Identifying While Learning for Document Event Causality Identification
Annual Meeting of the Association for Computational Linguistics(2024)
AI 理解论文
溯源树
样例
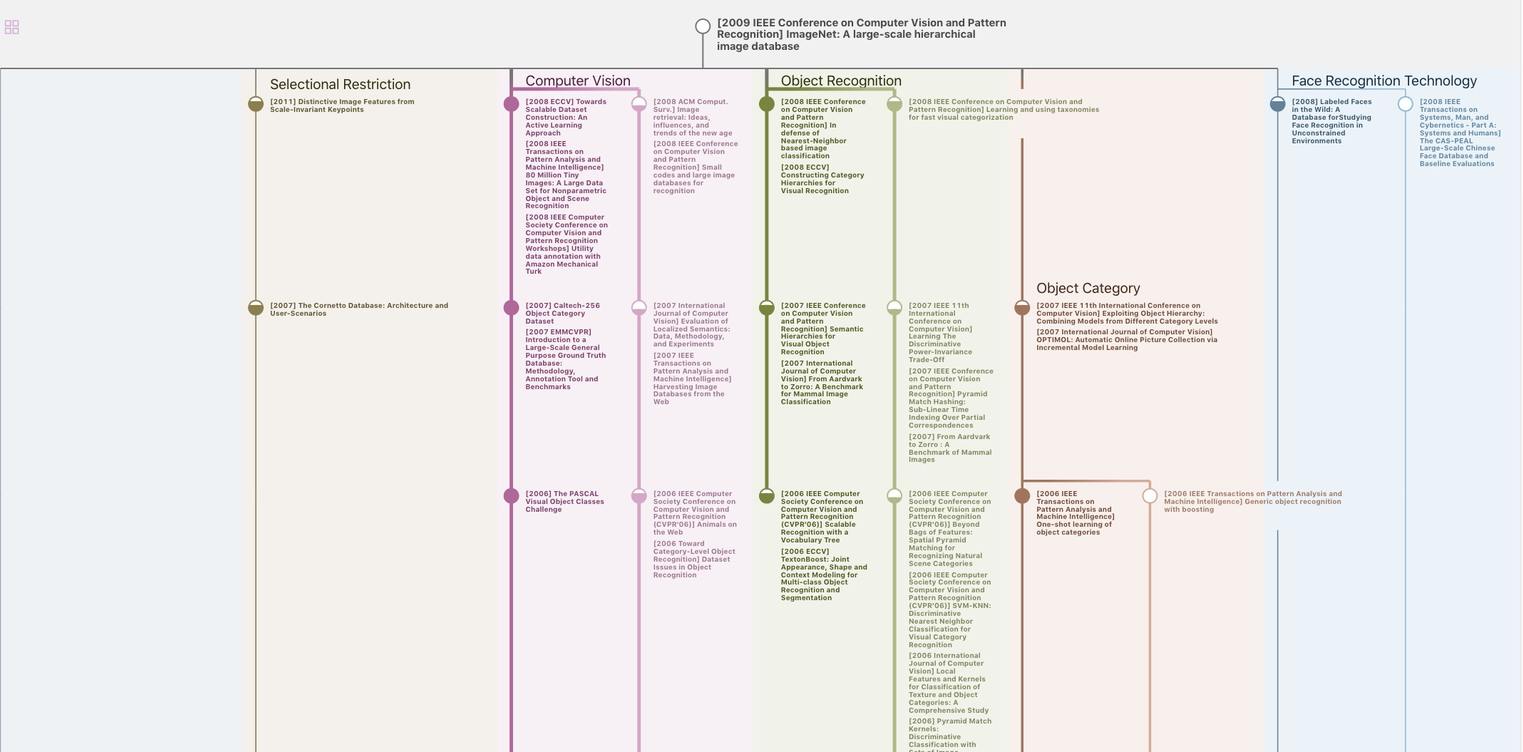
生成溯源树,研究论文发展脉络
Chat Paper
正在生成论文摘要
Annual Meeting of the Association for Computational Linguistics(2024)