Research on Hyperspectral Image Classification Based on Improved Deep Cross-Domain Few-Shot Learning
VSIP '23 Proceedings of the 2023 5th International Conference on Video, Signal and Image Processing(2024)
摘要
In this study, an enhanced method and system for hyperspectral image classification are presented, based on deep cross-scene few-shot learning. This pertains to the domain of remote sensing image processing technology and addresses the prevalent issues of inadequate classification performance in existing techniques for hyperspectral image categorization. The core aspects of this invention encompass the following: utilization of two mapping layers to standardize the input dimensions between the source and target domains; the deployment of an embedded feature extractor to incorporate the image cubes from both the source and target domains into a space-spectral embedding environment simultaneously, ensuring that like samples are closely aligned and dissimilar ones are distanced. Through gauging the distances between each class of unlabeled and labeled samples in this space-spectral embedding zone, learning with a few number of examples in both the source and target domains is achieved. Furthermore, a conditional domain discriminator is employed to mitigate domain shifts between domains, thus solidifying the domain stability of the extracted spatial-spectral embedding features. This innovative approach allows for high-precision hyperspectral data categorization, even when only a few examples are available.
更多查看译文
AI 理解论文
溯源树
样例
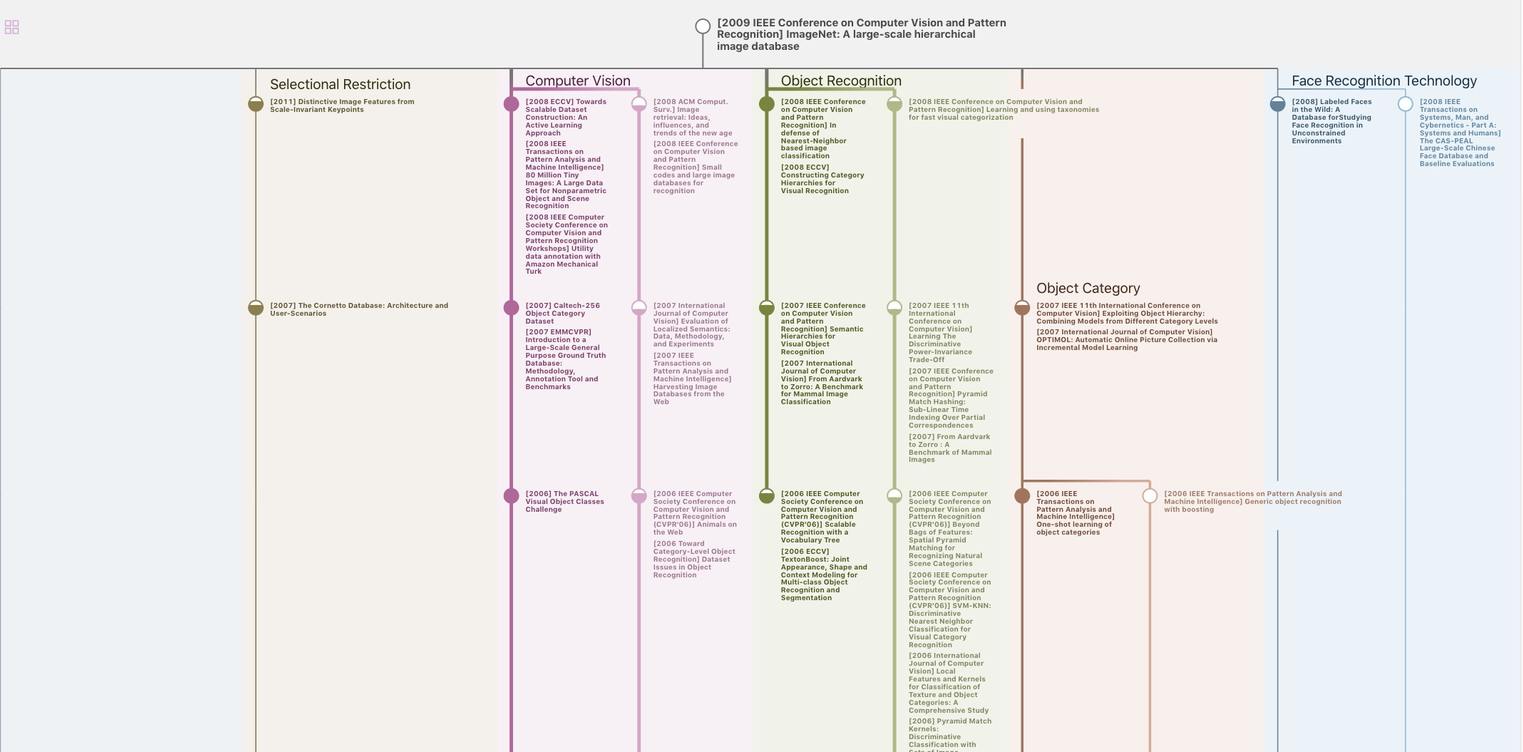
生成溯源树,研究论文发展脉络
Chat Paper
正在生成论文摘要