Mitigating Measurement Inaccuracies in Digital Twins of Construction Machinery Through Multi-Objective Optimization
SENSORS(2024)
摘要
The advent of digital twins facilitates the generation of high-fidelity replicas of actual systems or assets, thereby enhancing the design’s performance and feasibility. When developing digital twins, precise measurement data is essential to ensure alignment between the actual and digital models. However, inherent uncertainties in sensors and models lead to disparities between observed and predicted (simulated) behaviors. To mitigate these uncertainties, this study originally proposes a multi-objective optimization strategy utilizing a Gaussian process regression surrogate model, which integrates various uncertain parameters, such as load angle, bucket cylinder stroke, arm cylinder stroke, and boom cylinder stroke. This optimization employs a genetic algorithm to indicate the Pareto frontiers regarding the pressure exerted on the boom, arm, and bucket cylinders. Subsequently, TOPSIS is applied to ascertain the optimal candidate among the identified Pareto optima. The findings reveal a substantial congruence between the experimental and numerical outcomes of the devised virtual model, in conjunction with the TOPSIS-derived optimal parameter configuration.
更多查看译文
关键词
excavator finite element model,surrogate model,multi-objective optimization,Gaussian process regression,TOPSIS,digital twin
AI 理解论文
溯源树
样例
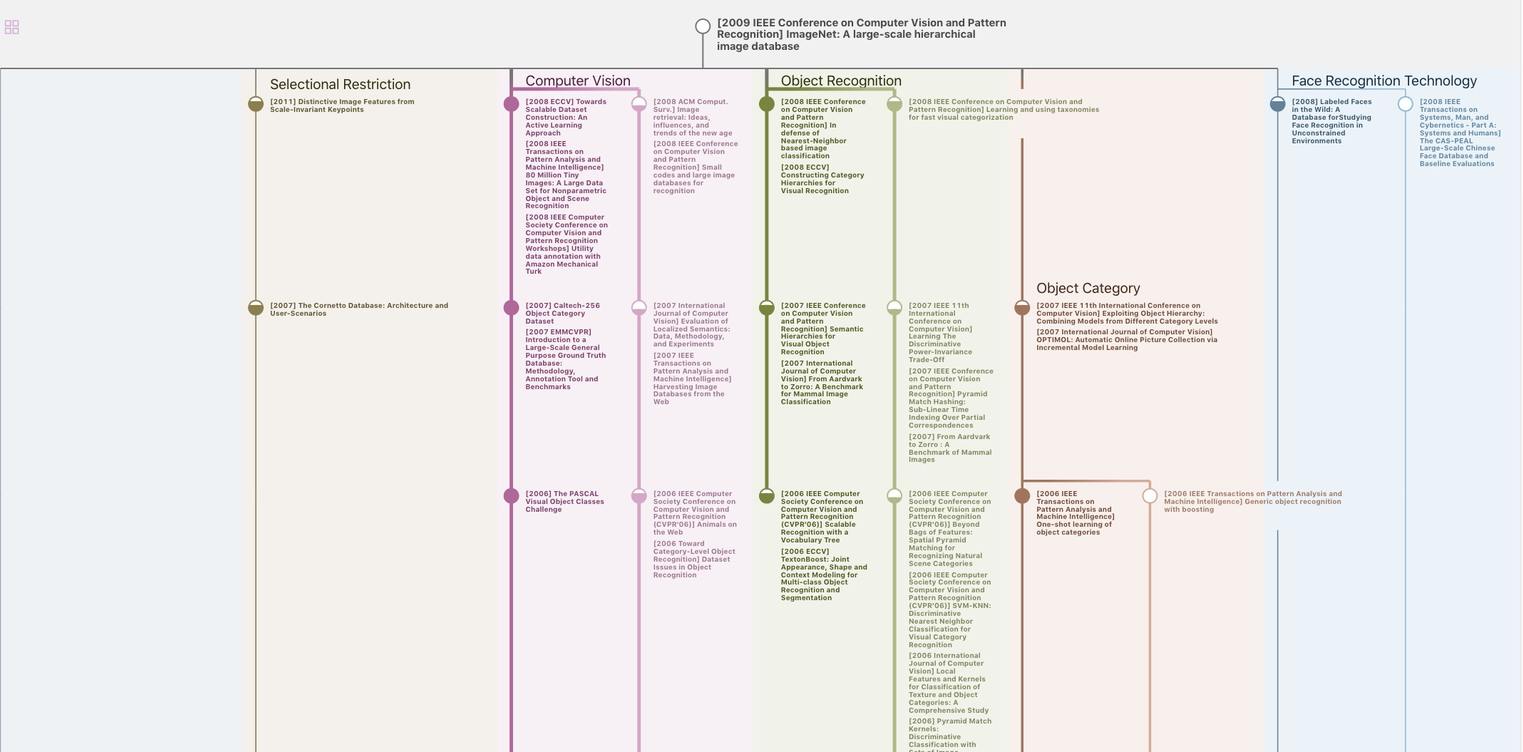
生成溯源树,研究论文发展脉络
Chat Paper
正在生成论文摘要