A Self-supervised Learning-Based Fine-Grained Classification Model for Distinguishing Malignant From Benign Subcentimeter Solid Pulmonary Nodules
Academic Radiology(2024)
摘要
Rationale and Objectives
Diagnosing subcentimeter solid pulmonary nodules (SSPNs) remains challenging in clinical practice. Deep learning may perform better than conventional methods in differentiating benign and malignant pulmonary nodules. This study aimed to develop and validate a model for differentiating malignant and benign SSPNs using CT images.
Materials and Methods
This retrospective study included consecutive patients with SSPNs detected between January 2015 and October 2021 as an internal dataset. Malignancy was confirmed pathologically; benignity was confirmed pathologically or via follow-up evaluations. The SSPNs were segmented manually. A self-supervision pre-training-based fine-grained network was developed for predicting SSPN malignancy. The pre-trained model was established using data from the National Lung Screening Trial, Lung Nodule Analysis 2016, and a database of 5478 pulmonary nodules from the previous study, with subsequent fine-tuning using the internal dataset. The model’s efficacy was investigated using an external cohort from another center, and its accuracy, sensitivity, specificity, and area under the receiver operating characteristic curve (AUC) were determined.
Results
Overall, 1276 patients (mean age, 56 ± 10 years; 497 males) with 1389 SSPNs (mean diameter, 7.5 ± 2.0 mm; 625 benign) were enrolled. The internal dataset was specifically enriched for malignancy. The model’s performance in the internal testing set (316 SSPNs) was: AUC, 0.964 (95% confidence interval (95%CI): 0.942–0.986); accuracy, 0.934; sensitivity, 0.965; and specificity, 0.908. The model’s performance in the external test set (202 SSPNs) was: AUC, 0.945 (95% CI: 0.910–0.979); accuracy, 0.911; sensitivity, 0.977; and specificity, 0.860.
Conclusion
This deep learning model was robust and exhibited good performance in predicting the malignancy of SSPNs, which could help optimize patient management.
更多查看译文
关键词
Solitary pulmonary nodule,Artificial intelligence,Deep learning,Tomography,X-Ray computed,Diagnosis,differential
AI 理解论文
溯源树
样例
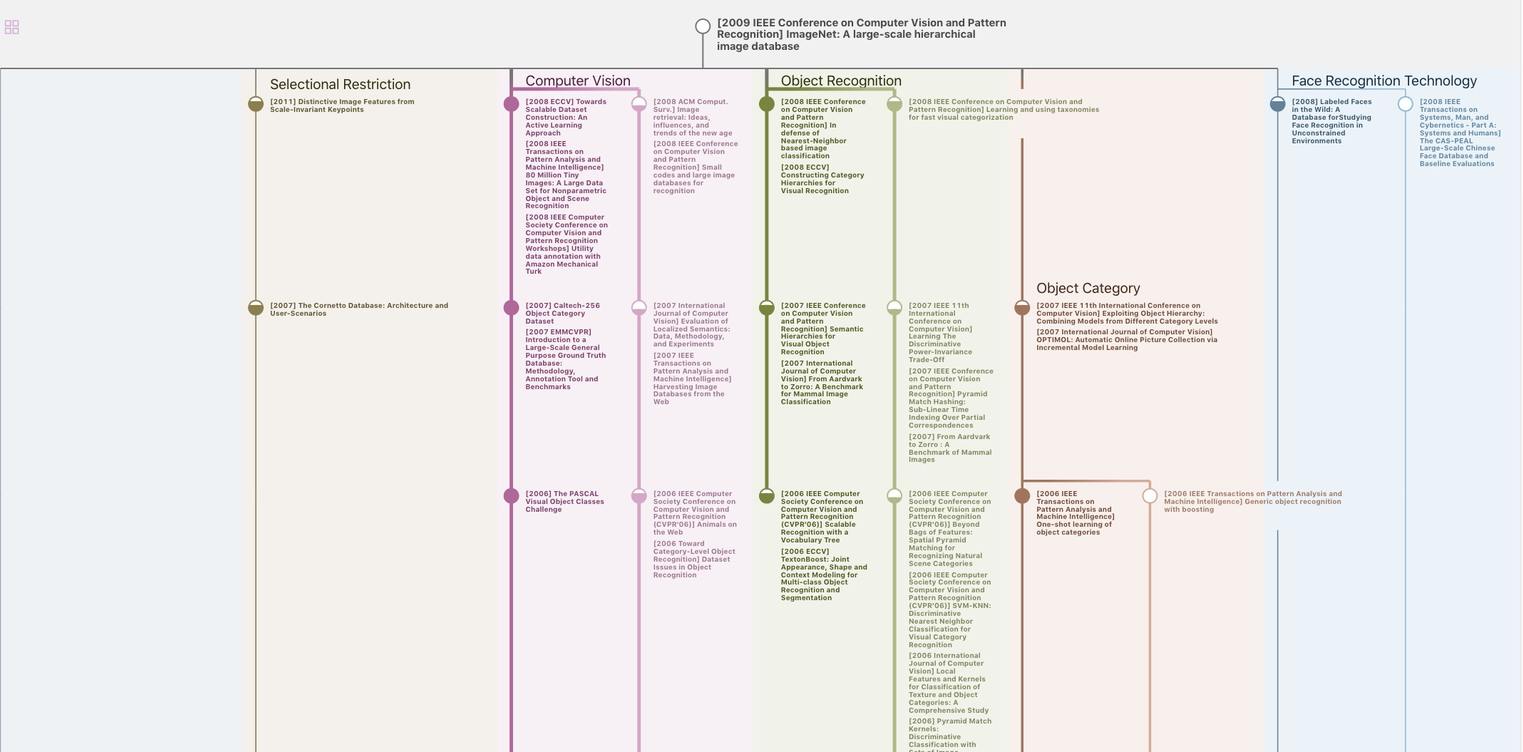
生成溯源树,研究论文发展脉络
Chat Paper
正在生成论文摘要