Integrating Multi-scale Contextualized Information for Byte-based Neural Machine Translation
Annual Meeting of the Association for Computational Linguistics(2024)
AI 理解论文
溯源树
样例
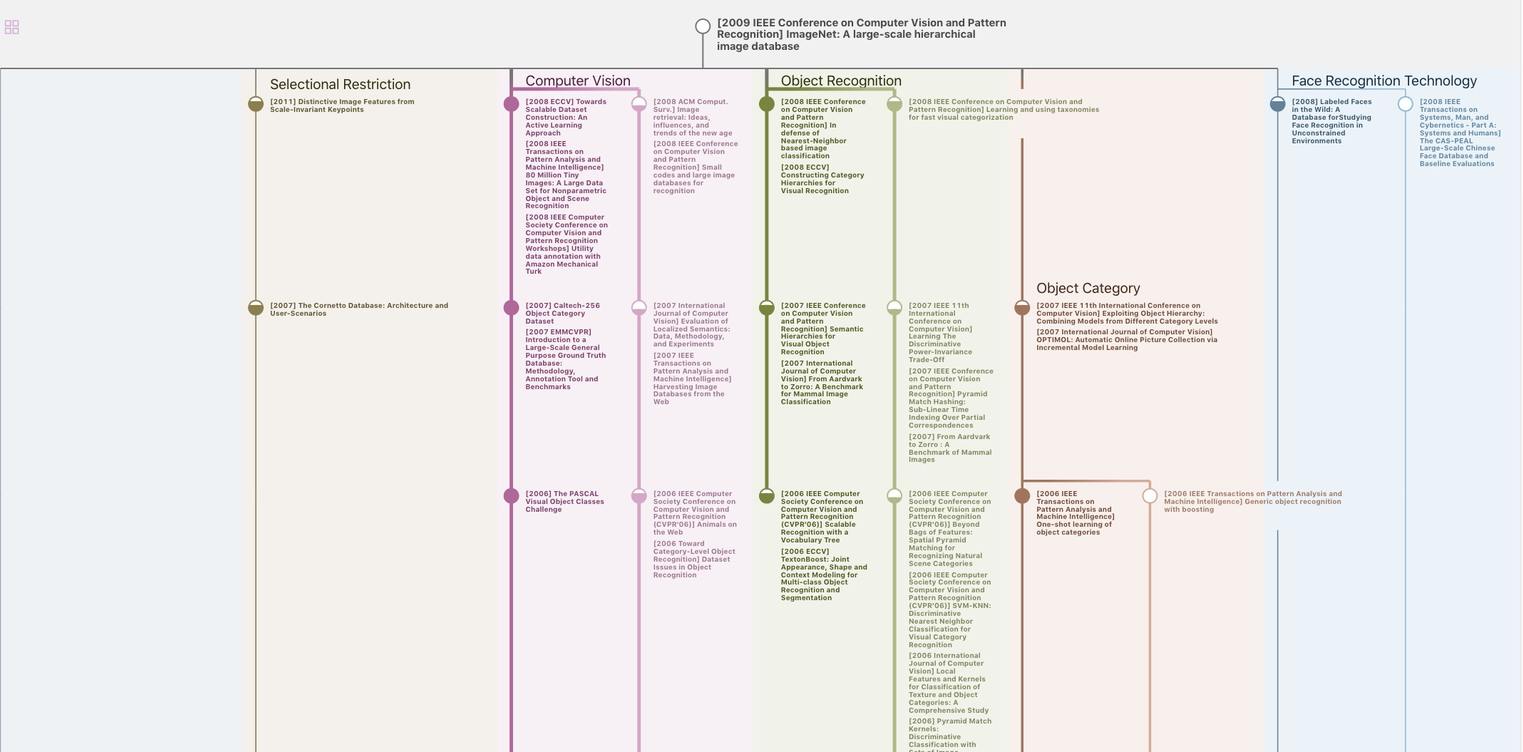
生成溯源树,研究论文发展脉络
Chat Paper
正在生成论文摘要
Annual Meeting of the Association for Computational Linguistics(2024)