An Autonomous Planning Model for Deploying IoT Services in Fog Computing
مهندسی مخابرات جنوب(2024)
Department of Information Technology and Computer Engineering
Abstract
IoT-based devices are constantly sending data to the cloud. However, the centralization of cloud data centers and the long distance to the location of data sources has reduced the efficiency of this paradigm in real-time applications. Fog computing can provide the resources needed by Internet of Things devices in a distributed manner at the edge of the network without involving the cloud. Therefore, processing, analysis and storage are closer to the source of data and end users cause the delay is reduced. Every Internet of Things program includes a set of Internet of Things services with different quality of service requirements, whose required resources can be provided by deploying on cloud nodes. This study deals with the challenge of locating Internet of Things services as an autonomous planning model in fog computing. We develop the colonial competition algorithm as a meta-heuristic approach to solve this problem. Since fog nodes with enough resources can host several IoT services, we consider resource distribution in the localization process. The proposed algorithm prioritizes Internet of Things services to reduce delay and solves the multi-objective positioning problem. The results of the experiments show that our algorithm can effectively improve the performance of the system and have 15% to 31% better effectiveness than the best results of the advanced algorithms in the literature.
MoreTranslated text
Key words
autonomous planning model,iot services,metaheuristic approach,fog computing
求助PDF
上传PDF
AI Read Science
AI Summary
AI Summary is the key point extracted automatically understanding the full text of the paper, including the background, methods, results, conclusions, icons and other key content, so that you can get the outline of the paper at a glance.
Example
Background
Key content
Introduction
Methods
Results
Related work
Fund
Key content
- Pretraining has recently greatly promoted the development of natural language processing (NLP)
- We show that M6 outperforms the baselines in multimodal downstream tasks, and the large M6 with 10 parameters can reach a better performance
- We propose a method called M6 that is able to process information of multiple modalities and perform both single-modal and cross-modal understanding and generation
- The model is scaled to large model with 10 billion parameters with sophisticated deployment, and the 10 -parameter M6-large is the largest pretrained model in Chinese
- Experimental results show that our proposed M6 outperforms the baseline in a number of downstream tasks concerning both single modality and multiple modalities We will continue the pretraining of extremely large models by increasing data to explore the limit of its performance
Upload PDF to Generate Summary
Must-Reading Tree
Example
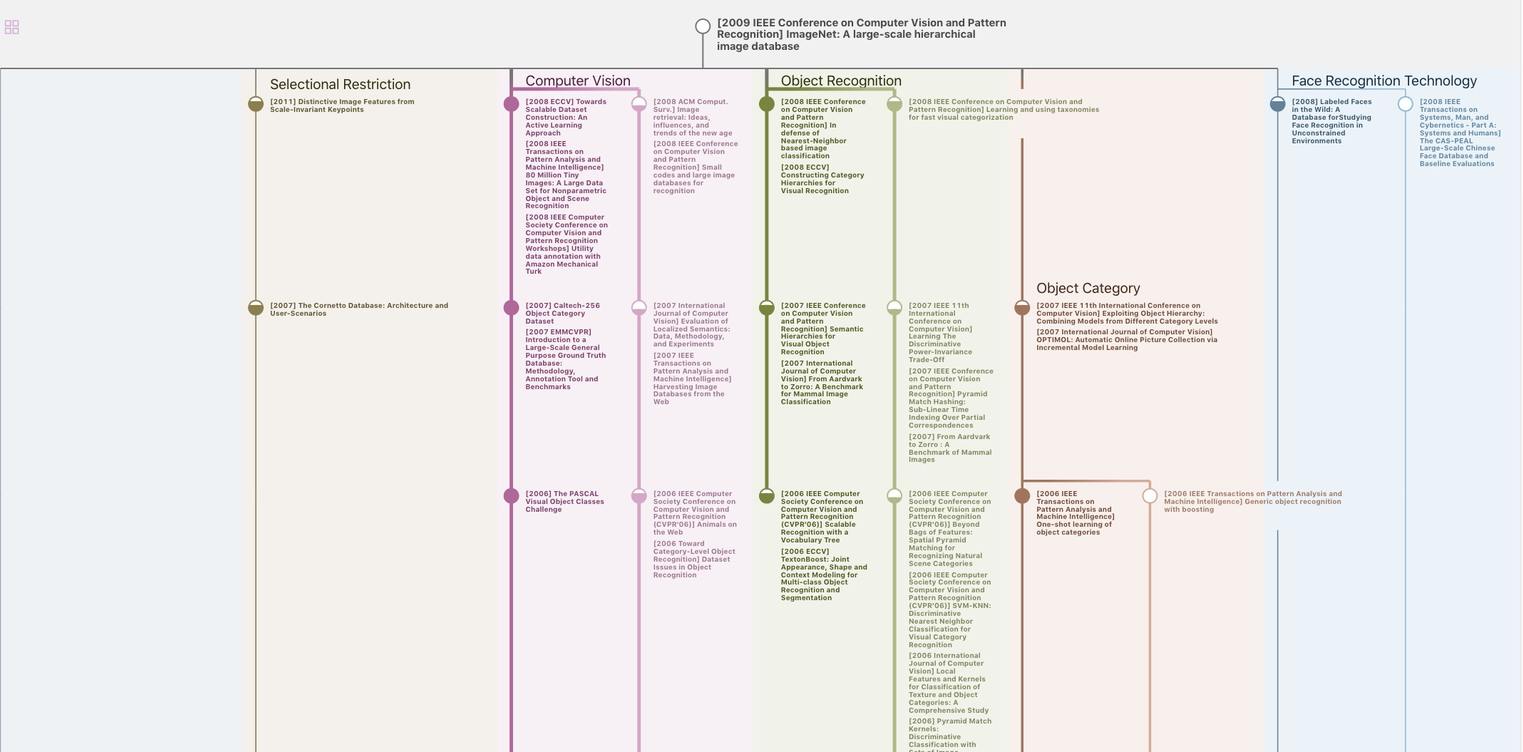
Generate MRT to find the research sequence of this paper
Data Disclaimer
The page data are from open Internet sources, cooperative publishers and automatic analysis results through AI technology. We do not make any commitments and guarantees for the validity, accuracy, correctness, reliability, completeness and timeliness of the page data. If you have any questions, please contact us by email: report@aminer.cn
Chat Paper
GPU is busy, summary generation fails
Rerequest