Investigating the Role of Instruction Variety and Task Difficulty in Robotic Manipulation Tasks
EMNLP 2024(2024)
Abstract
Evaluating the generalisation capabilities of multimodal models based solely on their performance on out-of-distribution data fails to capture their true robustness. This work introduces a comprehensive evaluation framework that systematically examines the role of instructions and inputs in the generalisation abilities of such models, considering architectural design, input perturbations across language and vision modalities, and increased task complexity. The proposed framework uncovers the resilience of multimodal models to extreme instruction perturbations and their vulnerability to observational changes, raising concerns about overfitting to spurious correlations. By employing this evaluation framework on current Transformer-based multimodal models for robotic manipulation tasks, we uncover limitations and suggest future advancements should focus on architectural and training innovations that better integrate multimodal inputs, enhancing a model's generalisation prowess by prioritising sensitivity to input content over incidental correlations.
MoreTranslated text
PDF
View via Publisher
AI Read Science
Must-Reading Tree
Example
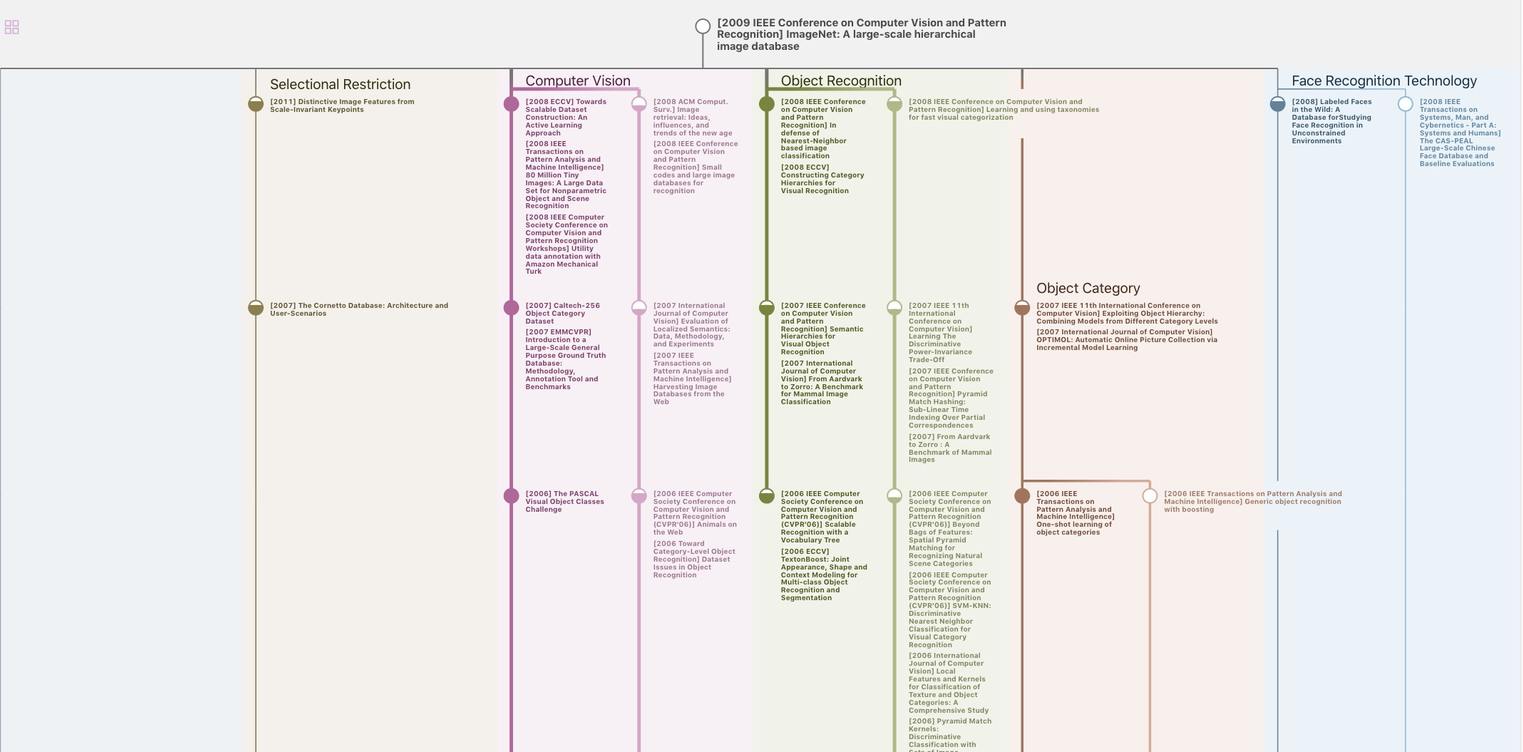
Generate MRT to find the research sequence of this paper
Data Disclaimer
The page data are from open Internet sources, cooperative publishers and automatic analysis results through AI technology. We do not make any commitments and guarantees for the validity, accuracy, correctness, reliability, completeness and timeliness of the page data. If you have any questions, please contact us by email: report@aminer.cn
Chat Paper
Summary is being generated by the instructions you defined