Multitask Learning-Based Affective Prediction for Videos of Films and TV Scenes
Applied sciences(2024)
摘要
Film and TV video scenes contain rich art and design elements such as light and shadow, color, composition, and complex affects. To recognize the fine-grained affects of the art carrier, this paper proposes a multitask affective value prediction model based on an attention mechanism. After comparing the characteristics of different models, a multitask prediction framework based on the improved progressive layered extraction (PLE) architecture (multi-headed attention and factor correlation-based PLE), incorporating a multi-headed self-attention mechanism and correlation analysis of affective factors, is constructed. Both the dynamic and static features of a video are chosen as fusion input, while the regression of fine-grained affects and classification of whether a character exists in a video are designed as different training tasks. Considering the correlation between different affects, we propose a loss function based on association constraints, which effectively solves the problem of training balance within tasks. Experimental results on a self-built video dataset show that the algorithm can give full play to the complementary advantages of different features and improve the accuracy of prediction, which is more suitable for fine-grained affect mining of film and TV scenes.
更多查看译文
关键词
film and TV scenes,multitask learning,fine-grained affects,affective value prediction
AI 理解论文
溯源树
样例
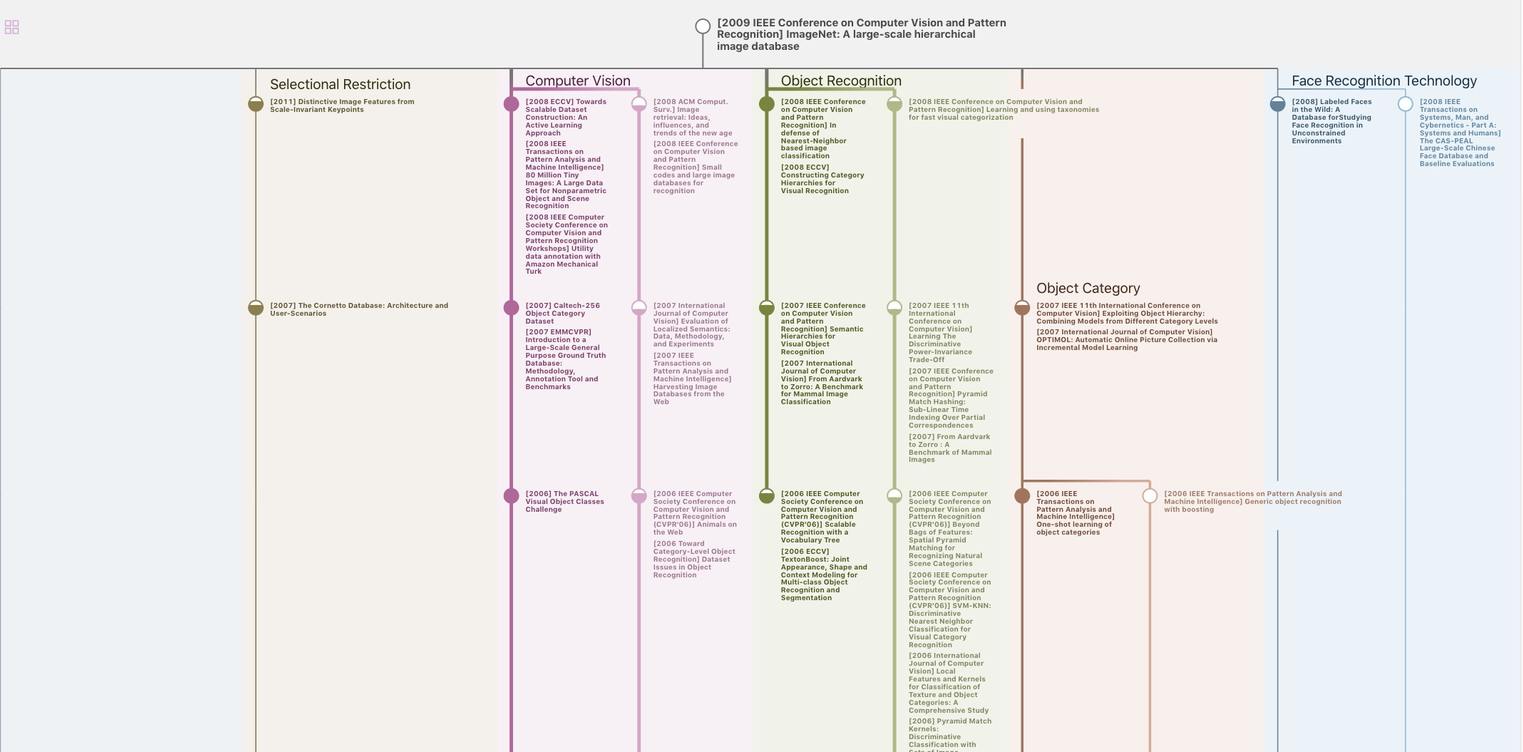
生成溯源树,研究论文发展脉络
Chat Paper
正在生成论文摘要