A Review of Crop Yield Prediction Models based on Crop Phenology Using Satellite Imagery and Environmental Data
2024 X International Conference on Information Technology and Nanotechnology (ITNT)(2024)
Key words
crop yield prediction,vegetation indices,phenology,environmental data,remote sensing,machine learning
AI Read Science
Must-Reading Tree
Example
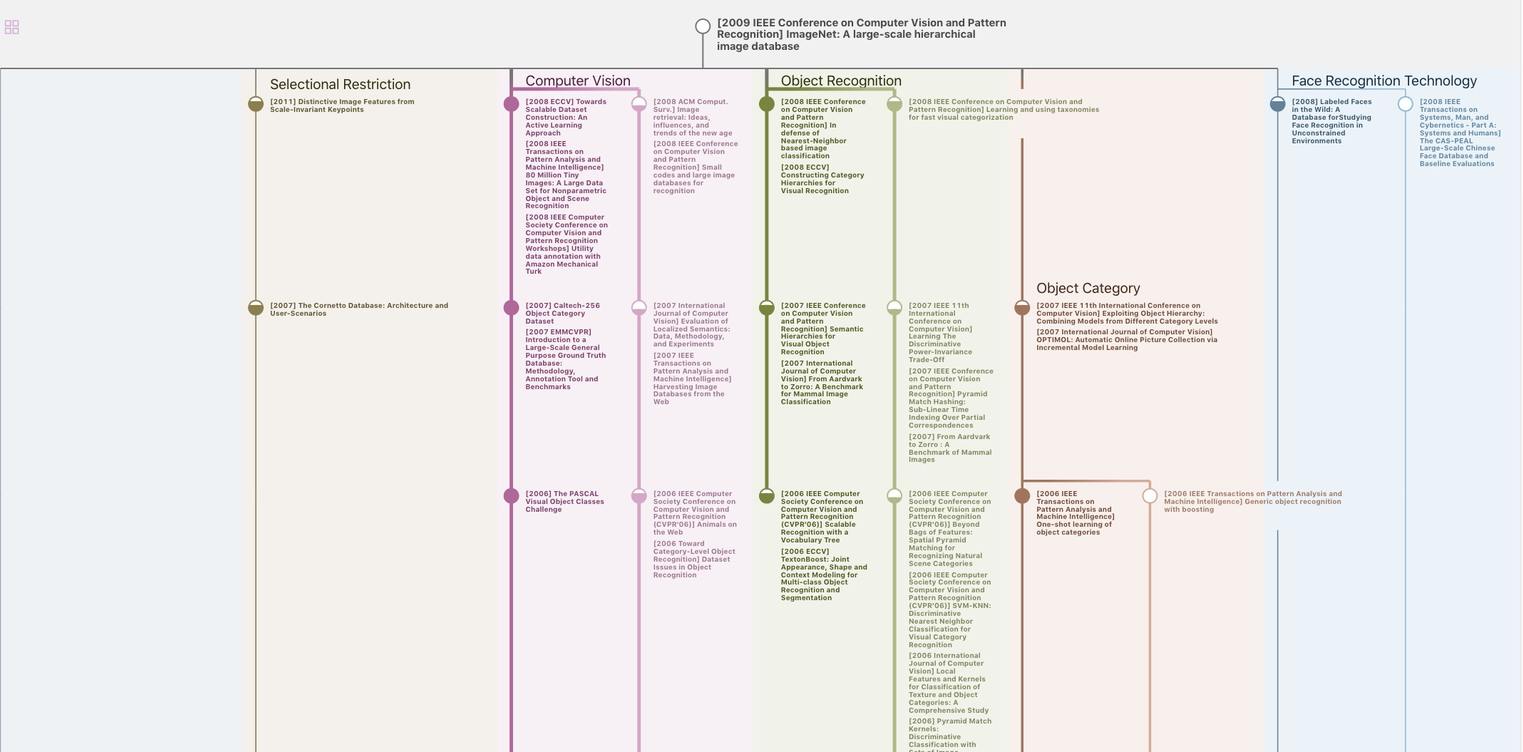
Generate MRT to find the research sequence of this paper
Chat Paper
Summary is being generated by the instructions you defined