Combined Classification of Hyperspectral and LiDAR Data Based on Dual-Channel Cross-Transformer.
VSIP '23 Proceedings of the 2023 5th International Conference on Video, Signal and Image Processing(2024)
摘要
In the face of complex scenes, single-modal dominant classification tasks encounter limitations in performance due to insufficient information. On the other hand, joint classification of multimodal remote sensing data faces challenges such as data sample differences and lack of correlation in physical features between modalities, which can impact classification performance. To fully integrate the heterogeneous information of multimodal data and improve classification performance, we propose a dual-channel cross-transformer feature fusion extraction network. The framework leverages self-attention mechanisms to aggregate features within each modality, and the feature fusion module based on cross-modal attention fully considers the complementary information between modalities. Classification tasks are performed using the fused spatial-spectral features obtained from the joint representation of modalities. Extensive experiments conducted on the Houston and MUUFL datasets demonstrate the effectiveness of the proposed model compared to existing methods.
更多查看译文
AI 理解论文
溯源树
样例
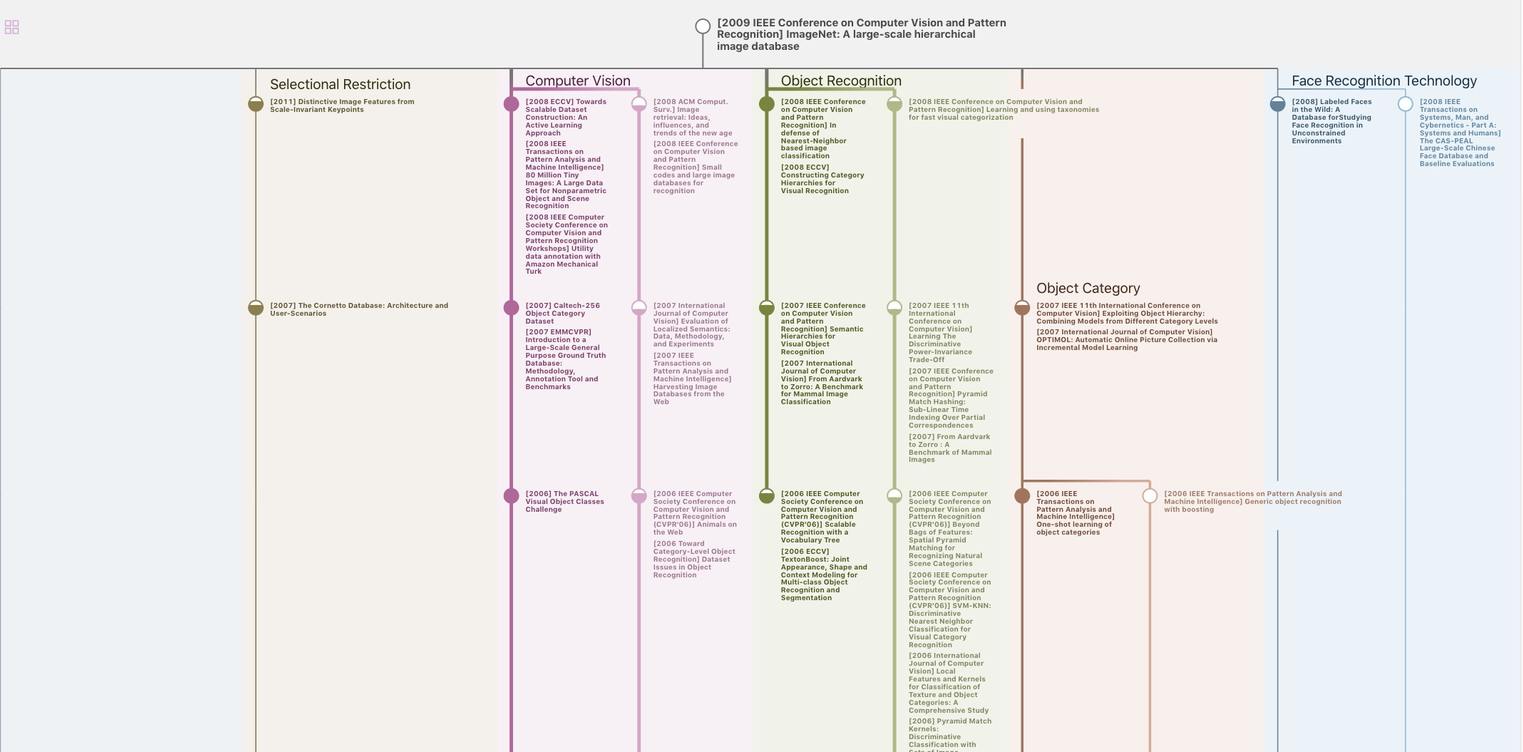
生成溯源树,研究论文发展脉络
Chat Paper
正在生成论文摘要