3D Deployment and Energy Efficiency Optimization Based on DRL for RIS-assisted Air-to-Ground Communications Networks
IEEE TRANSACTIONS ON VEHICULAR TECHNOLOGY(2024)
Key Laboratory of Information and Communication Systems | Key Laboratory of Universal Wireless Communications | Trobe Univ
Abstract
Research indicates that replacing relays with the reconfigurable intelligent surface (RIS) can effectively reduce energy consumption under certain conditions. Therefore, introducing RIS into unmanned aerial vehicle (UAV) assisted airto- ground communication networks can further enhance communication performance. In this paper, we propose a RISUAV- assisted communication network scenario that utilizes the zero-forcing (ZF) precoding method to eliminate multi-user interference, and then optimize the BS transmit power by using the Dinkelbach algorithm. To address the optimization problem of bandwidth allocation, RIS phase shifts, and threedimensional (3D) coordinates of the RIS-UAV, we propose two deep reinforcement learning (DRL) algorithms, which are termed D3QN-MM and D3QN-Pure, respectively. Both D3QN-MM and D3QN-Pure utilize the dueling double deep Q-network (D3QN) for optimizing bandwidth allocation and the 3D coordinates of the RIS-UAV. However, D3QN-MM employs the traditional majorizeminimization (MM) algorithm for RIS phase shifts optimization, while D3QN-Pure utilizes the D3QN. By comparing them with other algorithms, such as the DRL algorithm, the advantages of the algorithms proposed in this paper are highlighted. Furthermore, compared to the amplify-and-forward (AF) relay, the RIS can achieve a 48% energy efficiency improvement. Besides, the D3QN-Pure algorithm provided up to 14.3% energy effciency improvement compared to the D3QN-MM algorithm.
MoreTranslated text
Key words
Autonomous aerial vehicles,Optimization,Trajectory,Three-dimensional displays,Resource management,Relays,Array signal processing,Unmanned aerial vehicle (UAV),reconfigurable intelligent surface (RIS),deep reinforcement learning (DRL),dueling double deep Q-learning (D3QN),three-dimensional (3D) deployment
求助PDF
上传PDF
View via Publisher
AI Read Science
AI Summary
AI Summary is the key point extracted automatically understanding the full text of the paper, including the background, methods, results, conclusions, icons and other key content, so that you can get the outline of the paper at a glance.
Example
Background
Key content
Introduction
Methods
Results
Related work
Fund
Key content
- Pretraining has recently greatly promoted the development of natural language processing (NLP)
- We show that M6 outperforms the baselines in multimodal downstream tasks, and the large M6 with 10 parameters can reach a better performance
- We propose a method called M6 that is able to process information of multiple modalities and perform both single-modal and cross-modal understanding and generation
- The model is scaled to large model with 10 billion parameters with sophisticated deployment, and the 10 -parameter M6-large is the largest pretrained model in Chinese
- Experimental results show that our proposed M6 outperforms the baseline in a number of downstream tasks concerning both single modality and multiple modalities We will continue the pretraining of extremely large models by increasing data to explore the limit of its performance
Upload PDF to Generate Summary
Must-Reading Tree
Example
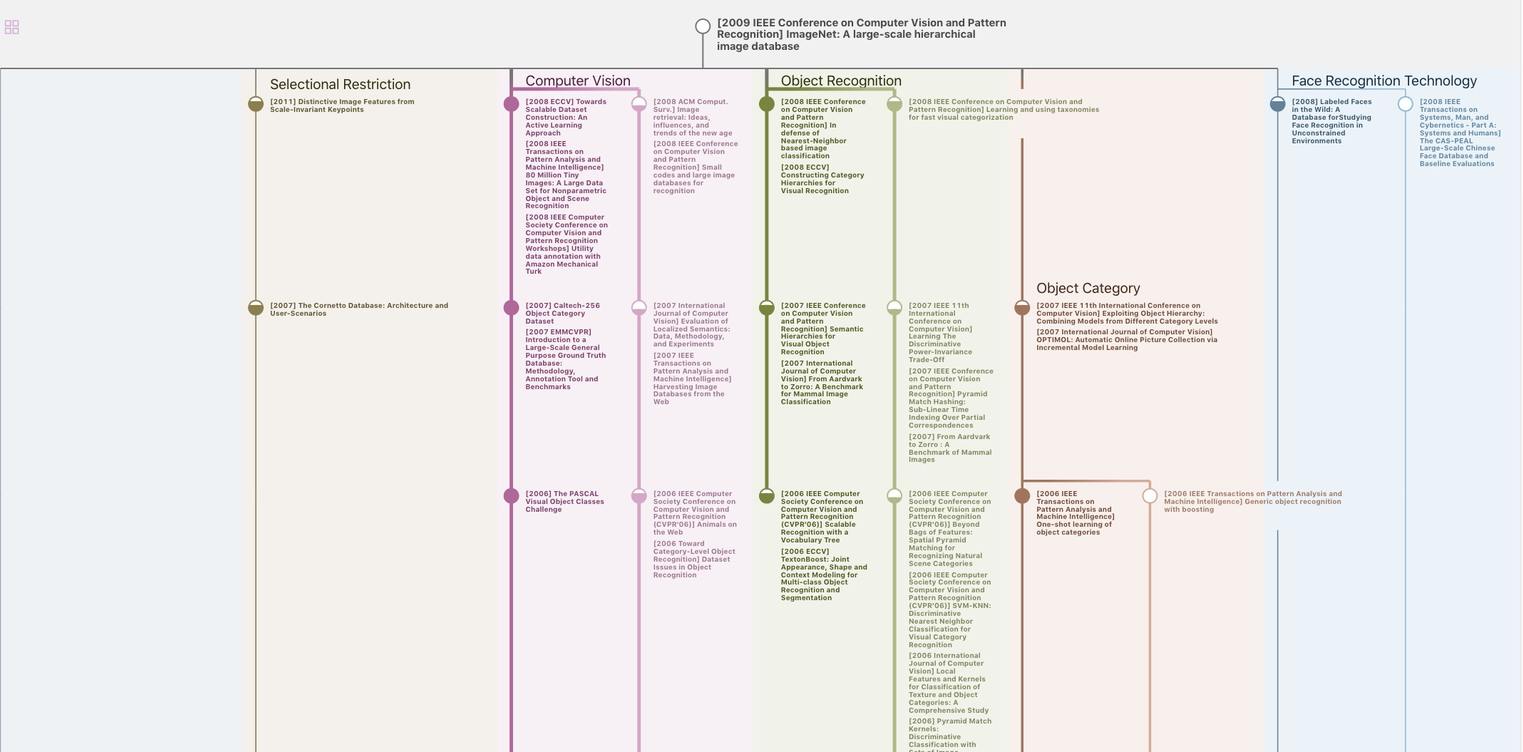
Generate MRT to find the research sequence of this paper
Data Disclaimer
The page data are from open Internet sources, cooperative publishers and automatic analysis results through AI technology. We do not make any commitments and guarantees for the validity, accuracy, correctness, reliability, completeness and timeliness of the page data. If you have any questions, please contact us by email: report@aminer.cn
Chat Paper