Transformer-based Pavement Crack Tracking with Neural-PID Controller on Vision-guided Robot
Proceedings of the International Symposium on Automation and Robotics in Construction (IAARC) Proceedings of the 41st International Symposium on Automation and Robotics in Construction(2024)
Abstract
Transformer-based Pavement Crack Tracking with Neural-PID Controller on Vision-guided Robot Jianqi Zhang, Xu Yang, Wei Wang, Ioannis Brilakis, Hainian Wang, Ling Ding Pages 699-706 (2024 Proceedings of the 41st ISARC, Lille, France, ISBN 978-0-6458322-1-1, ISSN 2413-5844) Abstract: Pavement crack tracking in unstructured road environments stands as a crucial and ongoing challenge, playing a pivotal role in ensuring precise crack sealing for automated pavement repair. However, slender cracks often present issues with insufficient feature extraction and reduced tracking efficiency. This article proposes a dynamic visual tracking method for pavement cracks utilizing a hybrid adaptive control scheme combined with a self-tuning neural network and proportional-integral-derivative (PID). Specifically, the system capitalizes on a transformer-based crack segmentation system to extract crack features from the road image plane and determine an optimized control input to direct the robot. Segmentation module cross-integrates coarse-grained and fine-grained features using the self-attention (SA) and cross-attention (CA) for fusion operations within the fusion transformer module. Crack tracking is additionally equipped with a Neural–PID controller for adaptable control parameter adjustment. The effectiveness of this proposed method, tested thoroughly on a physical robot platform, illustrates significantly positive results in achieving real-time tracking of pavement cracks. Keywords: Crack Tracking, Crack Segmentation, Transformer, Neural–PID Control, Mobile Robot DOI: https://doi.org/10.22260/ISARC2024/0091 Download fulltext Download BibTex Download Endnote (RIS) TeX Import to Mendeley
MoreTranslated text
求助PDF
上传PDF
View via Publisher
AI Read Science
AI Summary
AI Summary is the key point extracted automatically understanding the full text of the paper, including the background, methods, results, conclusions, icons and other key content, so that you can get the outline of the paper at a glance.
Example
Background
Key content
Introduction
Methods
Results
Related work
Fund
Key content
- Pretraining has recently greatly promoted the development of natural language processing (NLP)
- We show that M6 outperforms the baselines in multimodal downstream tasks, and the large M6 with 10 parameters can reach a better performance
- We propose a method called M6 that is able to process information of multiple modalities and perform both single-modal and cross-modal understanding and generation
- The model is scaled to large model with 10 billion parameters with sophisticated deployment, and the 10 -parameter M6-large is the largest pretrained model in Chinese
- Experimental results show that our proposed M6 outperforms the baseline in a number of downstream tasks concerning both single modality and multiple modalities We will continue the pretraining of extremely large models by increasing data to explore the limit of its performance
Upload PDF to Generate Summary
Must-Reading Tree
Example
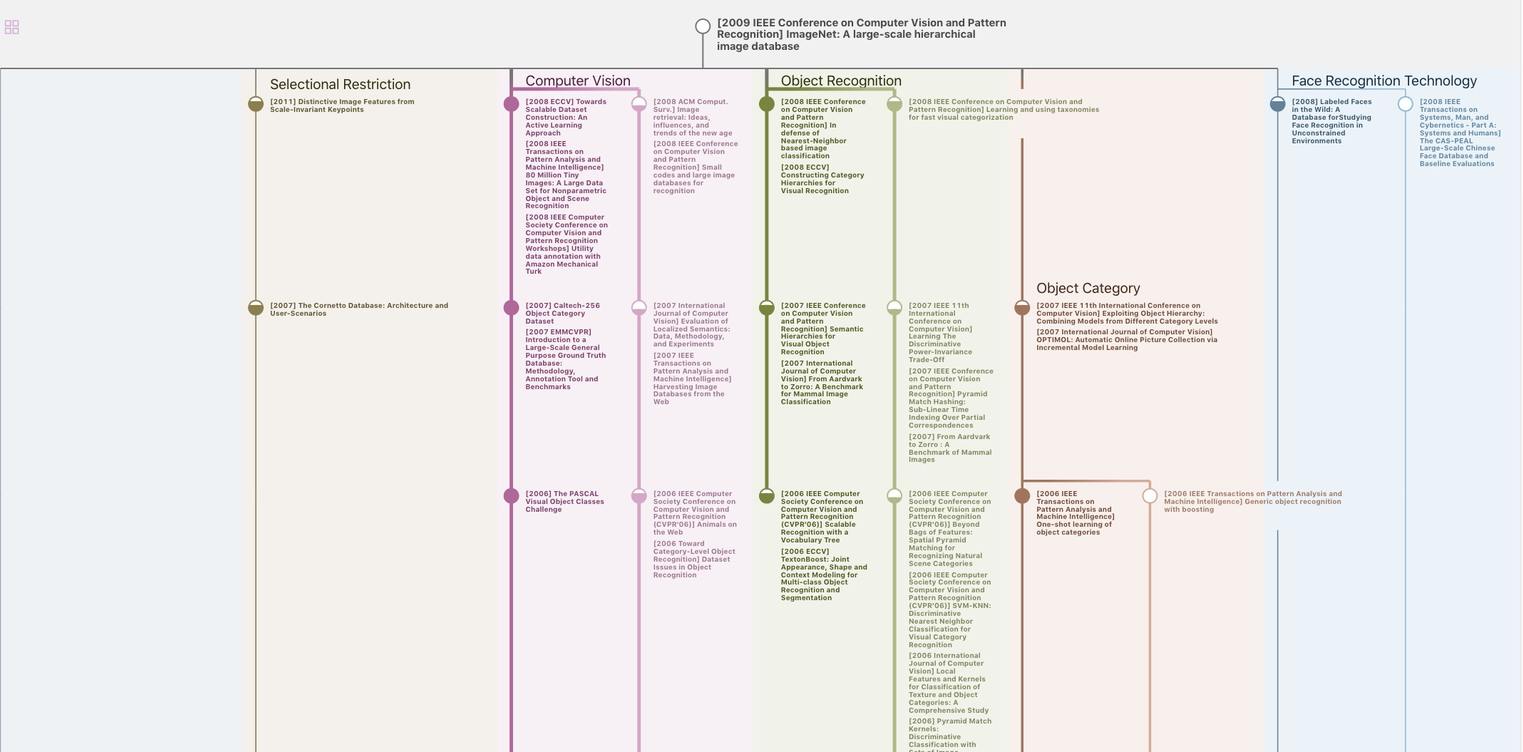
Generate MRT to find the research sequence of this paper
Data Disclaimer
The page data are from open Internet sources, cooperative publishers and automatic analysis results through AI technology. We do not make any commitments and guarantees for the validity, accuracy, correctness, reliability, completeness and timeliness of the page data. If you have any questions, please contact us by email: report@aminer.cn
Chat Paper
GPU is busy, summary generation fails
Rerequest