Standalone Deep Learning Versus Experts for Diagnosis Lung Cancer on Chest Computed Tomography: a Systematic Review
European Radiology(2024)
摘要
PURPOSE:To compare the diagnostic performance of standalone deep learning (DL) algorithms and human experts in lung cancer detection on chest computed tomography (CT) scans.MATERIALS AND METHODS:This study searched for studies on PubMed, Embase, and Web of Science from their inception until November 2023. We focused on adult lung cancer patients and compared the efficacy of DL algorithms and expert radiologists in disease diagnosis on CT scans. Quality assessment was performed using QUADAS-2, QUADAS-C, and CLAIM. Bivariate random-effects and subgroup analyses were performed for tasks (malignancy classification vs invasiveness classification), imaging modalities (CT vs low-dose CT [LDCT] vs high-resolution CT), study region, software used, and publication year.RESULTS:We included 20 studies on various aspects of lung cancer diagnosis on CT scans. Quantitatively, DL algorithms exhibited superior sensitivity (82%) and specificity (75%) compared to human experts (sensitivity 81%, specificity 69%). However, the difference in specificity was statistically significant, whereas the difference in sensitivity was not statistically significant. The DL algorithms' performance varied across different imaging modalities and tasks, demonstrating the need for tailored optimization of DL algorithms. Notably, DL algorithms matched experts in sensitivity on standard CT, surpassing them in specificity, but showed higher sensitivity with lower specificity on LDCT scans.CONCLUSION:DL algorithms demonstrated improved accuracy over human readers in malignancy and invasiveness classification on CT scans. However, their performance varies by imaging modality, underlining the importance of continued research to fully assess DL algorithms' diagnostic effectiveness in lung cancer.CLINICAL RELEVANCE STATEMENT:DL algorithms have the potential to refine lung cancer diagnosis on CT, matching human sensitivity and surpassing in specificity. These findings call for further DL optimization across imaging modalities, aiming to advance clinical diagnostics and patient outcomes.KEY POINTS:Lung cancer diagnosis by CT is challenging and can be improved with AI integration. DL shows higher accuracy in lung cancer detection on CT than human experts. Enhanced DL accuracy could lead to improved lung cancer diagnosis and outcomes.
更多查看译文
关键词
Deep learning,Lung neoplasms,Computed tomography (CT),Meta-analysis,Comparative study
AI 理解论文
溯源树
样例
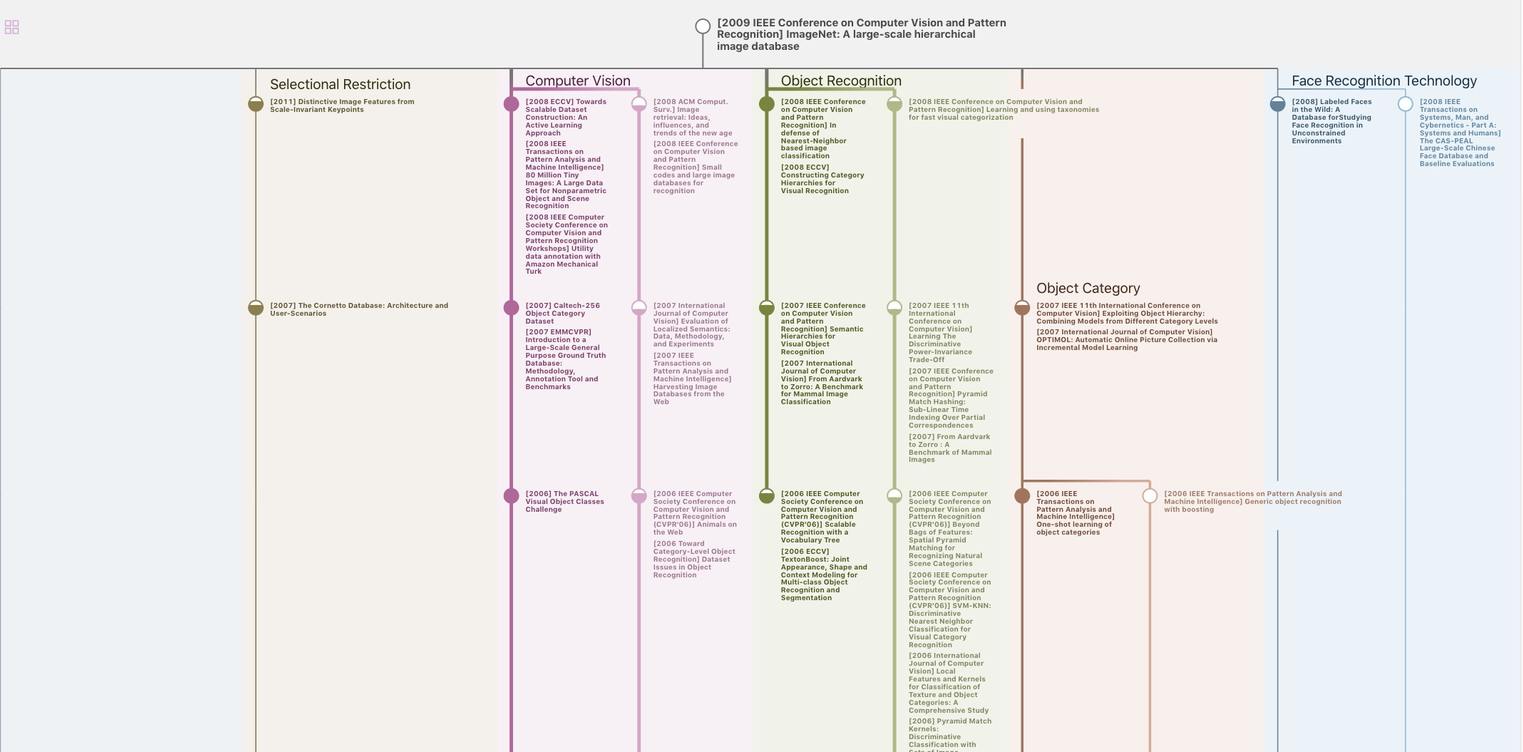
生成溯源树,研究论文发展脉络
Chat Paper
正在生成论文摘要