Classification of hepatic echinococcosis based on ultrasound using radiomics approaches including machine and deep learning models and Compared with primary medical workers: a retrospective, large-scale, dual-center application study
crossref(2024)
摘要
Abstract Background Hepatic echinococcosis (HE) is a life-threatening parasitic disease that requires precise diagnosis and proper treatment, and ultrasonography is recommended to diagnosis. However, challenges remain with primary medical workers in the diagnosis and differentiation of HE by ultrasound. This study aims to develop an intelligent artificial-intelligence-assisted model, based on ultrasound and radiomics flow, to differentiate different types of HE. Methods Between 2005 and 2023, this study employed ultrasonography-based radiomics approaches to build machine learning (ML) and deep learning (DL) models for the classification of HE. ML models, based on eight classifiers, including support vector machine, auto-encoder (AE), linear discriminant analysis, random forest (RF), logistic regression, adaptive boosting, decision tree, and naive Bayes, and DL models, based on EfficientNetB0, InceptionV3, ResNet50 and VGG19, were trained and tested. Sonographers with various diagnostic experience and from different endemic areas were recruited. The diagnostic performance of models was evaluated using receiver operating characteristic (ROC) curve and was compared with primary medical workers using Delong test. Results A total of 5,036 patients and 23,512 images were included. VGG19 network architecture outperformed all other models (P<.001 for all), with areas under the curve (AUCs) of 0.912 (95%CI 0.903-0.920), 0.907 (95%CI 0.898-0.916) and 0.974 (95%CI 0.942-1.000) in the validation, internal test, and prospective test set, respectively. ML models also showed comparable diagnostic efficacy, with AUC of 0.904 (95%CI 0.881-0.988) for RF model, 0.932 (95%CI 0.891-0.993) for AE model, in the prospective test set. Compared to sonographers in the senior high-endemicity area group (AUC 0.886, [95%CI 0.801-0.951]), VGG19 exhibited higher diagnostic performance (P<.001), which outperformed all groups, while random forest (P=.752) and auto-encoder (P=.380) models demonstrated comparable diagnostic efficacy, which outperformed all other groups. Conclusions The application of artificial-intelligence-assisted system could improve the ultrasonic diagnostic ability of primary medical workers for the classification of HE in high-endemicity areas.
更多查看译文
AI 理解论文
溯源树
样例
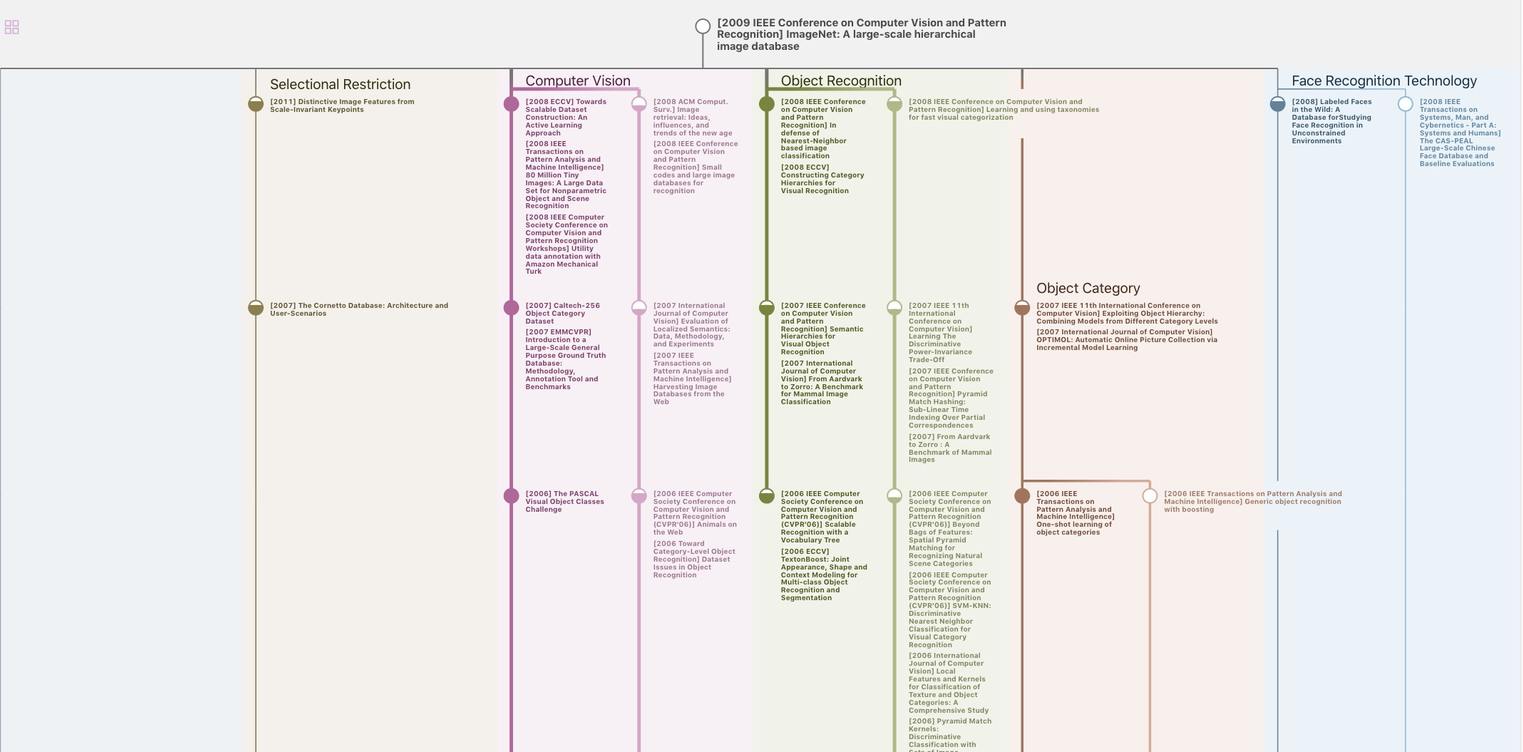
生成溯源树,研究论文发展脉络
Chat Paper
正在生成论文摘要