PDF Malware Detection: A Hybrid Approach Using Random Forest and K-Nearest Neighbors.
ICCR(2024)
摘要
The rise of Portable Document Format (PDF) malware poses a significant threat to organizations and individuals. Traditional signature-based detection methods are often ineffective against new and emerging malware strains, while machine learning-based detection approaches have the potential to be more effective. This paper proposes a hybrid detection approach for PDF-based malware based on machine learning integrating the Random Forest (RF) and K-Nearest Neighbors (KNN) algorithms called the hybrid RF-KNN PDF malware detection approach. By combining the strengths of both algorithms, our approach aims to enhance accuracy in identifying and preventing PDF malware attacks. Through an empirical assessment of the Evasive-PDFMal2022 dataset, our hybrid approach demonstrates remarkable effectiveness with an accuracy rate of 99.2%. This surpasses the performance of existing models in the field, establishing the RF-KNN PDF malware detection approach as a promising and high-accuracy tool for tackling PDF malware threats. The proposed hybrid approach highlights the potential of machine learning in strengthening cybersecurity efforts against emerging threats.
更多查看译文
关键词
portable document format (pdf),machine learning,detection,random forest,k-nearest neighbors,pdf malware,evasion attacks,malicious pdf detection,cybersecurity
AI 理解论文
溯源树
样例
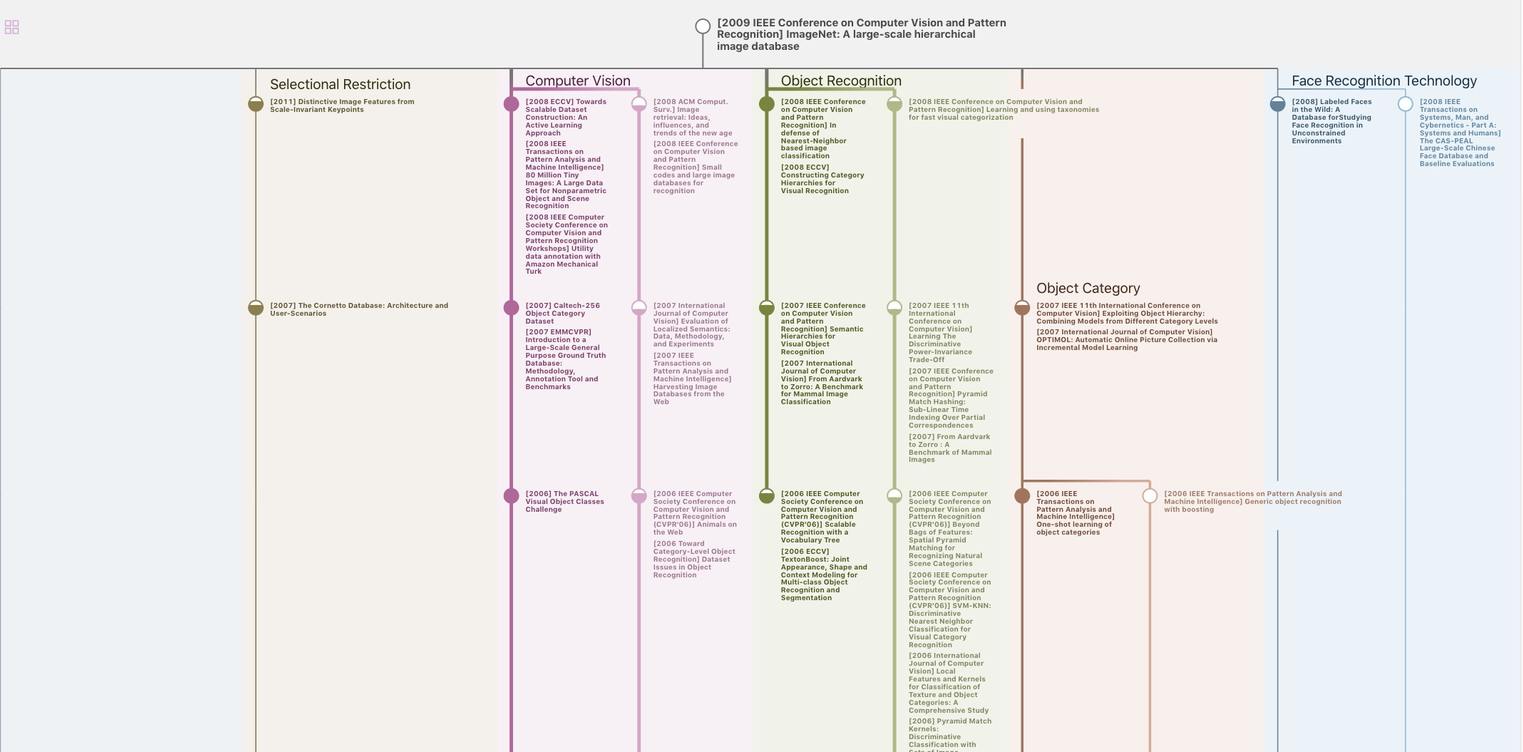
生成溯源树,研究论文发展脉络
Chat Paper
正在生成论文摘要