Continuous and Unconstrained Tremor Monitoring in Parkinson's Disease Using Supervised Machine Learning and Wearable Sensors.
Parkinson's disease(2024)
摘要
Background:Accurately assessing the severity and frequency of fluctuating motor symptoms is important at all stages of Parkinson's disease management. Contrarily to time-consuming clinical testing or patient self-reporting with uncertain reliability, recordings with wearable sensors show promise as a tool for continuously and objectively assessing PD symptoms. While wearables-based clinical assessments during standardised and scripted tasks have been successfully implemented, assessments during unconstrained activity remain a challenge.
Methods:We developed and implemented a supervised machine learning algorithm, trained and tested on tremor scores. We evaluated the algorithm on a 67-hour database comprising sensor data and clinical tremor scores for 24 Parkinson patients at four extremities for periods of about 3 hours. A random 25% subset of the labelled samples was used as test data, the remainder as training data. Based on features extracted from the sensor data, a Support Vector Machine was trained to predict tremor severity. Due to the inherent imbalance in tremor scores, we applied dataset rebalancing techniques.
Results:Our classifier demonstrated robust performance in detecting tremor events with a sensitivity of 0.90 on the test-portion of the resampled dataset. The overall classification accuracy was high at 0.88.
Conclusion:We implemented an accurate classifier for tremor monitoring in free-living environments that can be trained even with modestly sized and imbalanced datasets. This advancement offers significant clinical value in continuously monitoring Parkinson's disease symptoms beyond the hospital setting, paving the way for personalized management of PD, timely therapeutic adjustments, and improved patient quality of life.
更多查看译文
AI 理解论文
溯源树
样例
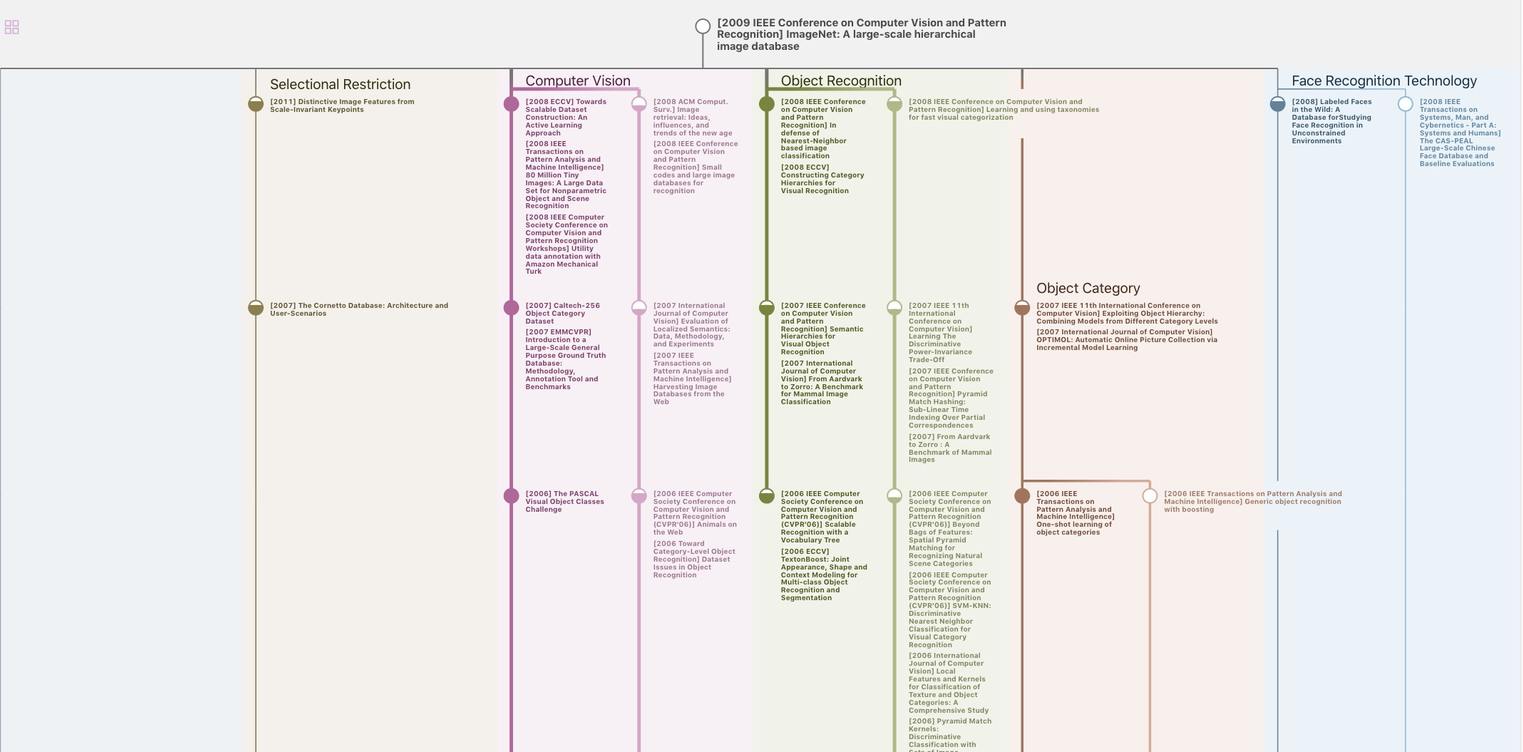
生成溯源树,研究论文发展脉络
Chat Paper
正在生成论文摘要