An Asset Subset-Constrained Minimax Optimization Framework for Online Portfolio Selection
EXPERT SYSTEMS WITH APPLICATIONS(2024)
Abstract
Effective online portfolio selection necessitates seamless integration of three key properties: diversity, sparsity, and risk control. However, existing algorithms often prioritize one property at the expense of the others due to inherent conflicts. To address this issue, we propose an asset subset-constrained minimax (ASCM) optimization framework, which generates optimal portfolios from diverse investment strategies represented as asset subsets. ASCM consists of: (i) a minimax optimization model that focuses on risk control by considering a set of loss functions constrained by different asset subsets; (ii) the construction of asset subsets via price-feature clipping, which effectively reduces redundant assets in the portfolio; (iii) a state-based estimation of price trends that guides all ASCM loss functions, facilitating the generation of sparse solutions. We solve the ASCM minimax model using an efficient iterative updating formula derived from the projected subgradient method. Furthermore, we achieve near O(1) time complexity through a novel initialization scheme. Experimental results demonstrate that ASCM outperforms eight representative algorithms, including the best constant rebalanced portfolio in hindsight (BCRP) on five out of the six real-world financial datasets. Notably, ASCM achieves a 67-fold improvement over BCRP in cumulative wealth on the TSE dataset.
MoreTranslated text
Key words
Asset subset-constrained online portfolio selection,Minimax optimization,Robust online portfolio selection,Projected subgradient method
求助PDF
上传PDF
View via Publisher
AI Read Science
AI Summary
AI Summary is the key point extracted automatically understanding the full text of the paper, including the background, methods, results, conclusions, icons and other key content, so that you can get the outline of the paper at a glance.
Example
Background
Key content
Introduction
Methods
Results
Related work
Fund
Key content
- Pretraining has recently greatly promoted the development of natural language processing (NLP)
- We show that M6 outperforms the baselines in multimodal downstream tasks, and the large M6 with 10 parameters can reach a better performance
- We propose a method called M6 that is able to process information of multiple modalities and perform both single-modal and cross-modal understanding and generation
- The model is scaled to large model with 10 billion parameters with sophisticated deployment, and the 10 -parameter M6-large is the largest pretrained model in Chinese
- Experimental results show that our proposed M6 outperforms the baseline in a number of downstream tasks concerning both single modality and multiple modalities We will continue the pretraining of extremely large models by increasing data to explore the limit of its performance
Upload PDF to Generate Summary
Must-Reading Tree
Example
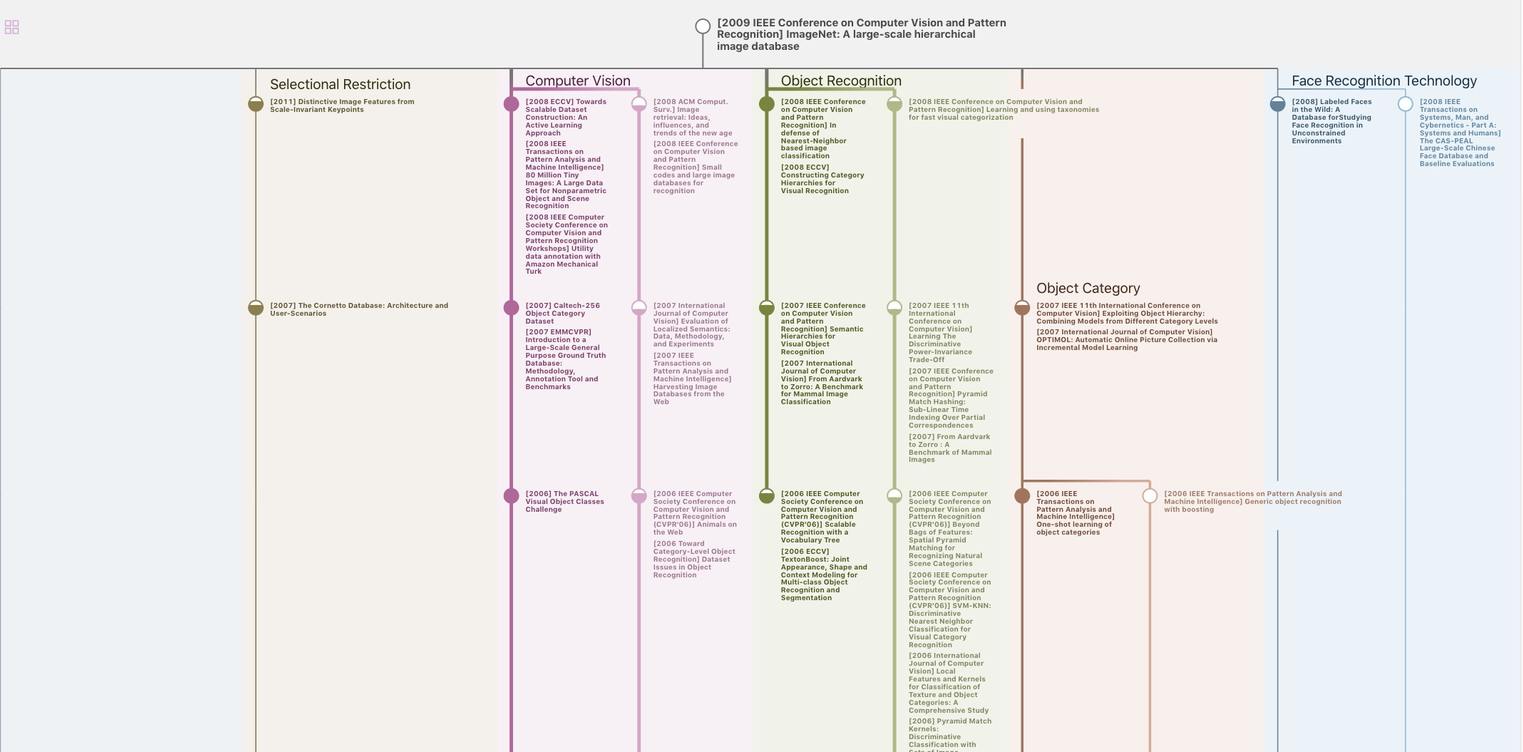
Generate MRT to find the research sequence of this paper
Related Papers
2024
被引用58 | 浏览
2012
被引用59 | 浏览
2017
被引用139 | 浏览
2021
被引用21 | 浏览
2021
被引用3 | 浏览
2021
被引用12 | 浏览
2023
被引用9 | 浏览
2023
被引用3 | 浏览
2024
被引用10 | 浏览
2022
被引用39 | 浏览
2023
被引用6 | 浏览
Data Disclaimer
The page data are from open Internet sources, cooperative publishers and automatic analysis results through AI technology. We do not make any commitments and guarantees for the validity, accuracy, correctness, reliability, completeness and timeliness of the page data. If you have any questions, please contact us by email: report@aminer.cn
Chat Paper