Coordinated Trading Strategies for Battery Storage in Reserve and Spot Markets
arXiv · Methodology(2024)
Abstract
Quantity and price risks are key uncertainties market participants face in electricity markets with increased volatility, for instance, due to high shares of renewables. From day ahead until real-time, there is a large variation in the best available information, leading to price changes that flexible assets, such as battery storage, can exploit economically. This study contributes to understanding how coordinated bidding strategies can enhance multi-market trading and large-scale energy storage integration. Our findings shed light on the complexities arising from interdependencies and the high-dimensional nature of the problem. We show how stochastic dual dynamic programming is a suitable solution technique for such an environment. We include the three markets of the frequency containment reserve, day-ahead, and intraday in stochastic modelling and develop a multi-stage stochastic program. Prices are represented in a multidimensional Markov Chain, following the scheduling of the markets and allowing for time-dependent randomness. Using the example of a battery storage in the German energy sector, we provide valuable insights into the technical aspects of our method and the economic feasibility of battery storage operation. We find that capacity reservation in the frequency containment reserve dominates over the battery's cycling in spot markets at the given resolution on prices in 2022. In an adjusted price environment, we find that coordination can yield an additional value of up to 12.5
MoreTranslated text
PDF
View via Publisher
AI Read Science
Must-Reading Tree
Example
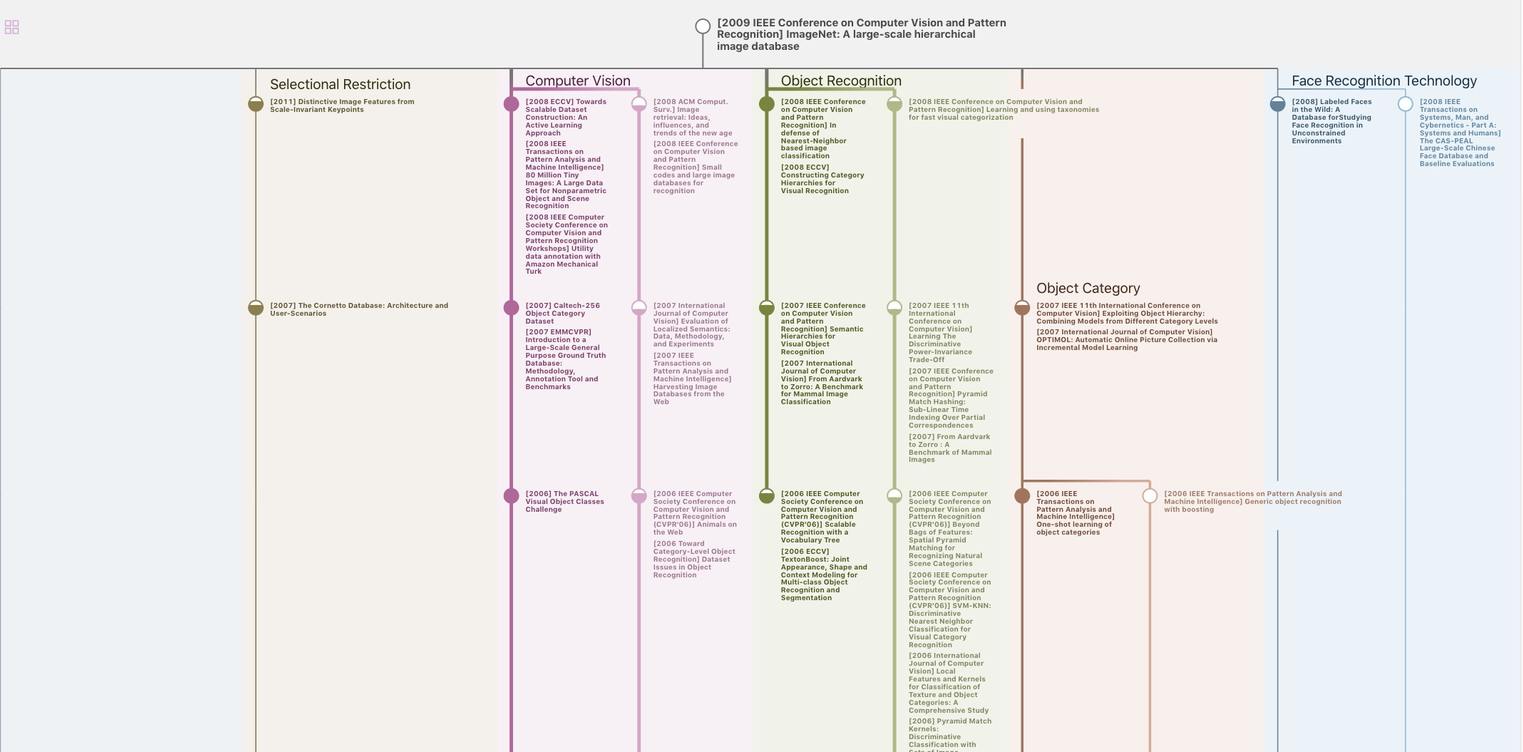
Generate MRT to find the research sequence of this paper
Data Disclaimer
The page data are from open Internet sources, cooperative publishers and automatic analysis results through AI technology. We do not make any commitments and guarantees for the validity, accuracy, correctness, reliability, completeness and timeliness of the page data. If you have any questions, please contact us by email: report@aminer.cn
Chat Paper
去 AI 文献库 对话