Evaluating the Accuracy of Machine Learning Classification Models for Similar Herbal Medicine Using Hyperspectral Imaging
Journal of Biosystems Engineering(2024)
摘要
Processed herbal medicines in East Asia are often misused or mixed because distinguishing their different forms is difficult using the naked eye. However, an artificial intelligence model can address this problem by classifying herbal medicines with similar shapes. This study proposes using hyperspectral images to classify herbal medicines from five plant species belonging to the Apiaceae family: Angelica Gigas, Angelica acutiloba, Saposhnikovia divaricata, Peucedanum japonicum, and Glehnia littoralis. To classify the hyperspectral images, we evaluated the performance using six machine learning-based models—SVM, KNN, DT, RF, LR, and LightGBM; preprocessed the original data with a Savitzky-Golay filter, first derivative, and second derivative; and evaluated processing changes in accuracy. The logistic regression model with the second derivative showed the best performance, with an accuracy of 0.99. The classification prediction error was due to the very high similarity between the second derivative data of the Peucedani Japonici Radix and Glehnia littoralis. The above results confirmed that the second derivative during preprocessing and logistic regression model performed best when analyzing the hyperspectral images. Hyperspectral data is proposed for the hyperspectral image analysis of herbal medicines that use the root zone as a medicinal site, owing to its excellent performance.
更多查看译文
关键词
Root medicine,Phenotyping,Apiaceae,Data preprocessing,Logistic regression
AI 理解论文
溯源树
样例
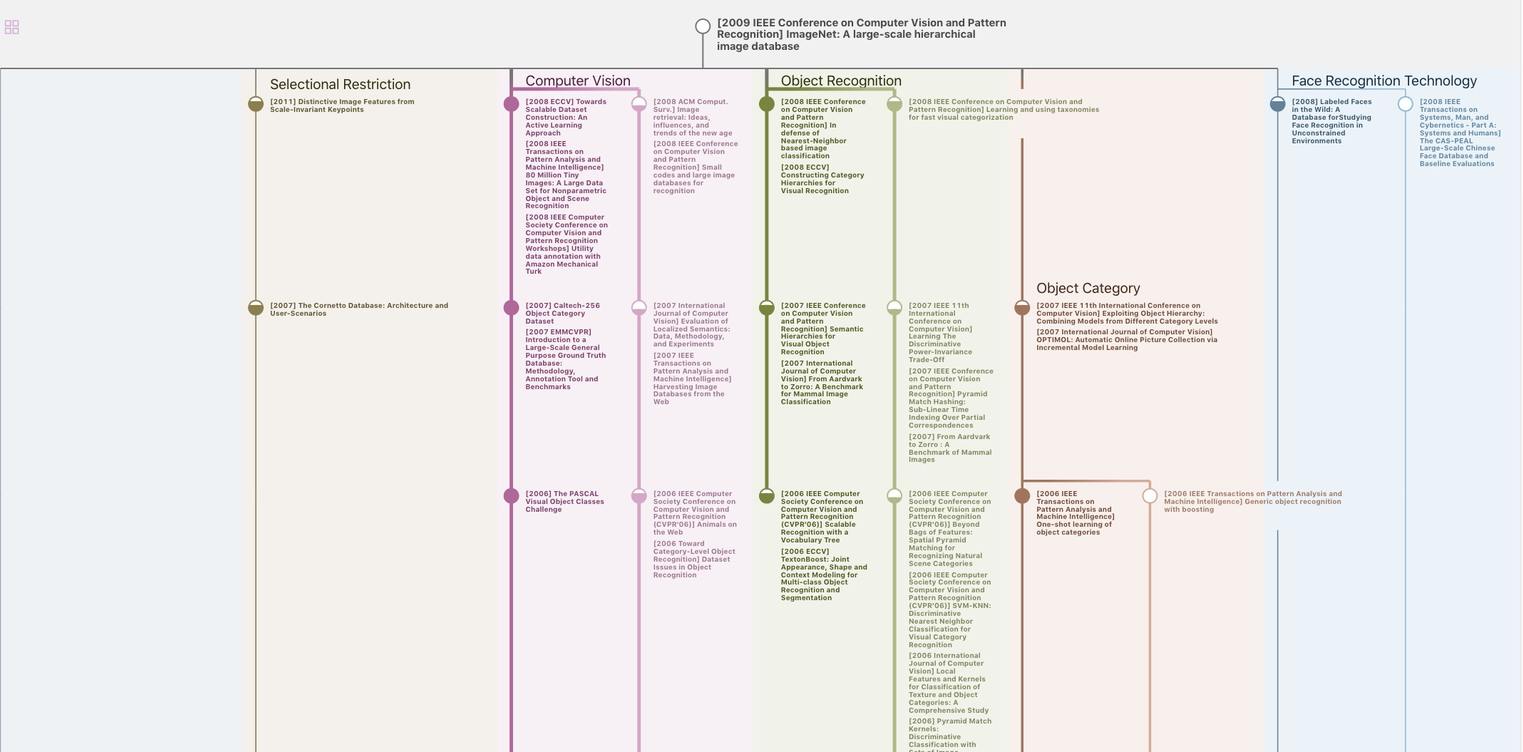
生成溯源树,研究论文发展脉络
Chat Paper
正在生成论文摘要