X-Instruction: Aligning Language Model in Low-resource Languages with Self-curated Cross-lingual Instructions
Annual Meeting of the Association for Computational Linguistics(2024)
Abstract
Large language models respond well in high-resource languages like Englishbut struggle in low-resource languages. It may arise from the lack ofhigh-quality instruction following data in these languages. Directlytranslating English samples into these languages can be a solution butunreliable, leading to responses with translation errors and lackinglanguage-specific or cultural knowledge. To address this issue, we propose anovel method to construct cross-lingual instruction following samples withinstruction in English and response in low-resource languages. Specifically,the language model first learns to generate appropriate English instructionsaccording to the natural web texts in other languages as responses. Thecandidate cross-lingual instruction tuning samples are further refined anddiversified. We have employed this method to build a large-scale cross-lingualinstruction tuning dataset on 10 languages, namely X-Instruction. Theinstruction data built using our method incorporate more language-specificknowledge compared with the naive translation method. Experimental results haveshown that the response quality of the model tuned on X-Instruction greatlyexceeds the model distilled from a powerful teacher model, reaching or evensurpassing the ones of ChatGPT. In addition, we find that models tuned oncross-lingual instruction following samples can follow the instruction in theoutput language without further tuning.
MoreTranslated text
PDF
View via Publisher
AI Read Science
AI Summary
AI Summary is the key point extracted automatically understanding the full text of the paper, including the background, methods, results, conclusions, icons and other key content, so that you can get the outline of the paper at a glance.
Example
Background
Key content
Introduction
Methods
Results
Related work
Fund
Key content
- Pretraining has recently greatly promoted the development of natural language processing (NLP)
- We show that M6 outperforms the baselines in multimodal downstream tasks, and the large M6 with 10 parameters can reach a better performance
- We propose a method called M6 that is able to process information of multiple modalities and perform both single-modal and cross-modal understanding and generation
- The model is scaled to large model with 10 billion parameters with sophisticated deployment, and the 10 -parameter M6-large is the largest pretrained model in Chinese
- Experimental results show that our proposed M6 outperforms the baseline in a number of downstream tasks concerning both single modality and multiple modalities We will continue the pretraining of extremely large models by increasing data to explore the limit of its performance
Try using models to generate summary,it takes about 60s
Must-Reading Tree
Example
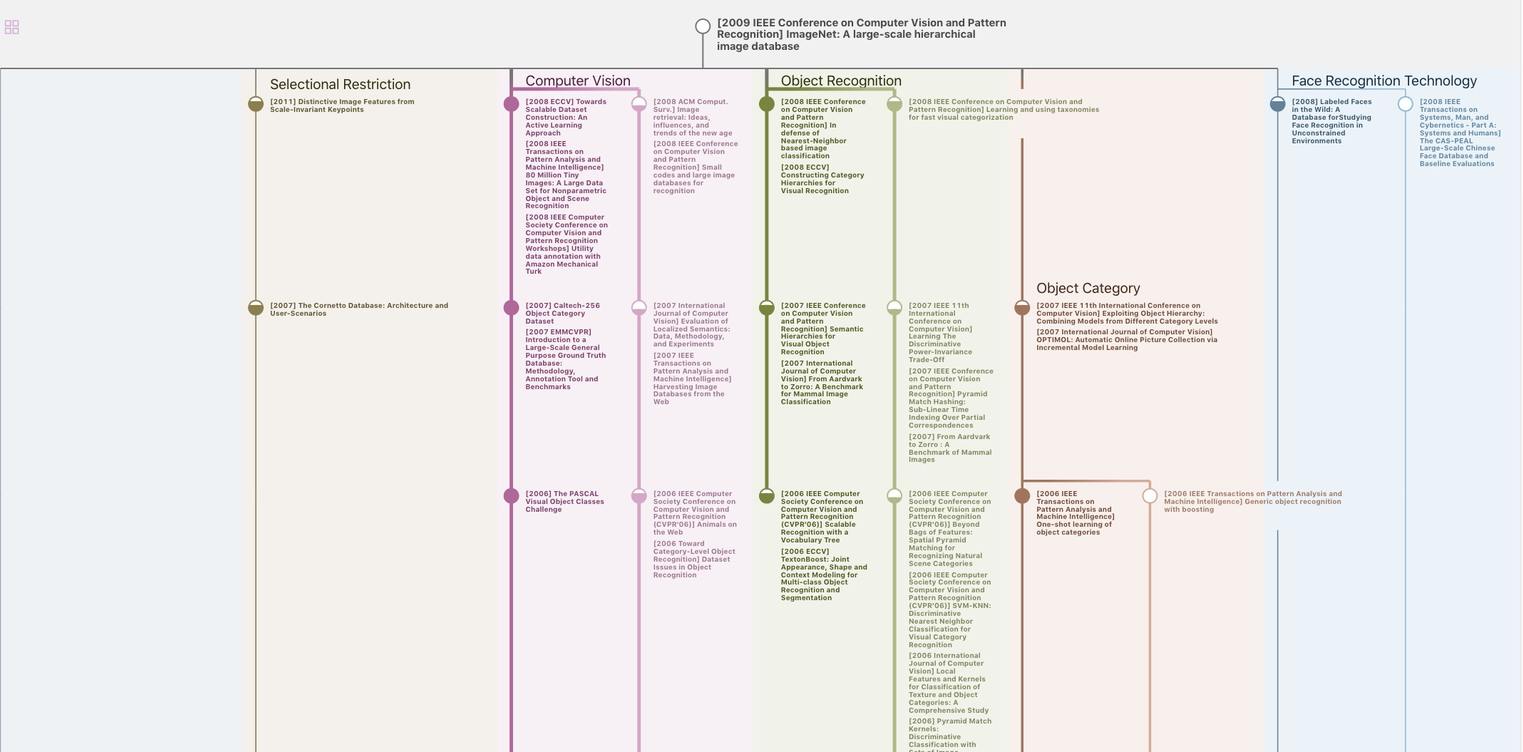
Generate MRT to find the research sequence of this paper
Related Papers
Data Disclaimer
The page data are from open Internet sources, cooperative publishers and automatic analysis results through AI technology. We do not make any commitments and guarantees for the validity, accuracy, correctness, reliability, completeness and timeliness of the page data. If you have any questions, please contact us by email: report@aminer.cn
Chat Paper
去 AI 文献库 对话