ARMA Processes with Discrete-Continuous Excitation: Compressibility Beyond Sparsity
CoRR(2024)
Abstract
Rényi Information Dimension (RID) plays a central role in quantifying the
compressibility of random variables with singularities in their distribution,
encompassing and extending beyond the class of sparse sources. The RID, from a
high perspective, presents the average number of bits that is needed for coding
the i.i.d. samples of a random variable with high precision. There are two main
extensions of the RID for stochastic processes: information dimension rate
(IDR) and block information dimension (BID). In addition, a more recent
approach towards the compressibility of stochastic processes revolves around
the concept of ϵ-achievable compression rates, which treat a random
process as the limiting point of finite-dimensional random vectors and apply
the compressed sensing tools on these random variables. While there is limited
knowledge about the interplay of the the BID, the IDR, and
ϵ-achievable compression rates, the value of IDR and BID themselves
are known only for very specific types of processes, namely i.i.d. sequences
(i.e., discrete-domain white noise) and moving-average (MA) processes. This
paper investigates the IDR and BID of discrete-time Auto-Regressive
Moving-Average (ARMA) processes in general, and their relations with
ϵ-achievable compression rates when the excitation noise has a
discrete-continuous measure. To elaborate, this paper shows that the RID and
ϵ-achievable compression rates of this type of processes are equal to
that of their excitation noise. In other words, the samples of such ARMA
processes can be compressed as much as their sparse excitation noise, although
the samples themselves are by no means sparse. The results of this paper can be
used to evaluate the compressibility of various types of locally correlated
data with finite- or infinite-memory as they are often modelled via ARMA
processes.
MoreTranslated text
PDF
View via Publisher
AI Read Science
AI Summary
AI Summary is the key point extracted automatically understanding the full text of the paper, including the background, methods, results, conclusions, icons and other key content, so that you can get the outline of the paper at a glance.
Example
Background
Key content
Introduction
Methods
Results
Related work
Fund
Key content
- Pretraining has recently greatly promoted the development of natural language processing (NLP)
- We show that M6 outperforms the baselines in multimodal downstream tasks, and the large M6 with 10 parameters can reach a better performance
- We propose a method called M6 that is able to process information of multiple modalities and perform both single-modal and cross-modal understanding and generation
- The model is scaled to large model with 10 billion parameters with sophisticated deployment, and the 10 -parameter M6-large is the largest pretrained model in Chinese
- Experimental results show that our proposed M6 outperforms the baseline in a number of downstream tasks concerning both single modality and multiple modalities We will continue the pretraining of extremely large models by increasing data to explore the limit of its performance
Try using models to generate summary,it takes about 60s
Must-Reading Tree
Example
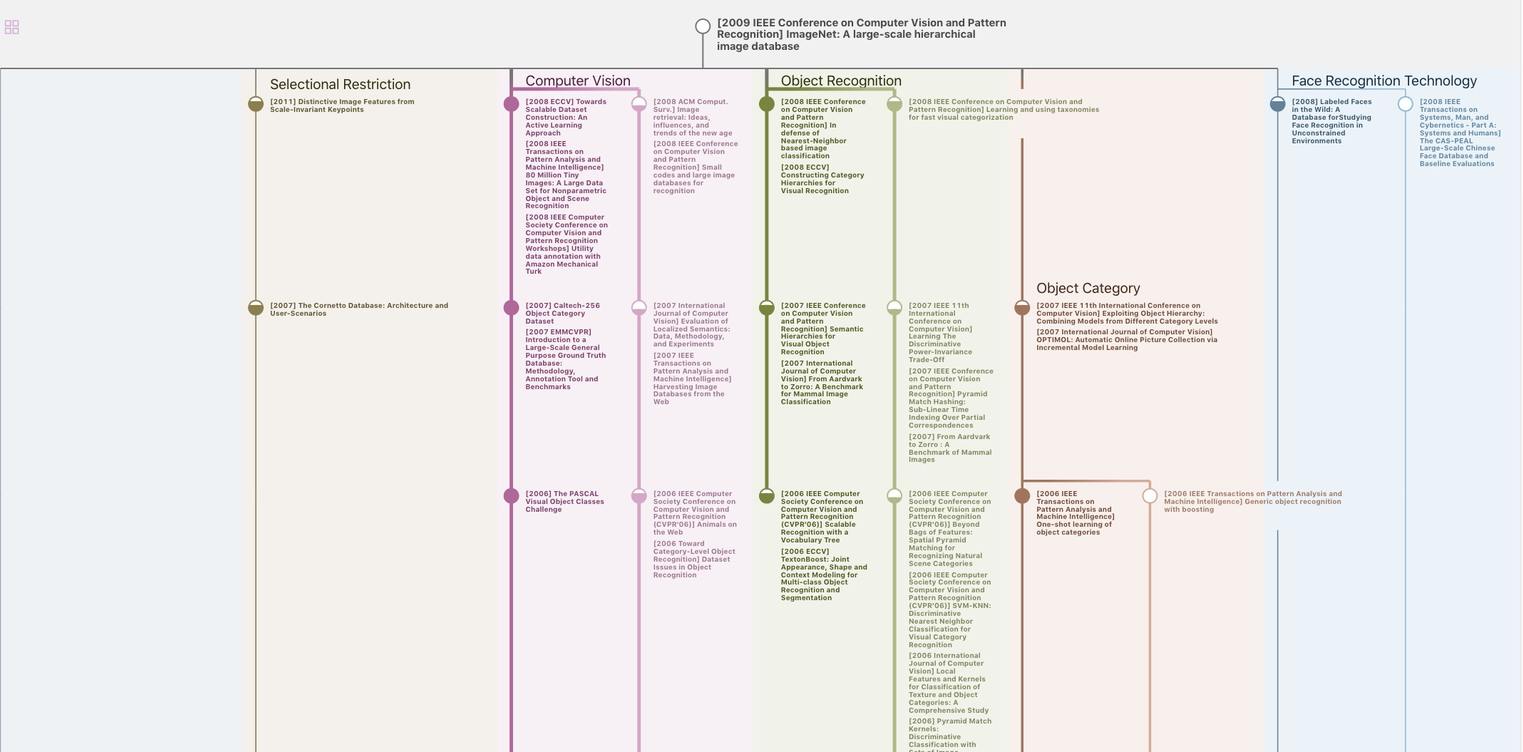
Generate MRT to find the research sequence of this paper
Data Disclaimer
The page data are from open Internet sources, cooperative publishers and automatic analysis results through AI technology. We do not make any commitments and guarantees for the validity, accuracy, correctness, reliability, completeness and timeliness of the page data. If you have any questions, please contact us by email: report@aminer.cn
Chat Paper
GPU is busy, summary generation fails
Rerequest