Tracing the Impact of Bias in Link Prediction
39TH ANNUAL ACM SYMPOSIUM ON APPLIED COMPUTING, SAC 2024(2024)
摘要
Link prediction (LP) in knowledge graphs (KGs) uses embedding-based approaches and machine learning (ML) models to uncover new facts. In order to not overstate and accurately assess the performance of these techniques, their comprehensive and rigorous evaluation is needed. In this work, we suggest a framework to systematically trace and analyze bias---specifically, test leakage bias and sample selection bias---in training and testing knowledge graphs (KGs). The goal is to evaluate how bias affects the performance of LP models We specify a collection of bias measures in SPARQL (the W3C standard query language) to facilitate the analysis of any RDF graph with regard to its structural bias properties. Further, we evaluate our framework over seven state-of-the-art LP datasets (e.g., FB15k-237, WN18RR, and YAGO3-10) and the TransE model. Our findings show how bias, i.e., overrepresentation of entities and relations and pronounced information redundancy, is present across all datasets and how it advantageously impacts the reported performance of the LP model. With these results, we make a call for thorough assessments of data sources in order to discourage the use of biased datasets when appropriate, and to also help improve our understanding of how LP models work and to better interpret their produced output.
更多查看译文
关键词
Link Prediction,Bias,Knowledge Graphs
AI 理解论文
溯源树
样例
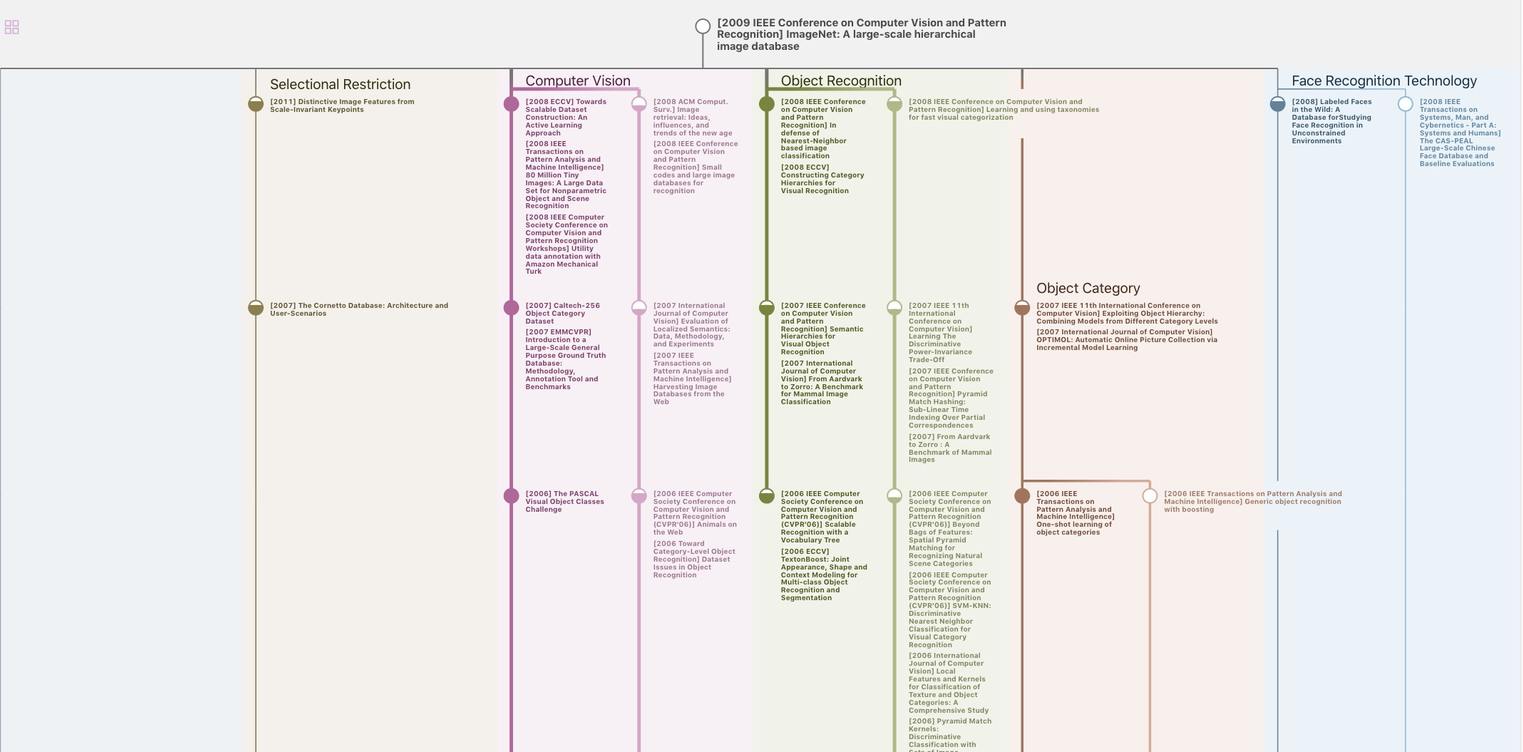
生成溯源树,研究论文发展脉络
Chat Paper
正在生成论文摘要