Biometrics and Behavior Analysis for Detecting Distractions in E-Learning
XXVI INTERNATIONAL SYMPOSIUM ON COMPUTERS IN EDUCATION, SIIE 2024(2024)
Abstract
In this article, we explore computer vision approaches to detect abnormal head pose during e-learning sessions and we introduce a study on the effects of mobile phone usage during these sessions. We utilize behavioral data collected from 120 learners monitored while participating in a MOOC learning sessions. Our study focuses on the influence of phone-usage events on behavior and physiological responses, specifically attention, heart rate, and meditation, before, during, and after phone usage. Additionally, we propose an approach for estimating head pose events using images taken by the webcam during the MOOC learning sessions to detect phone-usage events. Our hypothesis suggests that head posture undergoes significant changes when learners interact with a mobile phone, contrasting with the typical behavior seen when learners face a computer during e-learning sessions. We propose an approach designed to detect deviations in head posture from the average observed during a learner's session, operating as a semi-supervised method. This system flags events indicating alterations in head posture for subsequent human review and selection of mobile phone usage occurrences with a sensitivity over 90
MoreTranslated text
Key words
biometrics,head pose,machine learning,multi-modal learning,online learning,phone usage
PDF
View via Publisher
AI Read Science
AI Summary
AI Summary is the key point extracted automatically understanding the full text of the paper, including the background, methods, results, conclusions, icons and other key content, so that you can get the outline of the paper at a glance.
Example
Background
Key content
Introduction
Methods
Results
Related work
Fund
Key content
- Pretraining has recently greatly promoted the development of natural language processing (NLP)
- We show that M6 outperforms the baselines in multimodal downstream tasks, and the large M6 with 10 parameters can reach a better performance
- We propose a method called M6 that is able to process information of multiple modalities and perform both single-modal and cross-modal understanding and generation
- The model is scaled to large model with 10 billion parameters with sophisticated deployment, and the 10 -parameter M6-large is the largest pretrained model in Chinese
- Experimental results show that our proposed M6 outperforms the baseline in a number of downstream tasks concerning both single modality and multiple modalities We will continue the pretraining of extremely large models by increasing data to explore the limit of its performance
Try using models to generate summary,it takes about 60s
Must-Reading Tree
Example
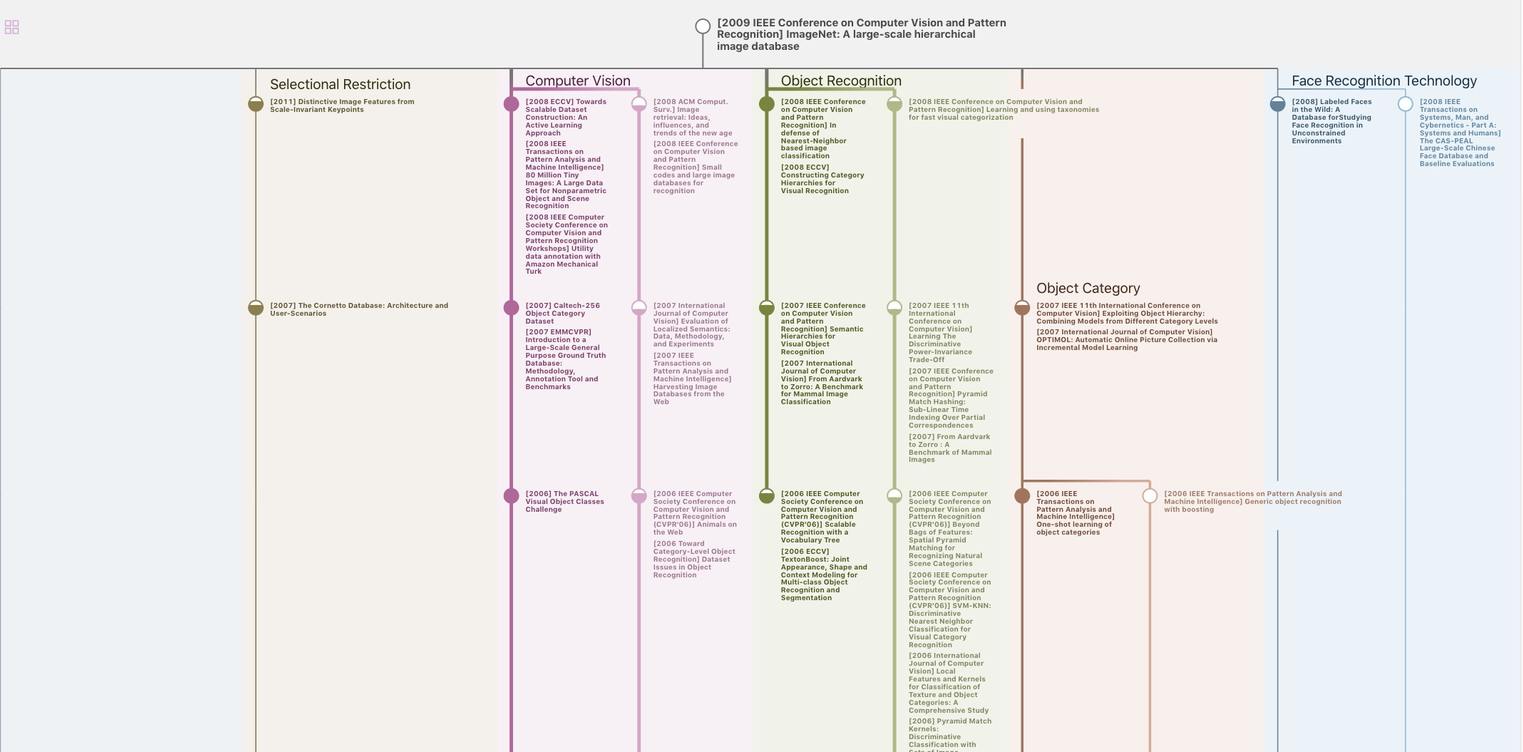
Generate MRT to find the research sequence of this paper
Related Papers
Data Disclaimer
The page data are from open Internet sources, cooperative publishers and automatic analysis results through AI technology. We do not make any commitments and guarantees for the validity, accuracy, correctness, reliability, completeness and timeliness of the page data. If you have any questions, please contact us by email: report@aminer.cn
Chat Paper
去 AI 文献库 对话