved in the peer review process.
更多查看译文
AI 理解论文
溯源树
样例
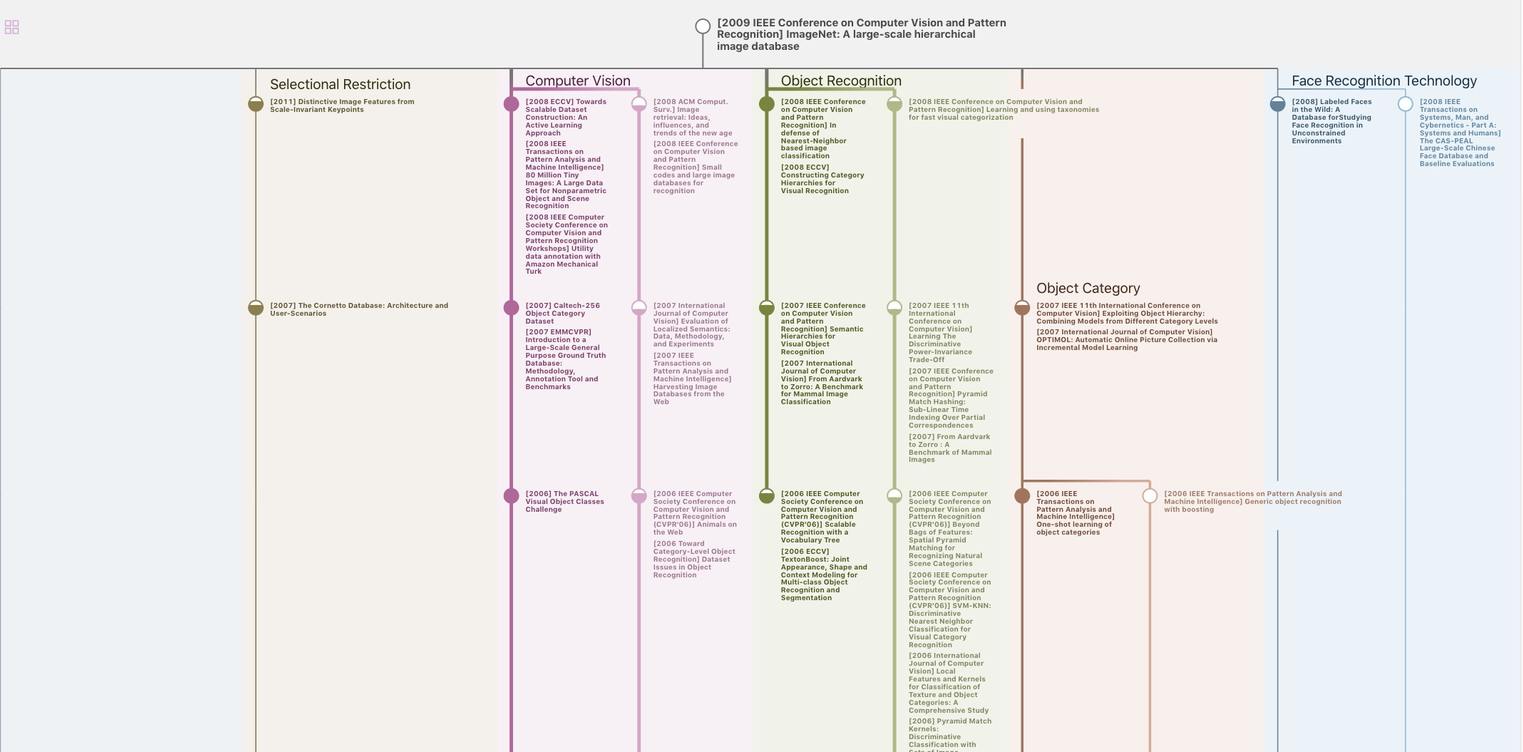
生成溯源树,研究论文发展脉络
Chat Paper
正在生成论文摘要