Encoding Hierarchical Schema Via Concept Flow for Multifaceted Ideology Detection
Annual Meeting of the Association for Computational Linguistics(2024)
Abstract
Multifaceted ideology detection (MID) aims to detect the ideological leaningsof texts towards multiple facets. Previous studies on ideology detection mainlyfocus on one generic facet and ignore label semantics and explanatorydescriptions of ideologies, which are a kind of instructive information andreveal the specific concepts of ideologies. In this paper, we develop a novelconcept semantics-enhanced framework for the MID task. Specifically, we proposea bidirectional iterative concept flow (BICo) method to encode multifacetedideologies. BICo enables the concepts to flow across levels of the schema treeand enriches concept representations with multi-granularity semantics.Furthermore, we explore concept attentive matching and concept-guidedcontrastive learning strategies to guide the model to capture ideology featureswith the learned concept semantics. Extensive experiments on the benchmarkdataset show that our approach achieves state-of-the-art performance in MID,including in the cross-topic scenario.
MoreTranslated text
PDF
View via Publisher
AI Read Science
AI Summary
AI Summary is the key point extracted automatically understanding the full text of the paper, including the background, methods, results, conclusions, icons and other key content, so that you can get the outline of the paper at a glance.
Example
Background
Key content
Introduction
Methods
Results
Related work
Fund
Key content
- Pretraining has recently greatly promoted the development of natural language processing (NLP)
- We show that M6 outperforms the baselines in multimodal downstream tasks, and the large M6 with 10 parameters can reach a better performance
- We propose a method called M6 that is able to process information of multiple modalities and perform both single-modal and cross-modal understanding and generation
- The model is scaled to large model with 10 billion parameters with sophisticated deployment, and the 10 -parameter M6-large is the largest pretrained model in Chinese
- Experimental results show that our proposed M6 outperforms the baseline in a number of downstream tasks concerning both single modality and multiple modalities We will continue the pretraining of extremely large models by increasing data to explore the limit of its performance
Try using models to generate summary,it takes about 60s
Must-Reading Tree
Example
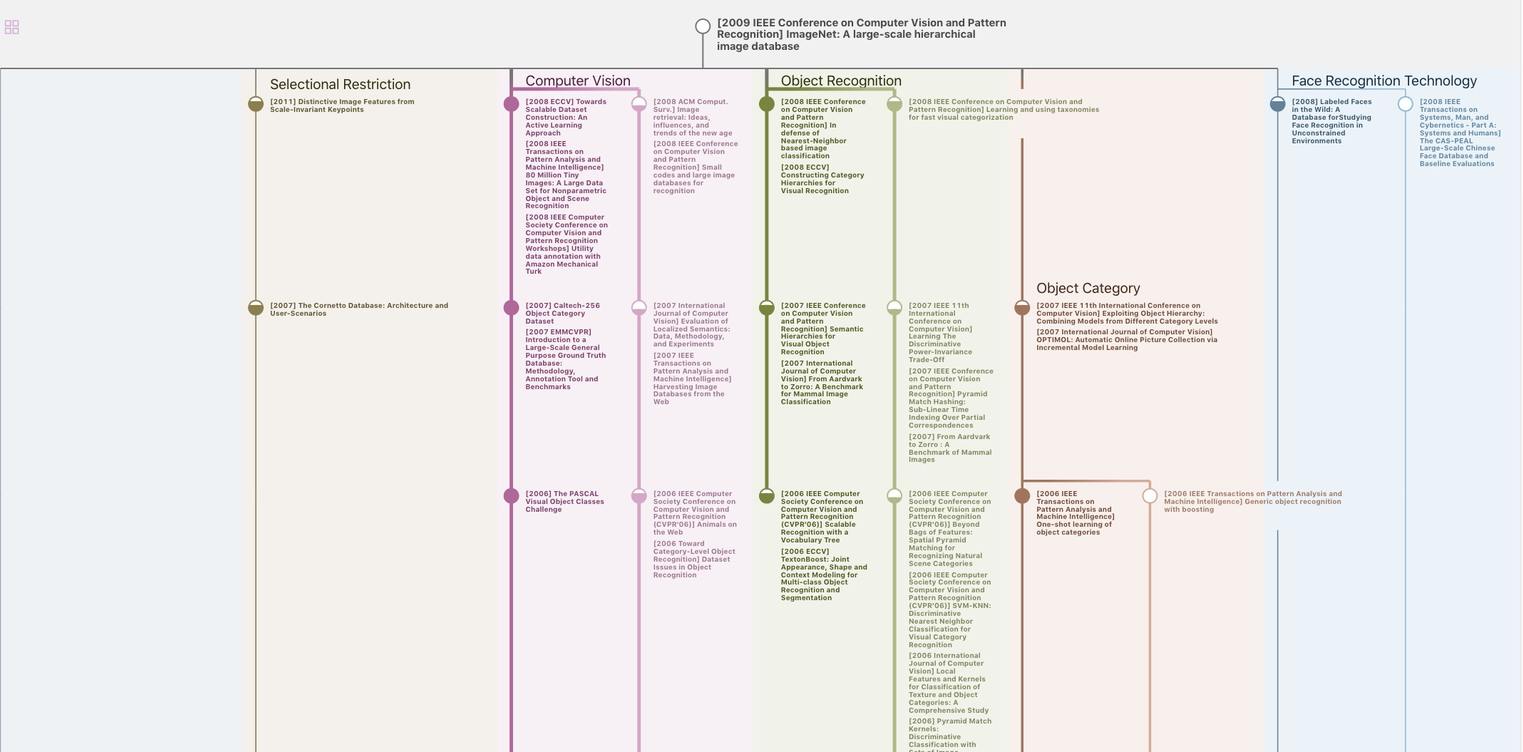
Generate MRT to find the research sequence of this paper
Data Disclaimer
The page data are from open Internet sources, cooperative publishers and automatic analysis results through AI technology. We do not make any commitments and guarantees for the validity, accuracy, correctness, reliability, completeness and timeliness of the page data. If you have any questions, please contact us by email: report@aminer.cn
Chat Paper
GPU is busy, summary generation fails
Rerequest