Measuring the Burgers Vector of Dislocations with Dark-Field X-ray Microscopy
arXiv · Materials Science(2024)
Abstract
The behavior of dislocations is essential to understand material properties,
but their subsurface dynamics that are representative of bulk phenomena cannot
be resolved by conventional transmission electron microscopy (TEM). Dark field
X-ray microscope (DFXM) was recently demonstrated to image hierarchical
structures of bulk dislocations by imaging lattice distortions along the
transmitted X-ray diffracted beam using an objective lens. While today's DFXM
can effectively map the line vector of dislocations, it still cannot quantify
the Burgers vector required to understand dislocation interactions, structures,
and energies. Our study formulates a theoretical model of how DFXM images
collected along specific scans can be used to directly measure the Burgers
vector of a dislocation. By revisiting the "invisibility criteria" from TEM
theory, we re-solve this formalism for DFXM and extend it to the
geometric-optics model developed for DFXM to evaluate how the images acquired
from different scans about a single hkl diffraction peak encode the Burgers
vector within them. We demonstrate this for edge, screw, and mixed dislocations
and discuss the observed symmetries. This work advances our understanding of
DFXM to establish its capabilities to connect bulk experiments to dislocation
theory and mechanics.
MoreTranslated text
PDF
View via Publisher
AI Read Science
Must-Reading Tree
Example
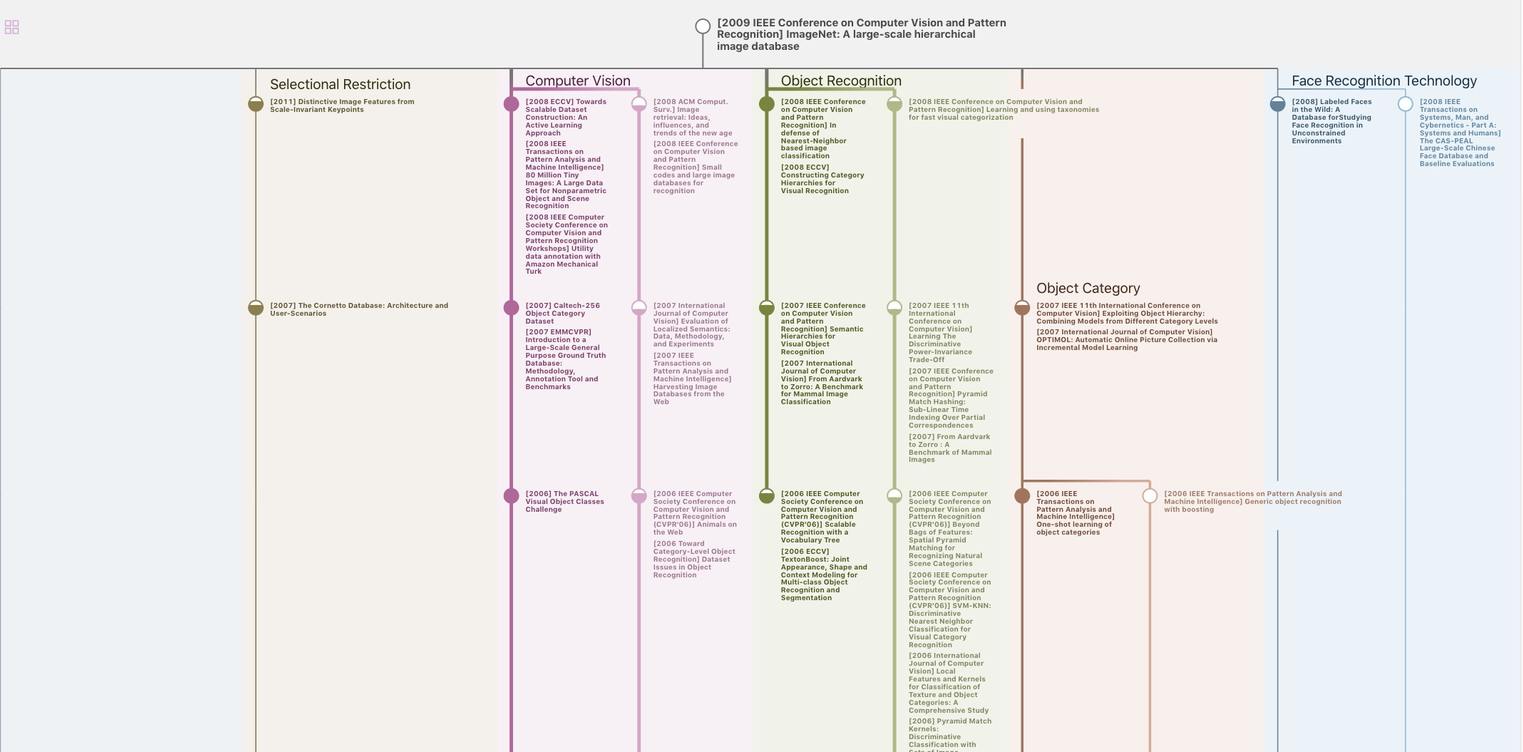
Generate MRT to find the research sequence of this paper
Related Papers
Data Disclaimer
The page data are from open Internet sources, cooperative publishers and automatic analysis results through AI technology. We do not make any commitments and guarantees for the validity, accuracy, correctness, reliability, completeness and timeliness of the page data. If you have any questions, please contact us by email: report@aminer.cn
Chat Paper
去 AI 文献库 对话