Layered Fusion Reconstruction Based on Fuzzy Features for Multi-Conductivity Electrical Impedance Tomography.
Sensors (Basel, Switzerland)(2024)
摘要
In medical imaging, detecting tissue anomalies is vital for accurate diagnosis and effective treatment. Electrical impedance tomography (EIT) is a non-invasive technique that monitors the changes in electrical conductivity within tissues in real time. However, the current challenge lies in simply and accurately reconstructing multi-conductivity distributions. This paper introduces a layered fusion framework for EIT to enhance imaging in multi-conductivity scenarios. The method begins with pre-imaging and extracts the main object from the fuzzy image to form one layer. Then, the voltage difference in the other layer, where the local anomaly is located, is estimated. Finally, the corresponding conductivity distribution is established, and multiple layers are fused to reconstruct the multi-conductivity distribution. The simulation and experimental results demonstrate that compared to traditional methods, the proposed method significantly improves multi-conductivity separation, precise anomaly localization, and robustness without adding uncertain parameters. Notably, the proposed method has demonstrated exceptional accuracy in local anomaly detection, with positional errors as low as 1% and size errors as low as 33%, which significantly outperforms the traditional method with respective minimum errors of 9% and 228%. This method ensures a balance between the simplicity and accuracy of the algorithm. At the same time, it breaks the constraints of traditional linear methods, struggling to identify multi-conductivity distributions, thereby providing new perspectives for clinical EIT.
更多查看译文
关键词
layered fusion,electrical impedance tomography (EIT),multi-conductivity distribution,local anomaly detection
AI 理解论文
溯源树
样例
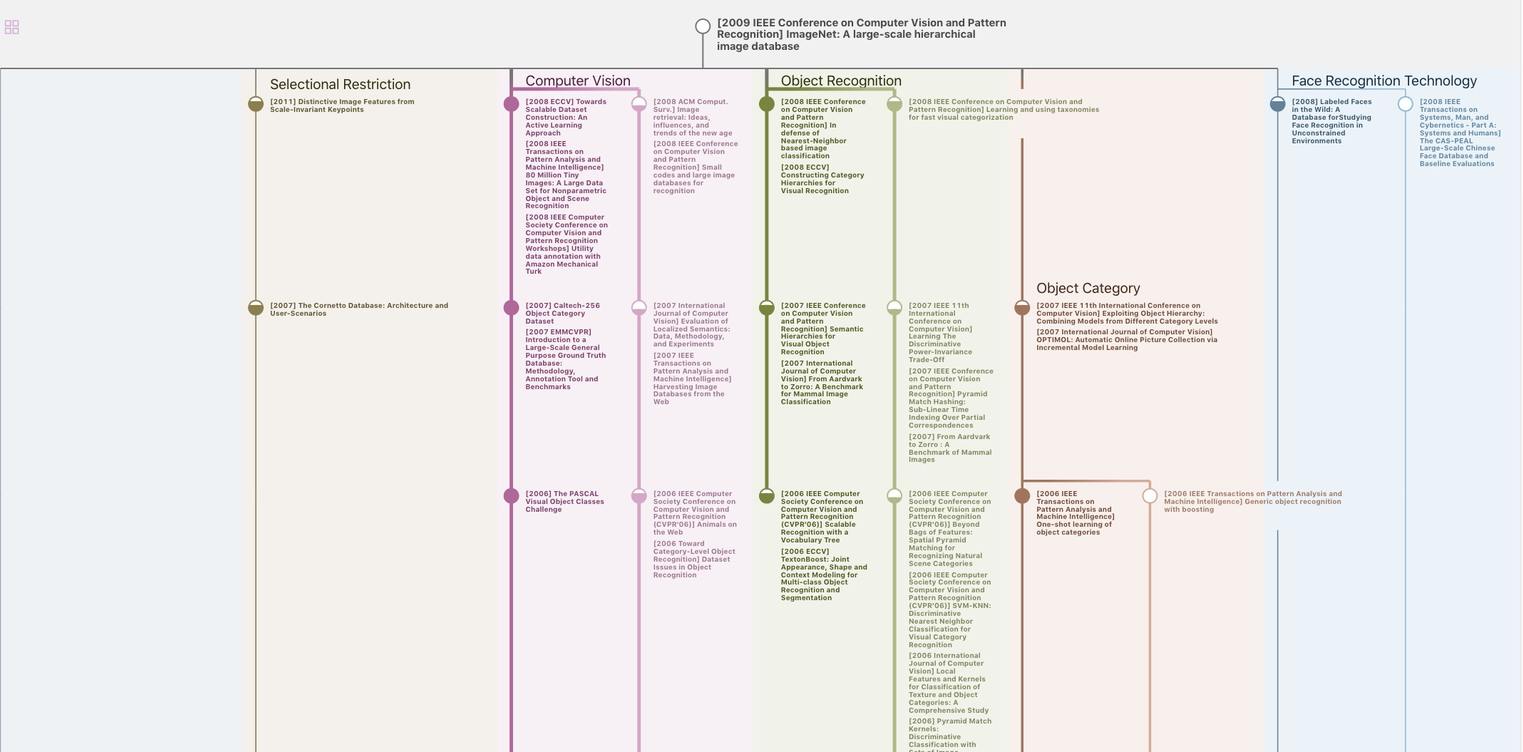
生成溯源树,研究论文发展脉络
Chat Paper
正在生成论文摘要