A First Look at IPv6 Hypergiant Infrastructure
Proceedings of the ACM on Networking(2024)
Abstract
Today's Internet is dominated by a small number of companies which are responsible for a large fraction of Internet traffic. These so called "hypergiants" make use of off-nets to deploy parts of their infrastructure in ISP networks. Off-nets ensure that clients from these ISPs get lower latencies and the ISP needs to send less traffic to its upstream providers. They have been relatively well studied in the IPv4 Internet, although their footprint in IPv6 remains unclear. In this paper, we take a first look at the IPv6 hypergiant infrastructure. We perform a first-of-its-kind study of IPv6 off-nets for 14 hypergiants and compare their deployment to IPv4. We find IPv6 off-nets in 2k ASes, compared to the more than 6k off-net ASes for IPv4. Moreover, the majority of IPv6 off-nets deployments are seen in ASes which already deploy IPv4 off-nets. Interestingly, we also see some hypergiants such as Disney and Hulu not making use of any IPv6 off-nets at all. We also uncover the phenomenon of cross-hypergiant deployments, where one hypergiant deploys its infrastructure in another hypergiant's network. Finally, we use latency measurements to compare IPv6 vs. IPv4 latency to off-net prefixes within off-net ASes and find similar results for both protocol versions. We make all our code and data available to encourage replicability.
MoreTranslated text
Key words
CDN infrastructure,IPv6,internet measurement
PDF
View via Publisher
AI Read Science
AI Summary
AI Summary is the key point extracted automatically understanding the full text of the paper, including the background, methods, results, conclusions, icons and other key content, so that you can get the outline of the paper at a glance.
Example
Background
Key content
Introduction
Methods
Results
Related work
Fund
Key content
- Pretraining has recently greatly promoted the development of natural language processing (NLP)
- We show that M6 outperforms the baselines in multimodal downstream tasks, and the large M6 with 10 parameters can reach a better performance
- We propose a method called M6 that is able to process information of multiple modalities and perform both single-modal and cross-modal understanding and generation
- The model is scaled to large model with 10 billion parameters with sophisticated deployment, and the 10 -parameter M6-large is the largest pretrained model in Chinese
- Experimental results show that our proposed M6 outperforms the baseline in a number of downstream tasks concerning both single modality and multiple modalities We will continue the pretraining of extremely large models by increasing data to explore the limit of its performance
Try using models to generate summary,it takes about 60s
Must-Reading Tree
Example
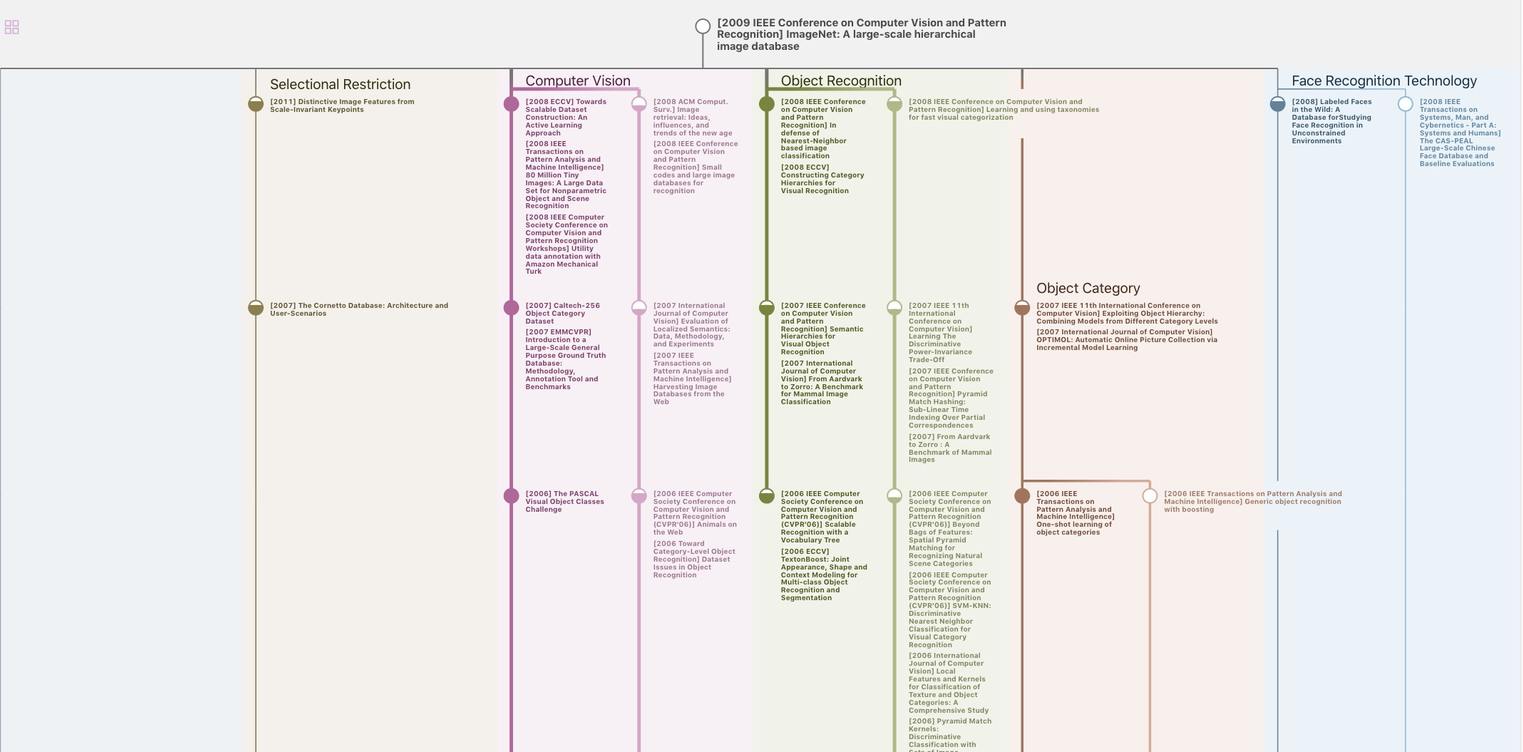
Generate MRT to find the research sequence of this paper
Data Disclaimer
The page data are from open Internet sources, cooperative publishers and automatic analysis results through AI technology. We do not make any commitments and guarantees for the validity, accuracy, correctness, reliability, completeness and timeliness of the page data. If you have any questions, please contact us by email: report@aminer.cn
Chat Paper
GPU is busy, summary generation fails
Rerequest