Improved Brain Metastases Segmentation Using Generative Adversarial Network and Conditional Random Field Optimization Mask R‐CNN
MEDICAL PHYSICS(2024)
摘要
BACKGROUND:In radiotherapy, the delineation of the gross tumor volume (GTV) in brain metastases using computed tomography (CT) simulation localization is very important. However, despite the criticality of this process, a pronounced gap exists in the availability of tools tailored for the automatic segmentation of the GTV based on CT simulation localization images.PURPOSE:This study aims to fill this gap by devising an effective tool specifically for the automatic segmentation of the GTV using CT simulation localization images.METHODS:A dual-network generative adversarial network (GAN) architecture was developed, wherein the generator focused on refining CT images for more precise delineation, and the discriminator differentiated between real and augmented images. This architecture was coupled with the Mask R-CNN model to achieve meticulous GTV segmentation. An end-to-end training process facilitated the integration between the GAN and Mask R-CNN functionalities. Furthermore, a conditional random field (CRF) was incorporated to refine the initial masks generated by the Mask R-CNN model to ensure optimal segmentation accuracy. The performance was assessed using key metrics, namely, the Dice coefficient (DSC), intersection over union (IoU), accuracy, specificity, and sensitivity.RESULTS:The GAN+Mask R-CNN+CRF integration method in this study performs well in GTV segmentation. In particular, the model has an overall average DSC of 0.819 ± 0.102 and an IoU of 0.712 ± 0.111 in the internal validation. The overall average DSC in the external validation data is 0.726 ± 0.128 and the IoU is 0.640 ± 0.136. It demonstrates favorable generalization ability.CONCLUSION:The integration of the GAN, Mask R-CNN, and CRF optimization provides a pioneering tool for the sophisticated segmentation of the GTV in brain metastases using CT simulation localization images. The method proposed in this study can provide a robust automatic segmentation approach for brain metastases in the absence of MRI.
更多查看译文
关键词
artificial intelligence,automatic segmentation,brain metastases,convolutional neural network,deep learning,gross tumor volume
AI 理解论文
溯源树
样例
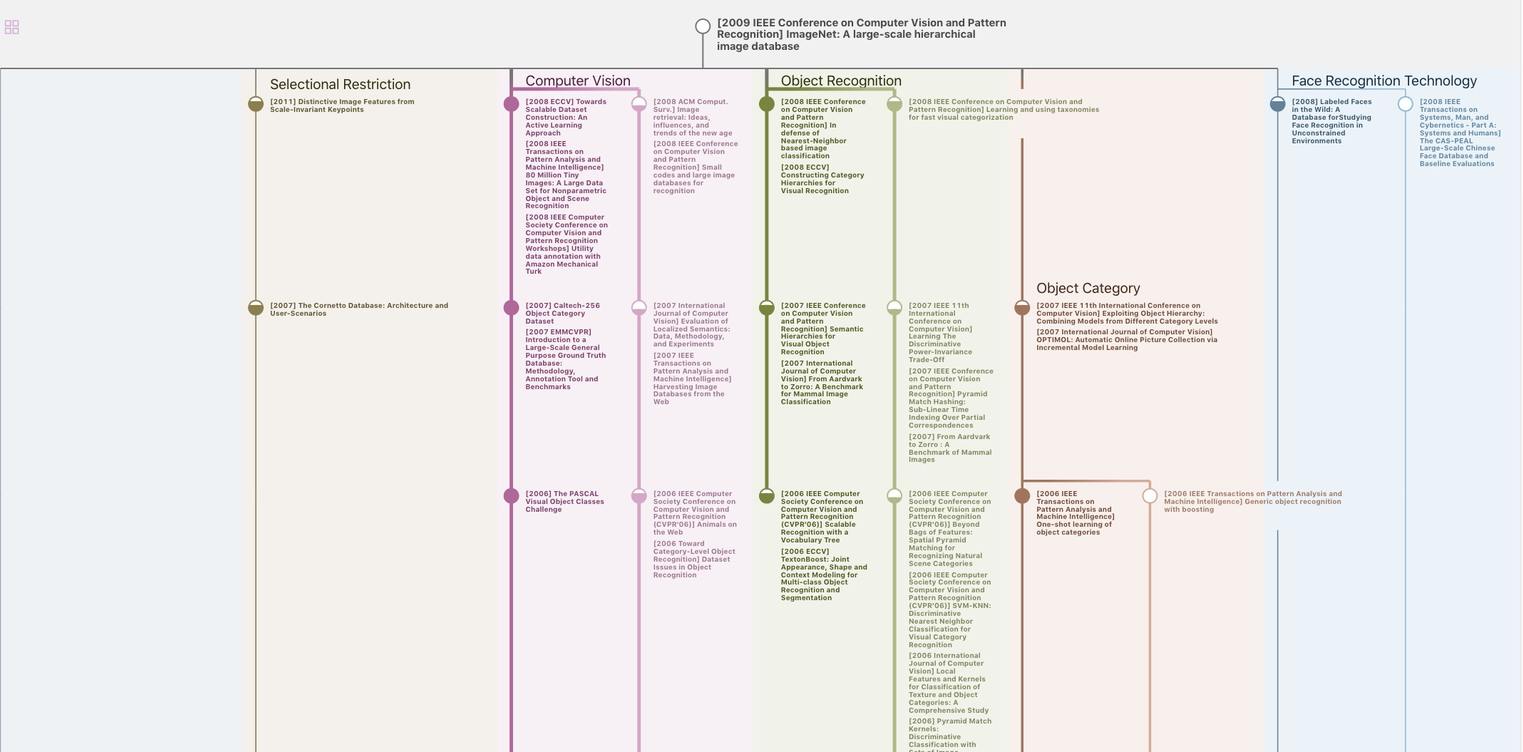
生成溯源树,研究论文发展脉络
Chat Paper
正在生成论文摘要