MuSAM: Mutual-Scenario-Aware Multimodal-Enhanced Representation Learning for Semantic Similarity
IEEE transactions on industrial informatics(2024)
摘要
Word polysemy poses a formidable challenge in the semantic similarity task, especially for complex Chinese semantic information. However, most existing methods tend to emphasize information expansion, often overlooking the fact that the added information may be either irrelevant or only weakly correlated. In view of this, we propose a novel approach that fuses knowledge enhancement and context filtering to achieve self-selective semantic expansion. This approach, termed mutual-scenario-aware multimodal-enhanced representation learning (MuSAM), integrates information across multiple modalities. Specifically, we extend individual words within three modalities, and the extended information with weak correlation is filtered and denoised, to get the mutual-scenario extended information. The extended information with strong correlation in each modality will be fused to obtain the multimodal fusion representation vector of the word pair. Experimental evaluations conducted on five datasets underscore the superiority of the MuSAM model over state-of-the-art methods, showcasing a performance improvement ranging from a minimum of 4% to a maximum of 21%. Remarkably, our model is designed in a completely engineering manner, which can be applied to real scenarios directly without manual intervention.
更多查看译文
关键词
Semantics,Dictionaries,Vectors,Task analysis,Training,Correlation,Representation learning,Multimodal enhanced,mutual scenario,representation learning,semantic similarity
AI 理解论文
溯源树
样例
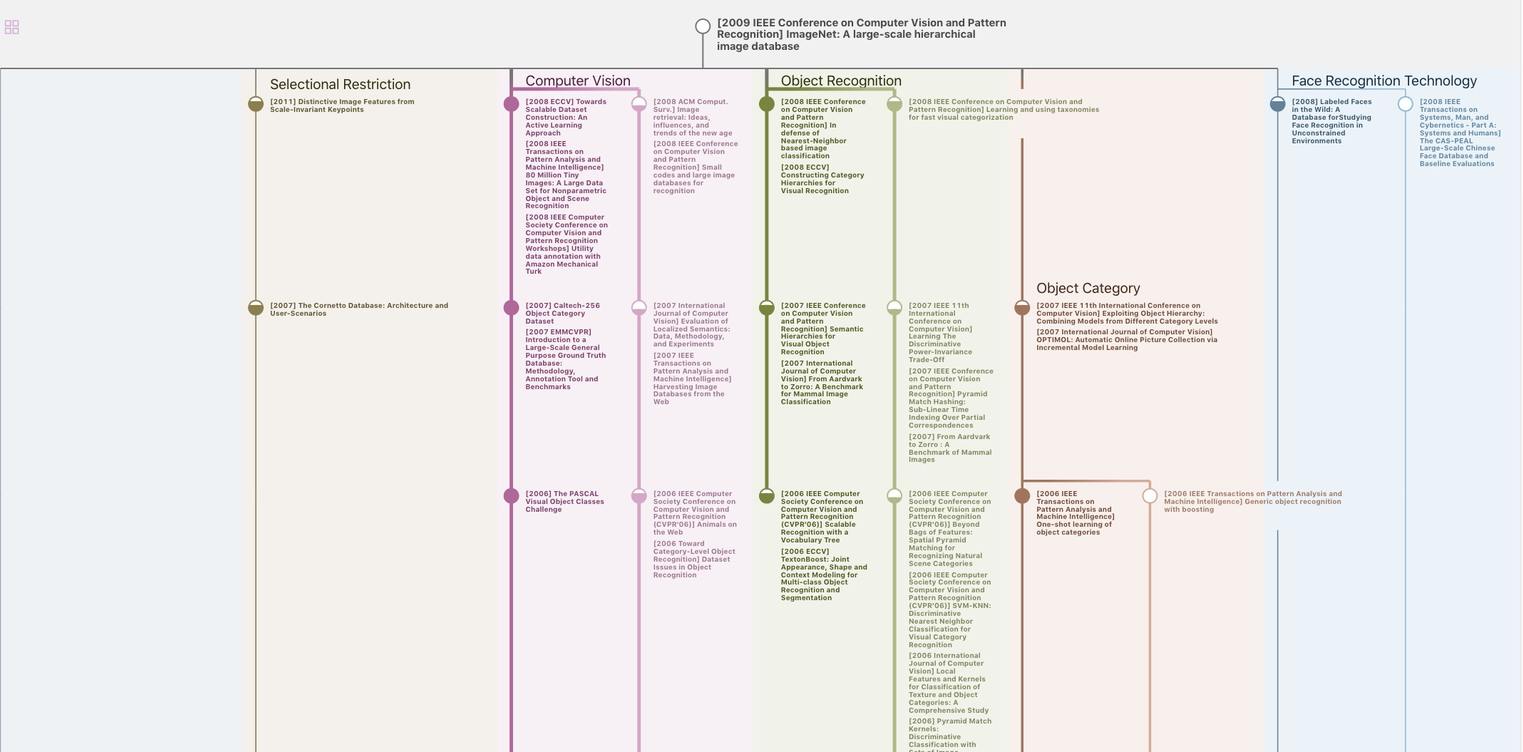
生成溯源树,研究论文发展脉络
Chat Paper
正在生成论文摘要