MobiAir: Unleashing Sensor Mobility for City-scale and Fine-grained Air-Quality Monitoring with AirBERT
PROCEEDINGS OF THE 2024 THE 22ND ANNUAL INTERNATIONAL CONFERENCE ON MOBILE SYSTEMS, APPLICATIONS AND SERVICES, MOBISYS 2024(2024)
Abstract
Mobile air pollution sensing methods are developed to collect air quality data with higher spatial-temporal resolutions. However, existing methods cannot process the spatially mixed gas samples effectively due to the highly dynamic temporal and spatial fluctuations experienced by the sensor, leading to significant measurement deviations. We find an opportunity to tackle the problem by exploring the potential patterns from sensor measurements. In light of this, we propose MobiAir , a novel city-scale fine-grained air quality estimation system to deliver accurate mobile air quality data. First, we design AirBERT , a representation learning model to discern mixed gas concentrations. Second, we design a knowledge-informed training strategy leveraging massive unlabeled city-scale data to enhance the AirBERT performance. To ensure the practicality of MobiAir, we have invested significant efforts in implementing the software stack on our meticulously crafted Sensing Front-end , which has successfully gathered air quality data at a city-scale for more than 1200 hours. Experiments conducted on collected data show that MobiAir reduces sensing errors by 96.7% with only 44.9 ms latency, outperforming the SOTA baseline by 39.5%.
MoreTranslated text
Key words
AQI Monitoring,Mobile Crowd-Sensing,Self-supervised Learning
求助PDF
上传PDF
View via Publisher
AI Read Science
AI Summary
AI Summary is the key point extracted automatically understanding the full text of the paper, including the background, methods, results, conclusions, icons and other key content, so that you can get the outline of the paper at a glance.
Example
Background
Key content
Introduction
Methods
Results
Related work
Fund
Key content
- Pretraining has recently greatly promoted the development of natural language processing (NLP)
- We show that M6 outperforms the baselines in multimodal downstream tasks, and the large M6 with 10 parameters can reach a better performance
- We propose a method called M6 that is able to process information of multiple modalities and perform both single-modal and cross-modal understanding and generation
- The model is scaled to large model with 10 billion parameters with sophisticated deployment, and the 10 -parameter M6-large is the largest pretrained model in Chinese
- Experimental results show that our proposed M6 outperforms the baseline in a number of downstream tasks concerning both single modality and multiple modalities We will continue the pretraining of extremely large models by increasing data to explore the limit of its performance
Upload PDF to Generate Summary
Must-Reading Tree
Example
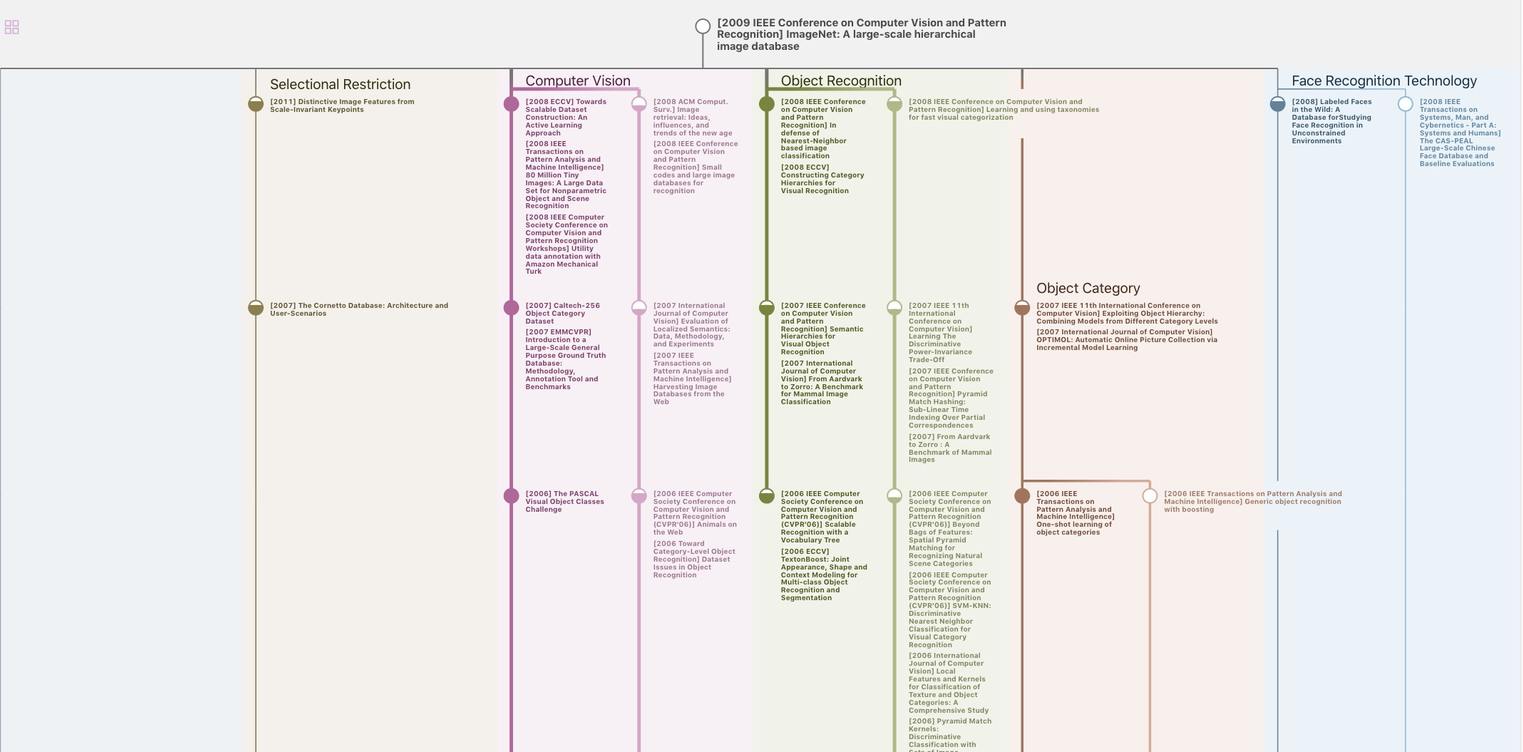
Generate MRT to find the research sequence of this paper
Related Papers
2015
被引用5628 | 浏览
Mobile Computing and Networking 2017
被引用8 | 浏览
2018
被引用15 | 浏览
Ubiquitous Computing 2018
被引用44 | 浏览
2017
被引用552 | 浏览
2019
被引用87 | 浏览
2020
被引用13 | 浏览
2020
被引用205 | 浏览
2020
被引用6 | 浏览
2022
被引用4 | 浏览
2022
被引用16 | 浏览
2023
被引用2 | 浏览
Data Disclaimer
The page data are from open Internet sources, cooperative publishers and automatic analysis results through AI technology. We do not make any commitments and guarantees for the validity, accuracy, correctness, reliability, completeness and timeliness of the page data. If you have any questions, please contact us by email: report@aminer.cn
Chat Paper